The Cascade Transformer: an Application for Efficient Answer Sentence Selection
Luca Soldaini, Alessandro Moschitti
Question Answering Long Paper
Session 9B: Jul 7
(18:00-19:00 GMT)
Session 10B: Jul 7
(21:00-22:00 GMT)
Abstract:
Large transformer-based language models have been shown to be very effective in many classification tasks. However, their computational complexity prevents their use in applications requiring the classification of a large set of candidates. While previous works have investigated approaches to reduce model size, relatively little attention has been paid to techniques to improve batch throughput during inference. In this paper, we introduce the Cascade Transformer, a simple yet effective technique to adapt transformer-based models into a cascade of rankers. Each ranker is used to prune a subset of candidates in a batch, thus dramatically increasing throughput at inference time. Partial encodings from the transformer model are shared among rerankers, providing further speed-up. When compared to a state-of-the-art transformer model, our approach reduces computation by 37% with almost no impact on accuracy, as measured on two English Question Answering datasets.
You can open the
pre-recorded video
in a separate window.
NOTE: The SlidesLive video may display a random order of the authors.
The correct author list is shown at the top of this webpage.
Similar Papers
The Right Tool for the Job: Matching Model and Instance Complexities
Roy Schwartz, Gabriel Stanovsky, Swabha Swayamdipta, Jesse Dodge, Noah A. Smith,
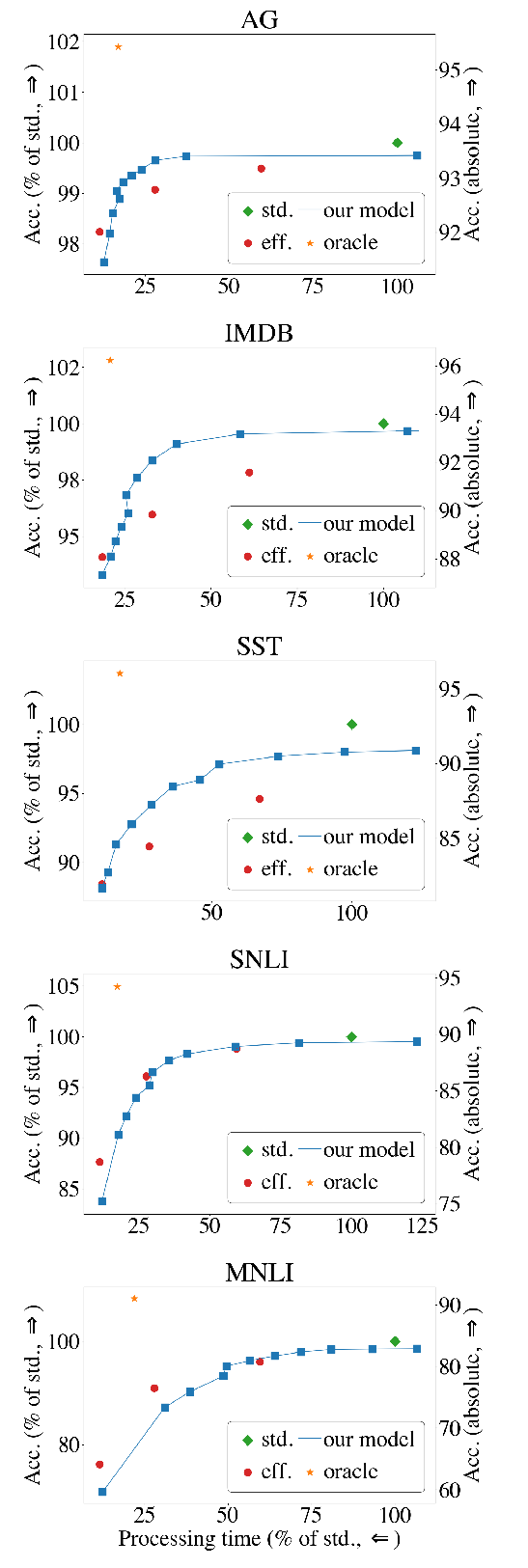
Lipschitz Constrained Parameter Initialization for Deep Transformers
Hongfei Xu, Qiuhui Liu, Josef van Genabith, Deyi Xiong, Jingyi Zhang,
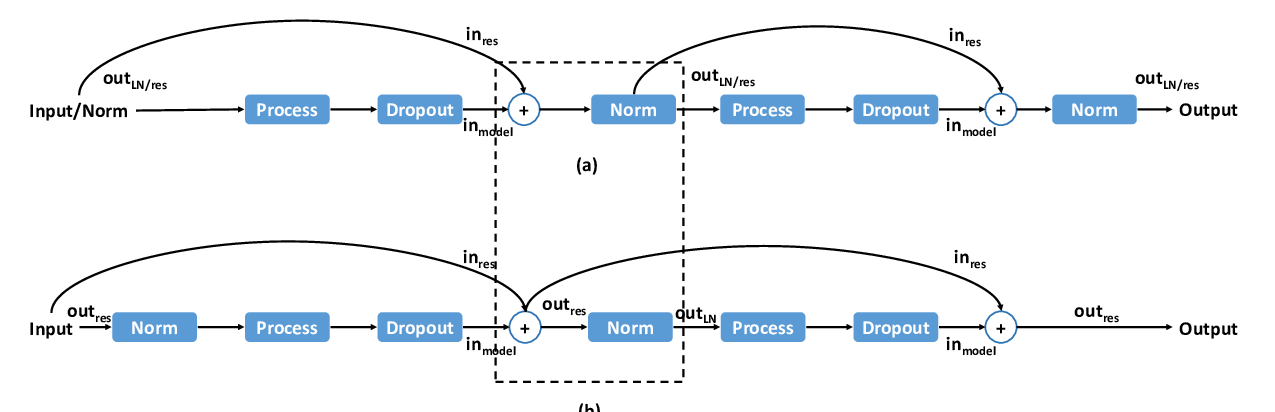
Lexically Constrained Neural Machine Translation with Levenshtein Transformer
Raymond Hendy Susanto, Shamil Chollampatt, Liling Tan,
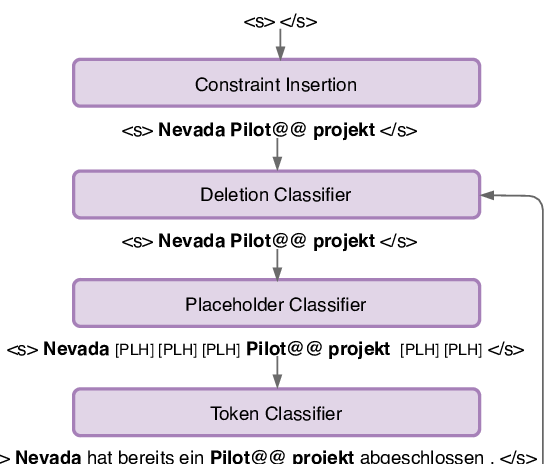
FastBERT: a Self-distilling BERT with Adaptive Inference Time
Weijie Liu, Peng Zhou, Zhiruo Wang, Zhe Zhao, Haotang Deng, QI JU,
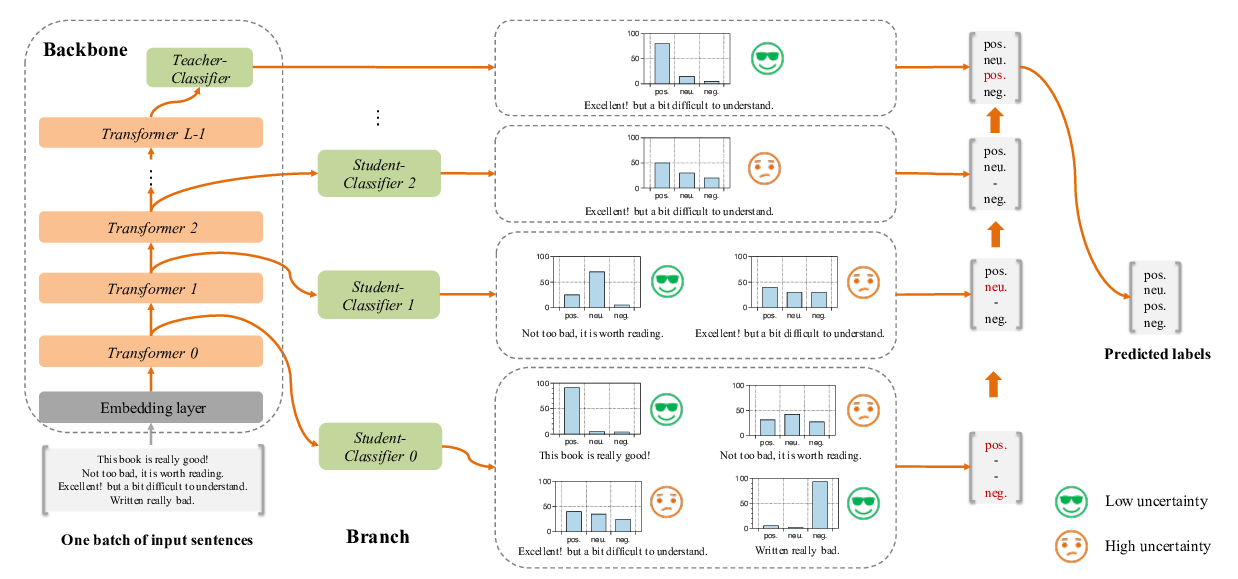