Contrastive Self-Supervised Learning for Commonsense Reasoning
Tassilo Klein, Moin Nabi
Machine Learning for NLP Short Paper
Session 13A: Jul 8
(12:00-13:00 GMT)
Session 14B: Jul 8
(18:00-19:00 GMT)
Abstract:
We propose a self-supervised method to solve Pronoun Disambiguation and Winograd Schema Challenge problems. Our approach exploits the characteristic structure of training corpora related to so-called ``trigger'' words, which are responsible for flipping the answer in pronoun disambiguation. We achieve such commonsense reasoning by constructing pair-wise contrastive auxiliary predictions. To this end, we leverage a mutual exclusive loss regularized by a contrastive margin. Our architecture is based on the recently introduced transformer networks, BERT, that exhibits strong performance on many NLP benchmarks. Empirical results show that our method alleviates the limitation of current supervised approaches for commonsense reasoning. This study opens up avenues for exploiting inexpensive self-supervision to achieve performance gain in commonsense reasoning tasks.
You can open the
pre-recorded video
in a separate window.
NOTE: The SlidesLive video may display a random order of the authors.
The correct author list is shown at the top of this webpage.
Similar Papers
Pre-training Is (Almost) All You Need: An Application to Commonsense Reasoning
Alexandre Tamborrino, Nicola Pellicanò, Baptiste Pannier, Pascal Voitot, Louise Naudin,
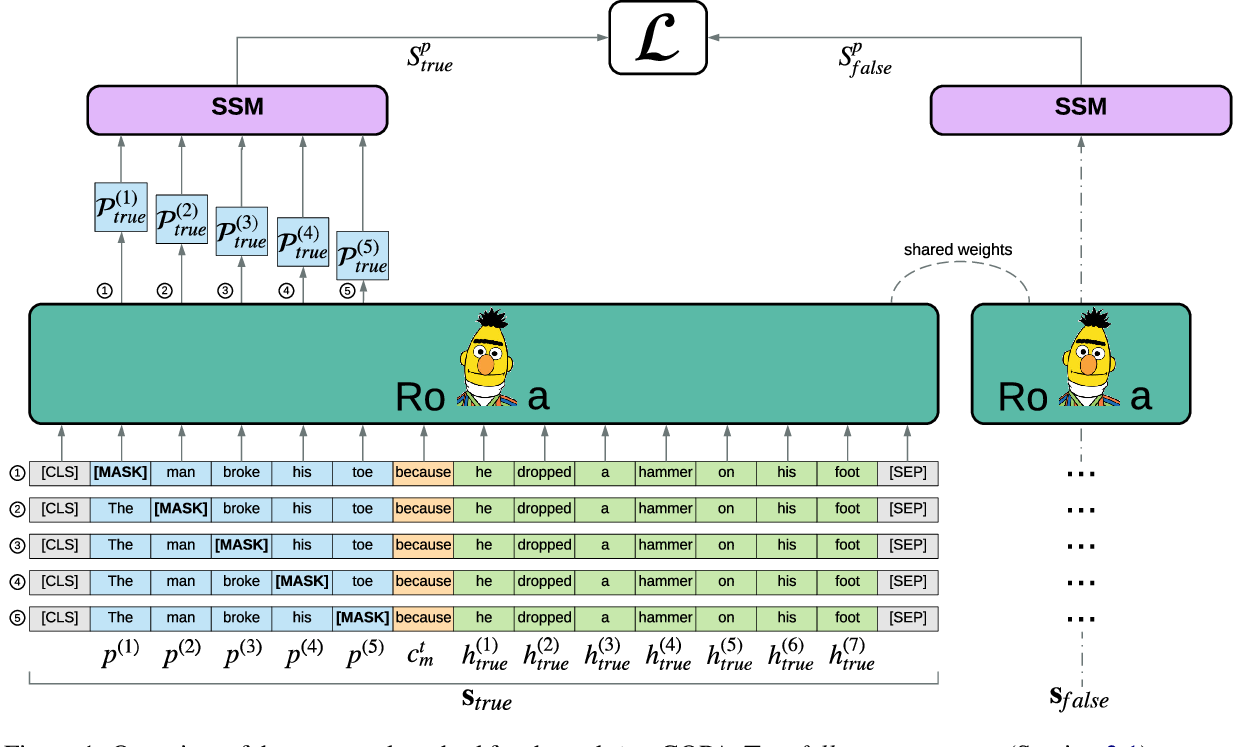
Span Selection Pre-training for Question Answering
Michael Glass, Alfio Gliozzo, Rishav Chakravarti, Anthony Ferritto, Lin Pan, G P Shrivatsa Bhargav, Dinesh Garg, Avi Sil,
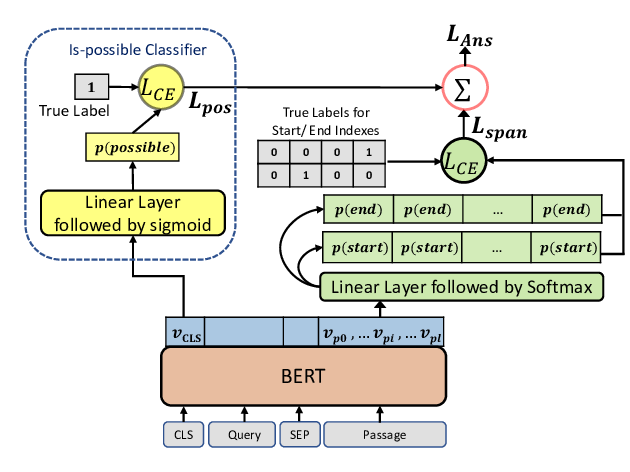
WinoWhy: A Deep Diagnosis of Essential Commonsense Knowledge for Answering Winograd Schema Challenge
Hongming Zhang, Xinran Zhao, Yangqiu Song,
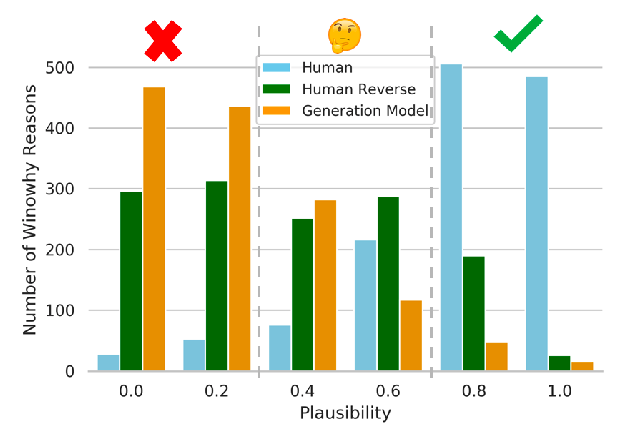