Logic-Guided Data Augmentation and Regularization for Consistent Question Answering
Akari Asai, Hannaneh Hajishirzi
Question Answering Short Paper
Session 9B: Jul 7
(18:00-19:00 GMT)
Session 10A: Jul 7
(20:00-21:00 GMT)
Abstract:
Many natural language questions require qualitative, quantitative or logical comparisons between two entities or events. This paper addresses the problem of improving the accuracy and consistency of responses to comparison questions by integrating logic rules and neural models. Our method leverages logical and linguistic knowledge to augment labeled training data and then uses a consistency-based regularizer to train the model. Improving the global consistency of predictions, our approach achieves large improvements over previous methods in a variety of question answering (QA) tasks, including multiple-choice qualitative reasoning, cause-effect reasoning, and extractive machine reading comprehension. In particular, our method significantly improves the performance of RoBERTa-based models by 1-5% across datasets. We advance state of the art by around 5-8% on WIQA and QuaRel and reduce consistency violations by 58% on HotpotQA. We further demonstrate that our approach can learn effectively from limited data.
You can open the
pre-recorded video
in a separate window.
NOTE: The SlidesLive video may display a random order of the authors.
The correct author list is shown at the top of this webpage.
Similar Papers
Low-Resource Generation of Multi-hop Reasoning Questions
Jianxing Yu, Wei Liu, Shuang Qiu, Qinliang Su, Kai Wang, Xiaojun Quan, Jian Yin,
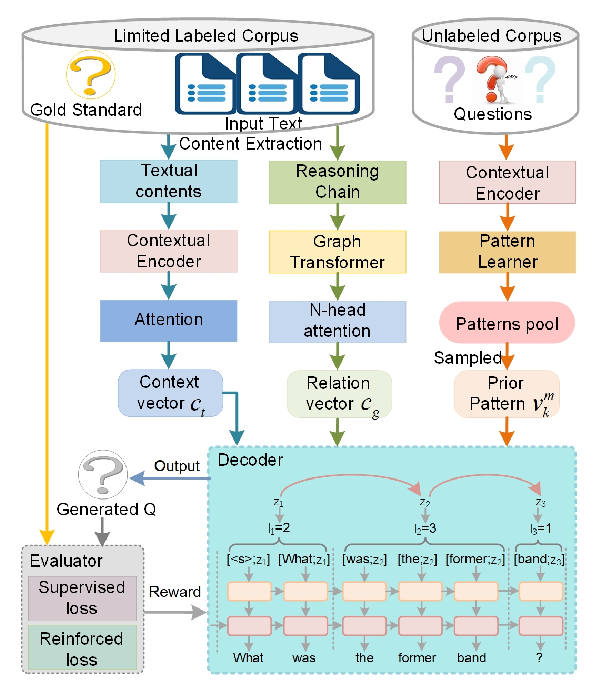
Harvesting and Refining Question-Answer Pairs for Unsupervised QA
Zhongli Li, Wenhui Wang, Li Dong, Furu Wei, Ke Xu,
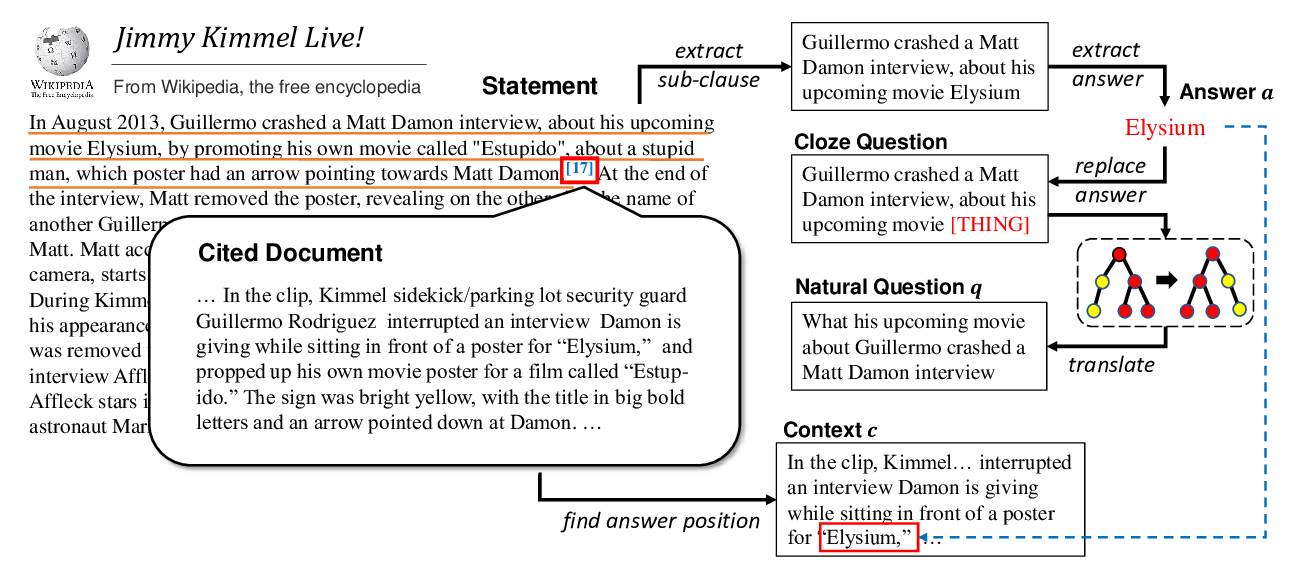
Span Selection Pre-training for Question Answering
Michael Glass, Alfio Gliozzo, Rishav Chakravarti, Anthony Ferritto, Lin Pan, G P Shrivatsa Bhargav, Dinesh Garg, Avi Sil,
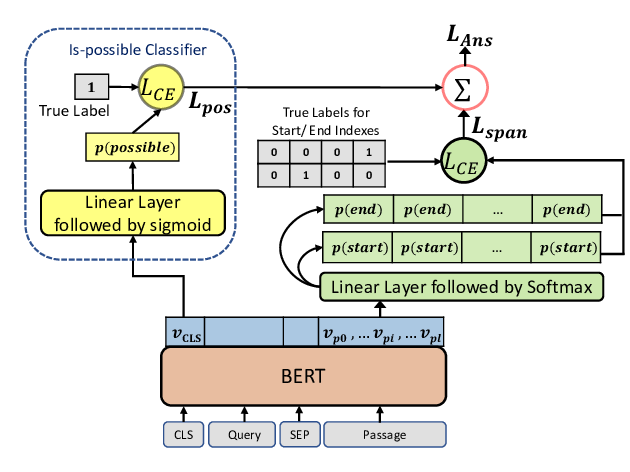
Fluent Response Generation for Conversational Question Answering
Ashutosh Baheti, Alan Ritter, Kevin Small,
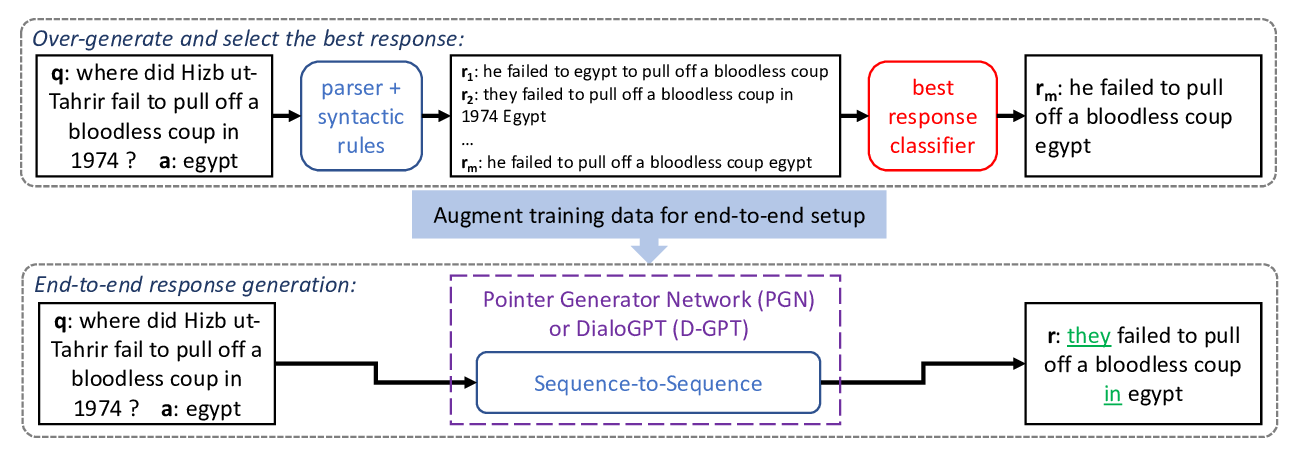