Neural-DINF: A Neural Network based Framework for Measuring Document Influence
Jie Tan, Changlin Yang, Ying Li, Siliang Tang, Chen Huang, Yueting Zhuang
NLP Applications Short Paper
Session 11A: Jul 8
(05:00-06:00 GMT)
Session 12A: Jul 8
(08:00-09:00 GMT)
Abstract:
Measuring the scholarly impact of a document without citations is an important and challenging problem. Existing approaches such as Document Influence Model (DIM) are based on dynamic topic models, which only consider the word frequency change. In this paper, we use both frequency changes and word semantic shifts to measure document influence by developing a neural network framework. Our model has three steps. Firstly, we train the word embeddings for different time periods. Subsequently, we propose an unsupervised method to align vectors for different time periods. Finally, we compute the influence value of documents. Our experimental results show that our model outperforms DIM.
You can open the
pre-recorded video
in a separate window.
NOTE: The SlidesLive video may display a random order of the authors.
The correct author list is shown at the top of this webpage.
Similar Papers
Explaining Black Box Predictions and Unveiling Data Artifacts through Influence Functions
Xiaochuang Han, Byron C. Wallace, Yulia Tsvetkov,
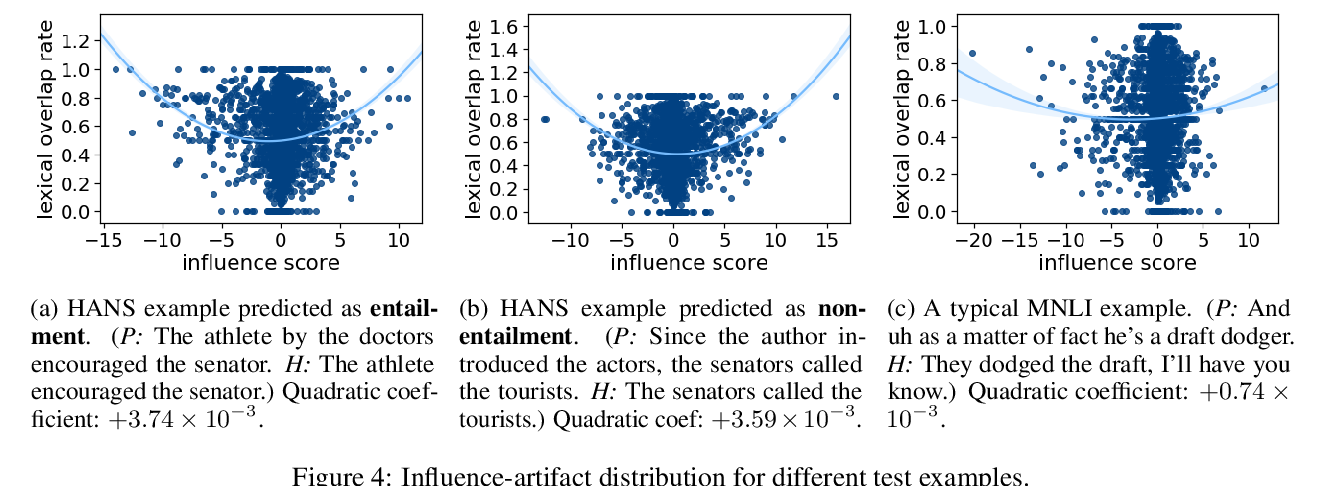
Every Document Owns Its Structure: Inductive Text Classification via Graph Neural Networks
Yufeng Zhang, Xueli Yu, Zeyu Cui, Shu Wu, Zhongzhen Wen, Liang Wang,
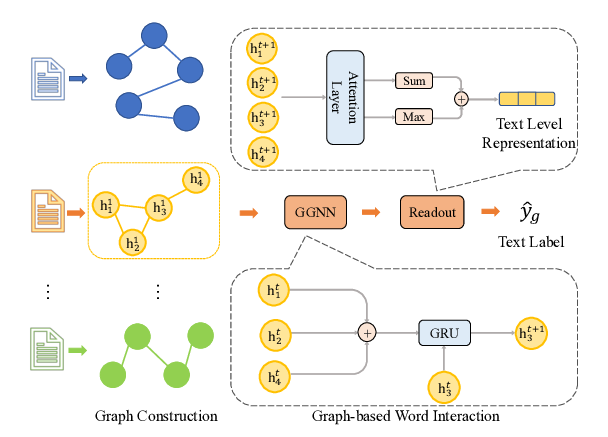
Better Document-level Machine Translation with Bayes' Rule
Lei Yu, Laurent Sartran, Wojciech Stokowiec, Wang Ling, Lingpeng Kong, Phil Blunsom, Chris Dyer,
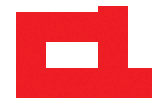
SPECTER: Document-level Representation Learning using Citation-informed Transformers
Arman Cohan, Sergey Feldman, Iz Beltagy, Doug Downey, Daniel Weld,
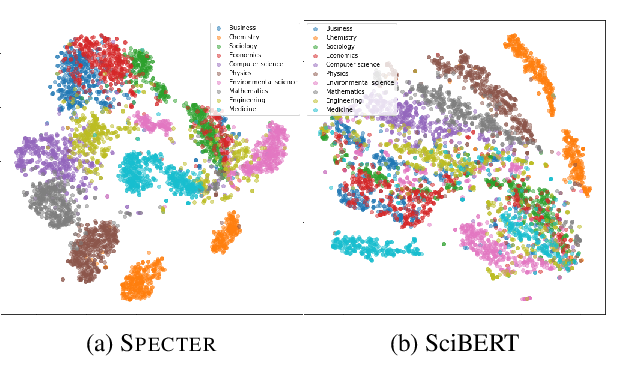