The Right Tool for the Job: Matching Model and Instance Complexities
Roy Schwartz, Gabriel Stanovsky, Swabha Swayamdipta, Jesse Dodge, Noah A. Smith
Machine Learning for NLP Long Paper
Session 11B: Jul 8
(06:00-07:00 GMT)
Session 14B: Jul 8
(18:00-19:00 GMT)
Abstract:
As NLP models become larger, executing a trained model requires significant computational resources incurring monetary and environmental costs. To better respect a given inference budget, we propose a modification to contextual representation fine-tuning which, during inference, allows for an early (and fast) “exit” from neural network calculations for simple instances, and late (and accurate) exit for hard instances. To achieve this, we add classifiers to different layers of BERT and use their calibrated confidence scores to make early exit decisions. We test our proposed modification on five different datasets in two tasks: three text classification datasets and two natural language inference benchmarks. Our method presents a favorable speed/accuracy tradeoff in almost all cases, producing models which are up to five times faster than the state of the art, while preserving their accuracy. Our method also requires almost no additional training resources (in either time or parameters) compared to the baseline BERT model. Finally, our method alleviates the need for costly retraining of multiple models at different levels of efficiency; we allow users to control the inference speed/accuracy tradeoff using a single trained model, by setting a single variable at inference time. We publicly release our code.
You can open the
pre-recorded video
in a separate window.
NOTE: The SlidesLive video may display a random order of the authors.
The correct author list is shown at the top of this webpage.
Similar Papers
FastBERT: a Self-distilling BERT with Adaptive Inference Time
Weijie Liu, Peng Zhou, Zhiruo Wang, Zhe Zhao, Haotang Deng, QI JU,
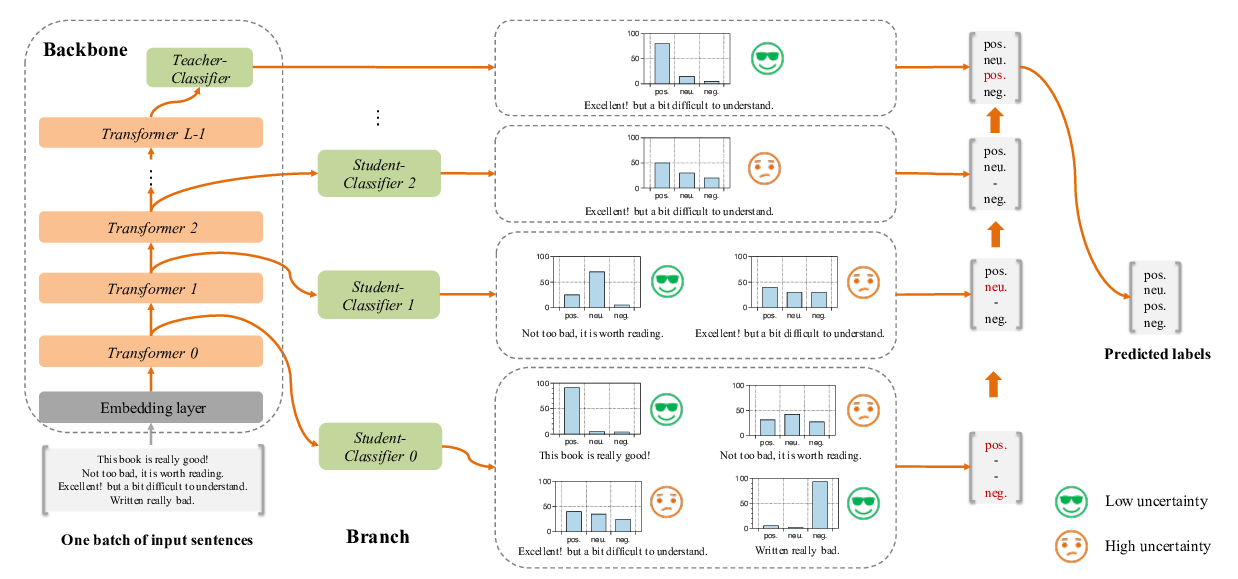
DeeBERT: Dynamic Early Exiting for Accelerating BERT Inference
Ji Xin, Raphael Tang, Jaejun Lee, Yaoliang Yu, Jimmy Lin,
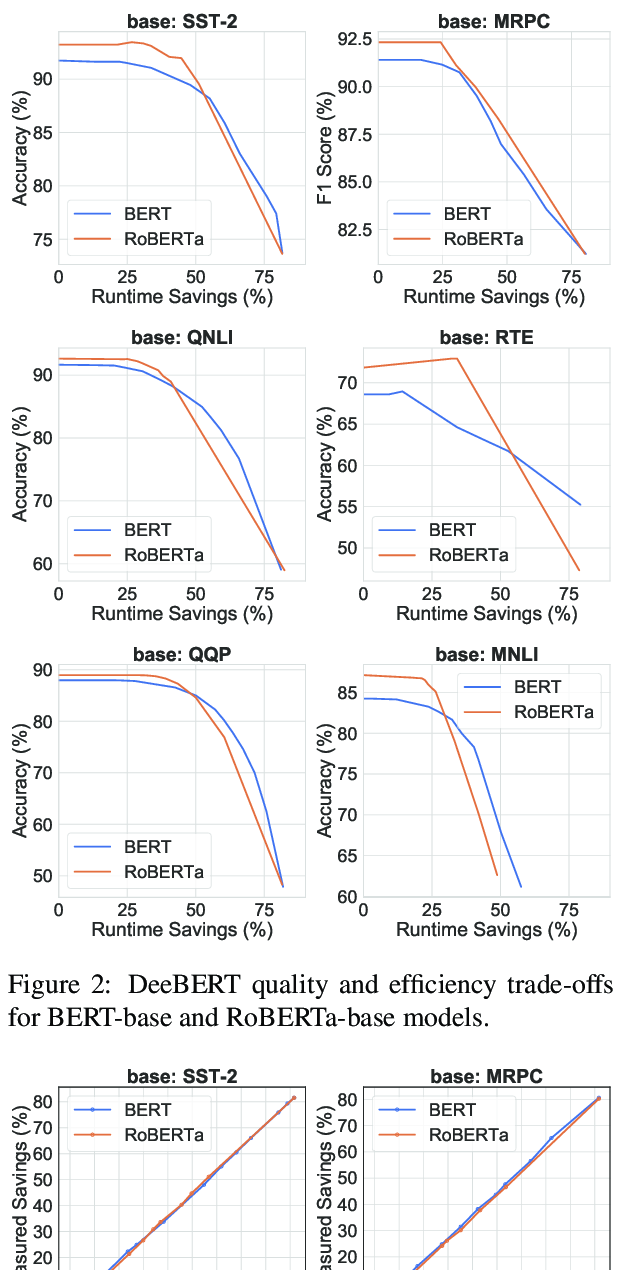
The Cascade Transformer: an Application for Efficient Answer Sentence Selection
Luca Soldaini, Alessandro Moschitti,
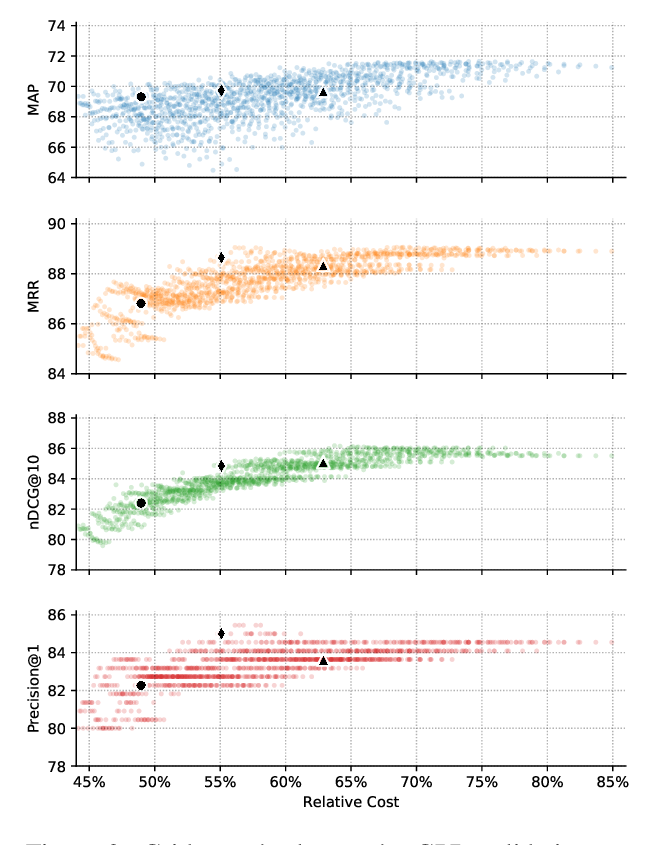
Lexically Constrained Neural Machine Translation with Levenshtein Transformer
Raymond Hendy Susanto, Shamil Chollampatt, Liling Tan,
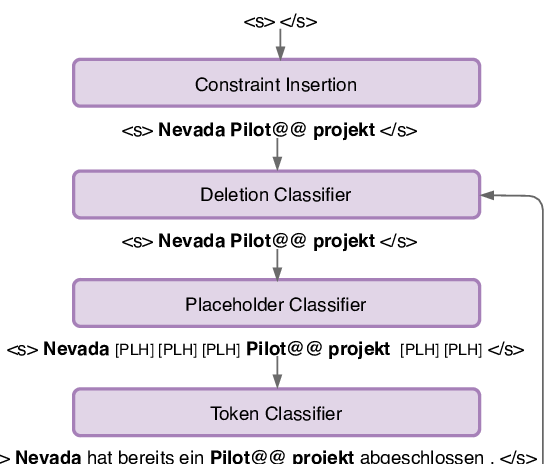