Lexically Constrained Neural Machine Translation with Levenshtein Transformer
Raymond Hendy Susanto, Shamil Chollampatt, Liling Tan
Machine Translation Short Paper
Session 6B: Jul 7
(06:00-07:00 GMT)
Session 7B: Jul 7
(09:00-10:00 GMT)
Abstract:
This paper proposes a simple and effective algorithm for incorporating lexical constraints in neural machine translation. Previous work either required re-training existing models with the lexical constraints or incorporating them during beam search decoding with significantly higher computational overheads. Leveraging the flexibility and speed of a recently proposed Levenshtein Transformer model (Gu et al., 2019), our method injects terminology constraints at inference time without any impact on decoding speed. Our method does not require any modification to the training procedure and can be easily applied at runtime with custom dictionaries. Experiments on English-German WMT datasets show that our approach improves an unconstrained baseline and previous approaches.
You can open the
pre-recorded video
in a separate window.
NOTE: The SlidesLive video may display a random order of the authors.
The correct author list is shown at the top of this webpage.
Similar Papers
The Right Tool for the Job: Matching Model and Instance Complexities
Roy Schwartz, Gabriel Stanovsky, Swabha Swayamdipta, Jesse Dodge, Noah A. Smith,
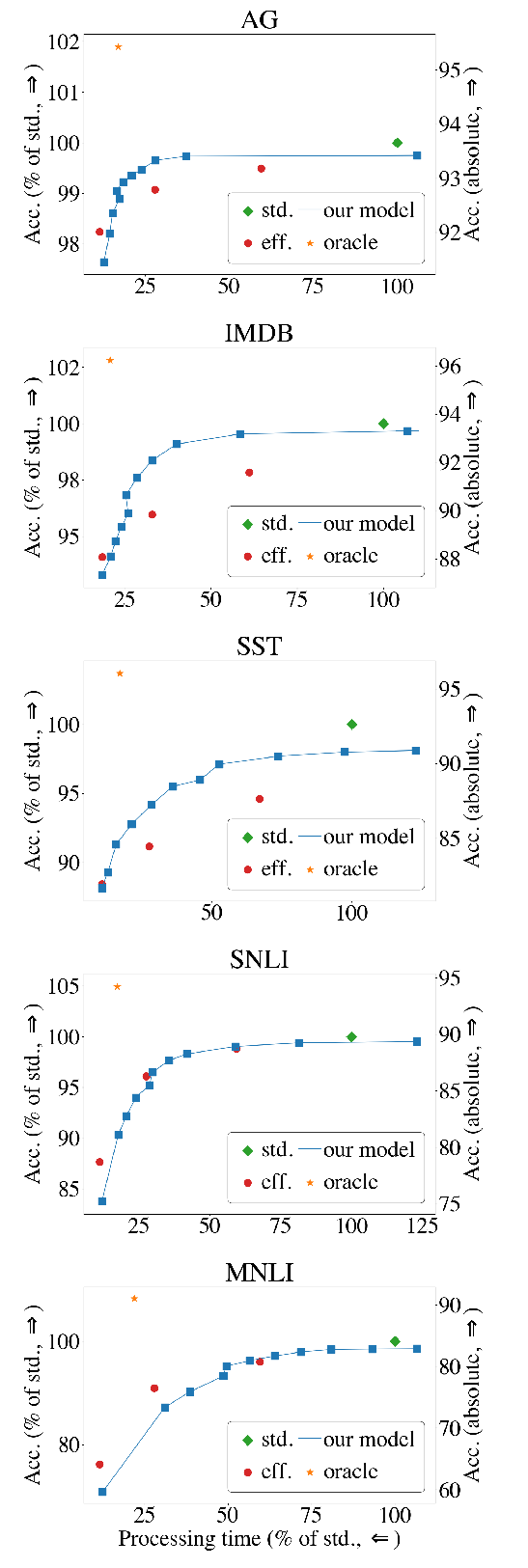
The Cascade Transformer: an Application for Efficient Answer Sentence Selection
Luca Soldaini, Alessandro Moschitti,
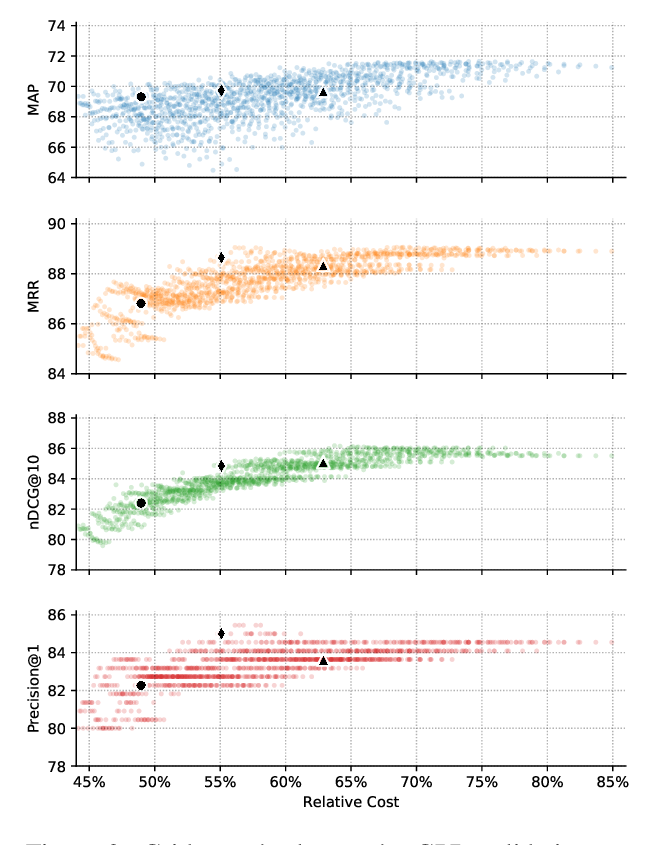
Structured Tuning for Semantic Role Labeling
Tao Li, Parth Anand Jawale, Martha Palmer, Vivek Srikumar,
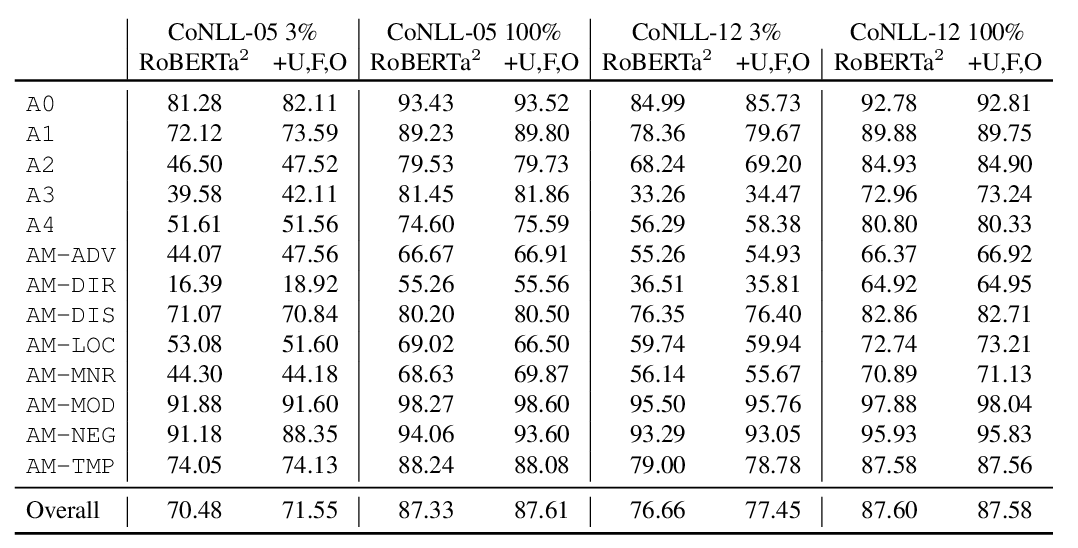
FastBERT: a Self-distilling BERT with Adaptive Inference Time
Weijie Liu, Peng Zhou, Zhiruo Wang, Zhe Zhao, Haotang Deng, QI JU,
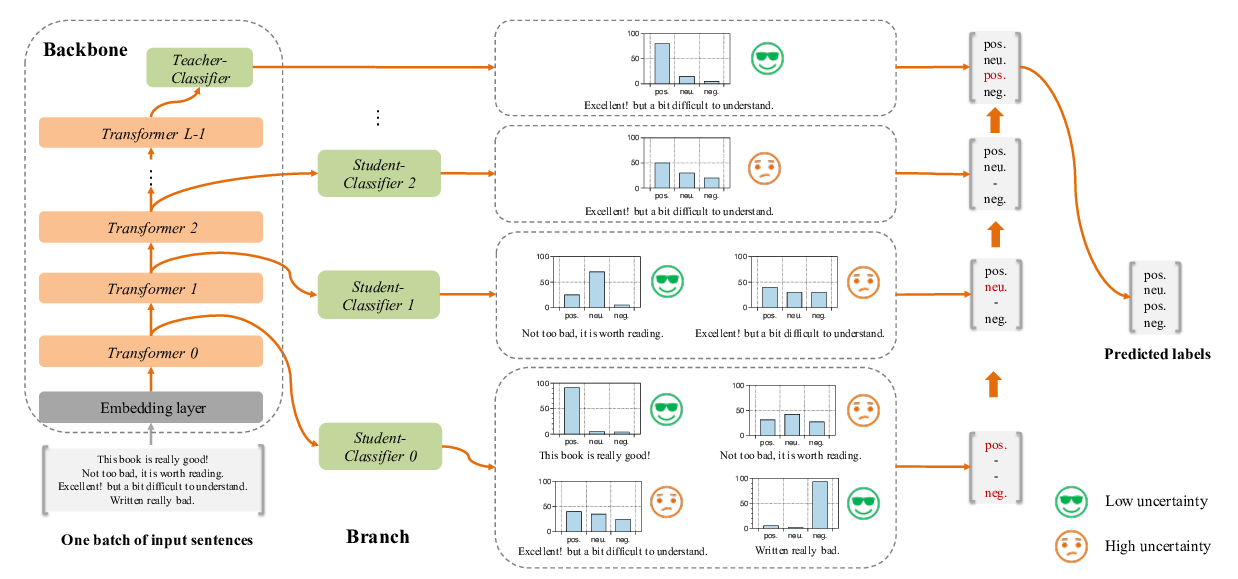