FastBERT: a Self-distilling BERT with Adaptive Inference Time
Weijie Liu, Peng Zhou, Zhiruo Wang, Zhe Zhao, Haotang Deng, QI JU
Semantics: Sentence Level Long Paper
Session 11A: Jul 8
(05:00-06:00 GMT)
Session 13A: Jul 8
(12:00-13:00 GMT)
Abstract:
Pre-trained language models like BERT have proven to be highly performant. However, they are often computationally expensive in many practical scenarios, for such heavy models can hardly be readily implemented with limited resources. To improve their efficiency with an assured model performance, we propose a novel speed-tunable FastBERT with adaptive inference time. The speed at inference can be flexibly adjusted under varying demands, while redundant calculation of samples is avoided. Moreover, this model adopts a unique self-distillation mechanism at fine-tuning, further enabling a greater computational efficacy with minimal loss in performance. Our model achieves promising results in twelve English and Chinese datasets. It is able to speed up by a wide range from 1 to 12 times than BERT if given different speedup thresholds to make a speed-performance tradeoff.
You can open the
pre-recorded video
in a separate window.
NOTE: The SlidesLive video may display a random order of the authors.
The correct author list is shown at the top of this webpage.
Similar Papers
The Right Tool for the Job: Matching Model and Instance Complexities
Roy Schwartz, Gabriel Stanovsky, Swabha Swayamdipta, Jesse Dodge, Noah A. Smith,
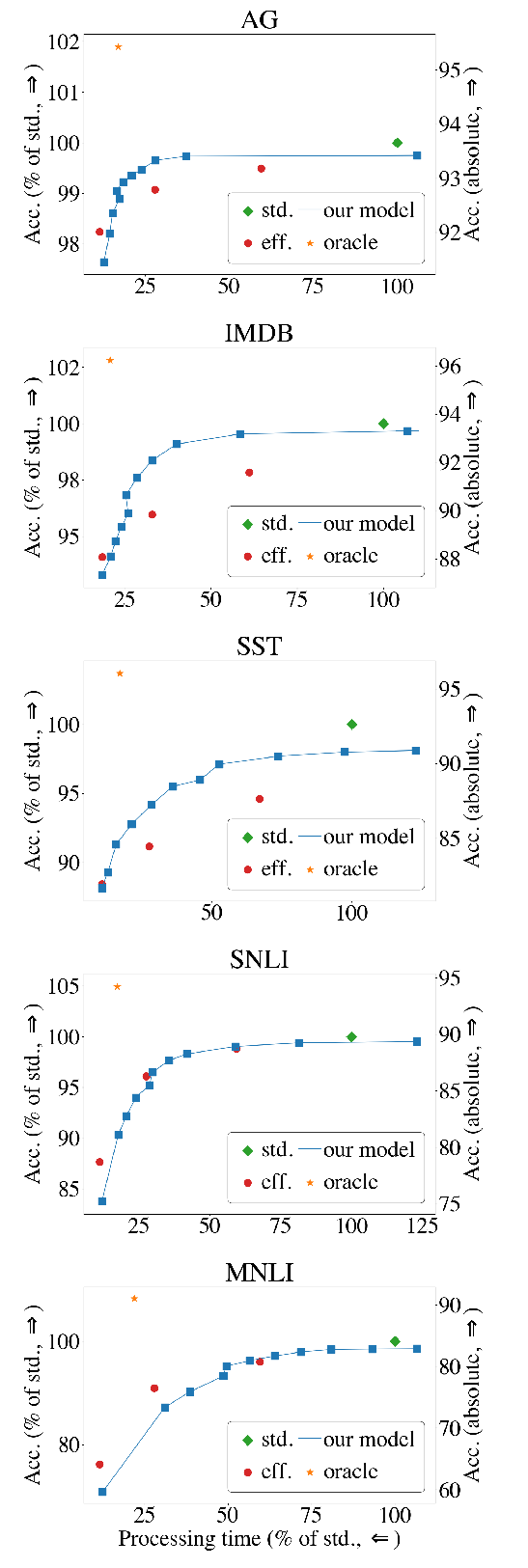
DeeBERT: Dynamic Early Exiting for Accelerating BERT Inference
Ji Xin, Raphael Tang, Jaejun Lee, Yaoliang Yu, Jimmy Lin,
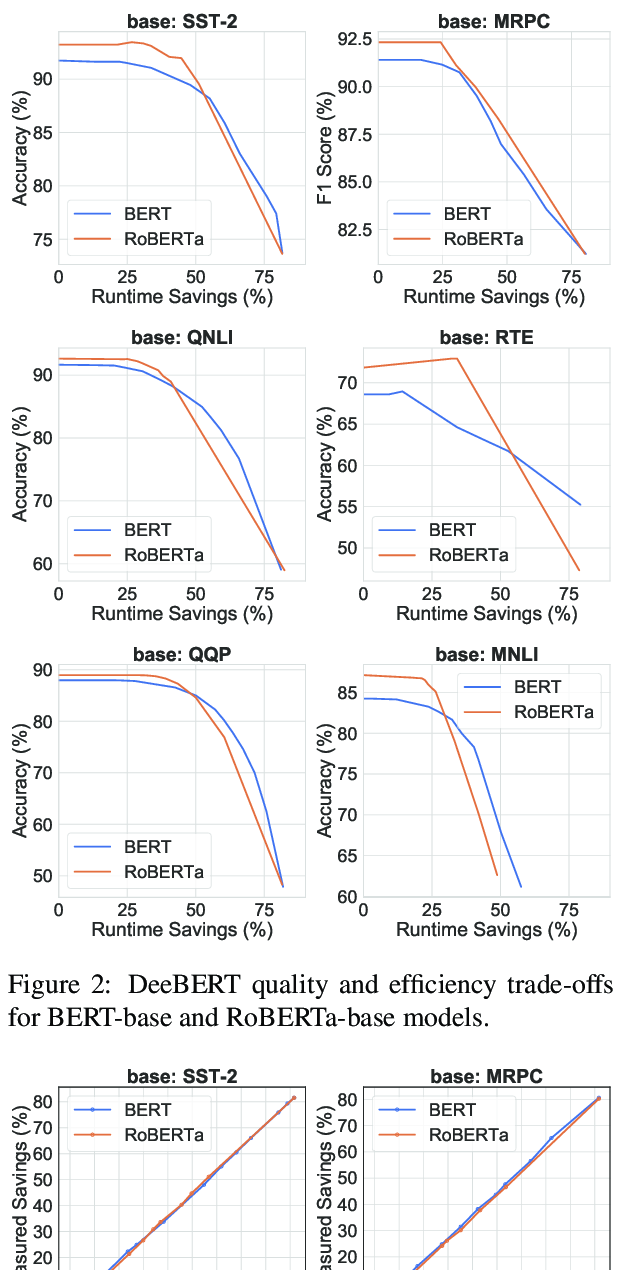
MobileBERT: a Compact Task-Agnostic BERT for Resource-Limited Devices
Zhiqing Sun, Hongkun Yu, Xiaodan Song, Renjie Liu, Yiming Yang, Denny Zhou,
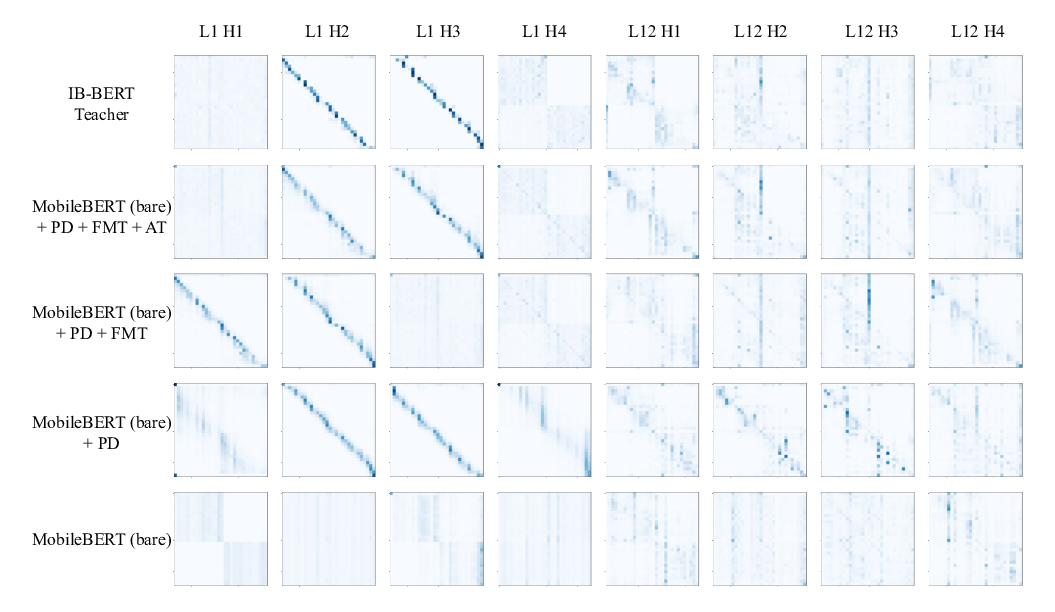
SMART: Robust and Efficient Fine-Tuning for Pre-trained Natural Language Models through Principled Regularized Optimization
Haoming Jiang, Pengcheng He, Weizhu Chen, Xiaodong Liu, Jianfeng Gao, Tuo Zhao,
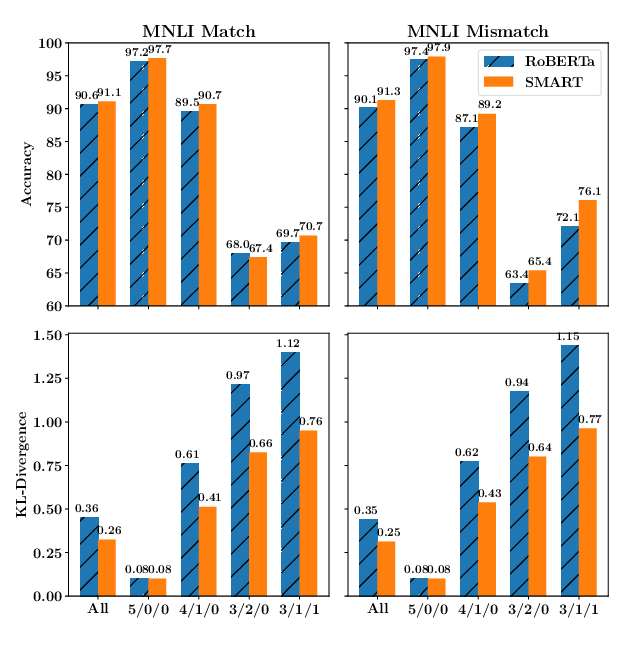