Neural Mixed Counting Models for Dispersed Topic Discovery
Jiemin Wu, Yanghui Rao, Zusheng Zhang, Haoran Xie, Qing Li, Fu Lee Wang, Ziye Chen
Semantics: Textual Inference and Other Areas of Semantics Long Paper
Session 11A: Jul 8
(05:00-06:00 GMT)
Session 14B: Jul 8
(18:00-19:00 GMT)
Abstract:
Mixed counting models that use the negative binomial distribution as the prior can well model over-dispersed and hierarchically dependent random variables; thus they have attracted much attention in mining dispersed document topics. However, the existing parameter inference method like Monte Carlo sampling is quite time-consuming. In this paper, we propose two efficient neural mixed counting models, i.e., the Negative Binomial-Neural Topic Model (NB-NTM) and the Gamma Negative Binomial-Neural Topic Model (GNB-NTM) for dispersed topic discovery. Neural variational inference algorithms are developed to infer model parameters by using the reparameterization of Gamma distribution and the Gaussian approximation of Poisson distribution. Experiments on real-world datasets indicate that our models outperform state-of-the-art baseline models in terms of perplexity and topic coherence. The results also validate that both NB-NTM and GNB-NTM can produce explainable intermediate variables by generating dispersed proportions of document topics.
You can open the
pre-recorded video
in a separate window.
NOTE: The SlidesLive video may display a random order of the authors.
The correct author list is shown at the top of this webpage.
Similar Papers
Neural Topic Modeling with Bidirectional Adversarial Training
Rui Wang, Xuemeng Hu, Deyu Zhou, Yulan He, Yuxuan Xiong, Chenchen Ye, Haiyang Xu,
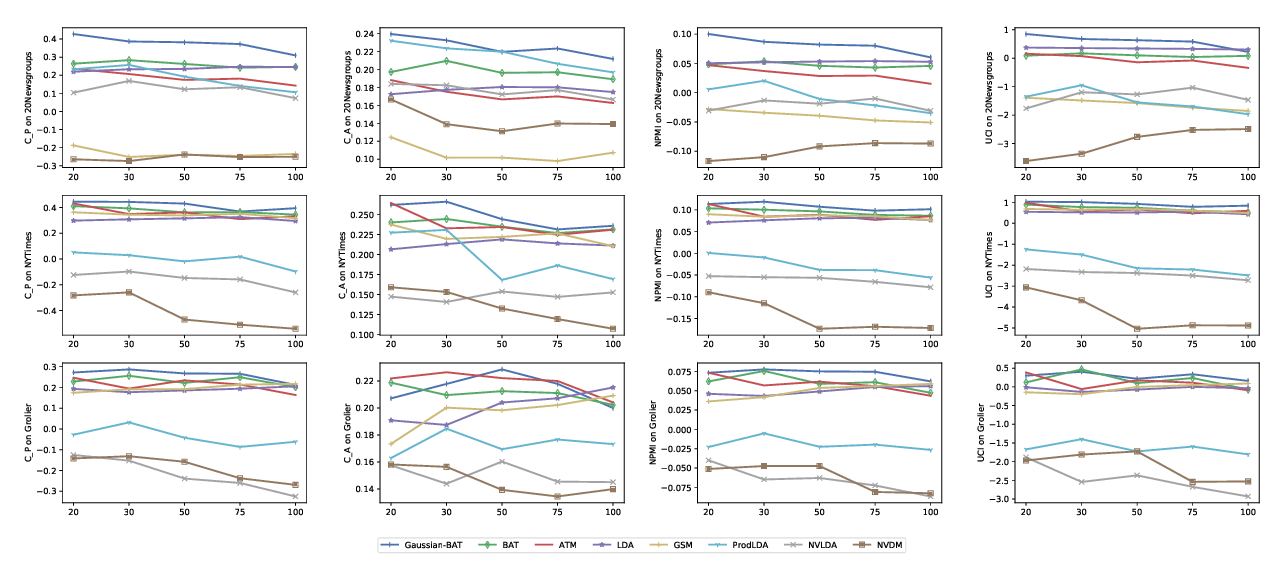
Topic Balancing with Additive Regularization of Topic Models
Eugeniia Veselova, Konstantin Vorontsov,
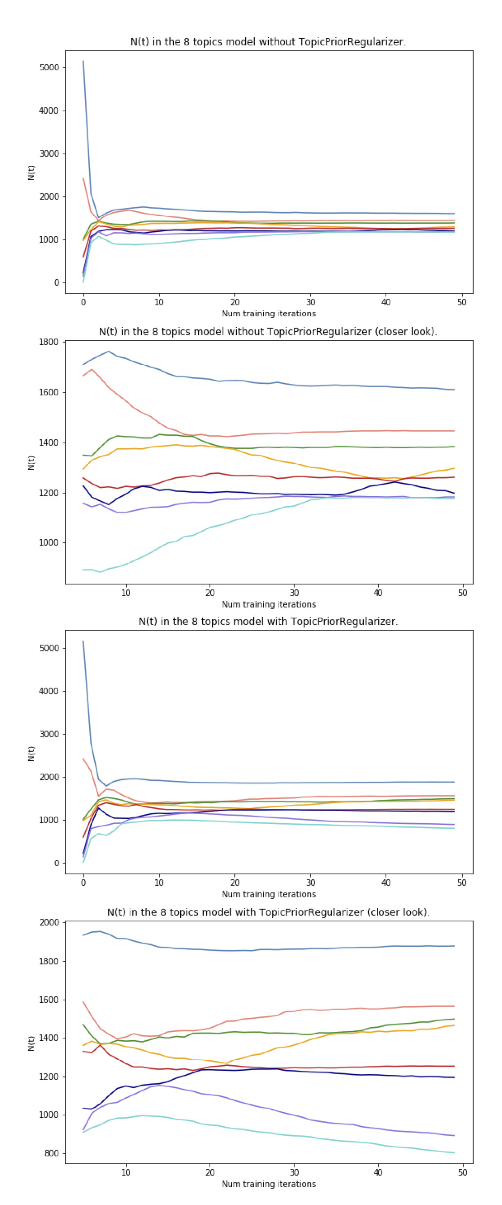
Variational Neural Machine Translation with Normalizing Flows
Hendra Setiawan, Matthias Sperber, Udhyakumar Nallasamy, Matthias Paulik,
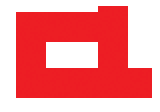
Tree-Structured Neural Topic Model
Masaru Isonuma, Junichiro Mori, Danushka Bollegala, Ichiro Sakata,
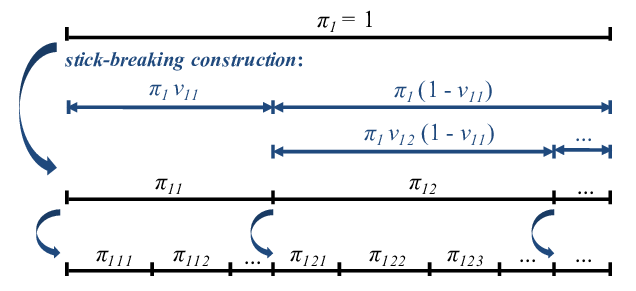