Tree-Structured Neural Topic Model
Masaru Isonuma, Junichiro Mori, Danushka Bollegala, Ichiro Sakata
Information Retrieval and Text Mining Short Paper
Session 1B: Jul 6
(06:00-07:00 GMT)
Session 2A: Jul 6
(08:00-09:00 GMT)
Abstract:
This paper presents a tree-structured neural topic model, which has a topic distribution over a tree with an infinite number of branches. Our model parameterizes an unbounded ancestral and fraternal topic distribution by applying doubly-recurrent neural networks. With the help of autoencoding variational Bayes, our model improves data scalability and achieves competitive performance when inducing latent topics and tree structures, as compared to a prior tree-structured topic model (Blei et al., 2010). This work extends the tree-structured topic model such that it can be incorporated with neural models for downstream tasks.
You can open the
pre-recorded video
in a separate window.
NOTE: The SlidesLive video may display a random order of the authors.
The correct author list is shown at the top of this webpage.
Similar Papers
Neural Topic Modeling with Bidirectional Adversarial Training
Rui Wang, Xuemeng Hu, Deyu Zhou, Yulan He, Yuxuan Xiong, Chenchen Ye, Haiyang Xu,
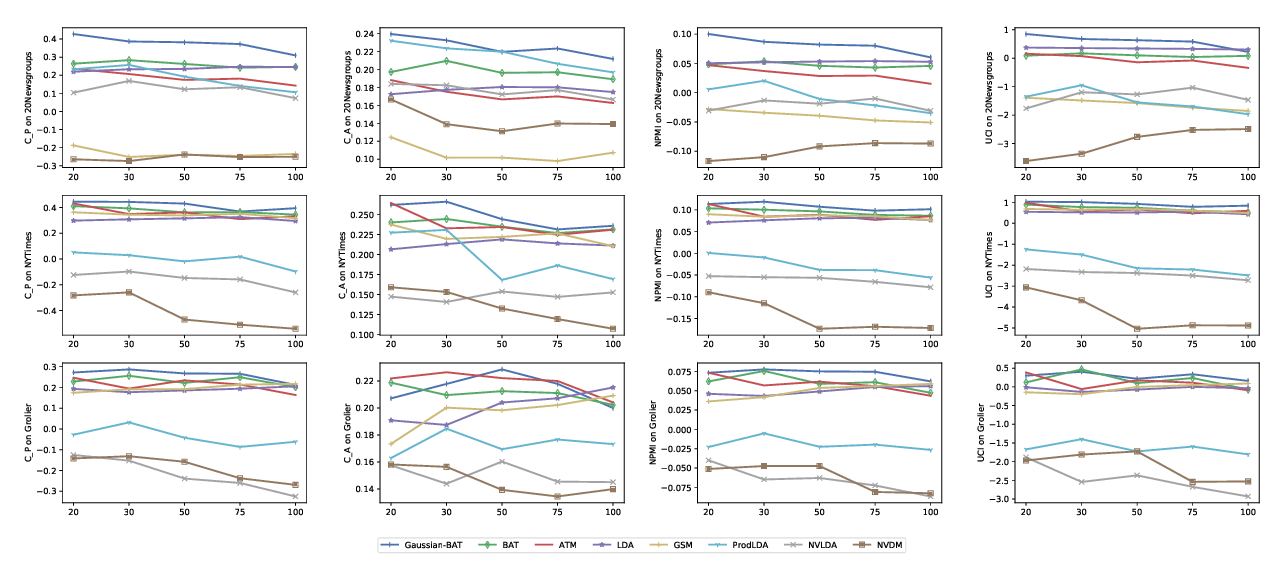
tBERT: Topic Models and BERT Joining Forces for Semantic Similarity Detection
Nicole Peinelt, Dong Nguyen, Maria Liakata,
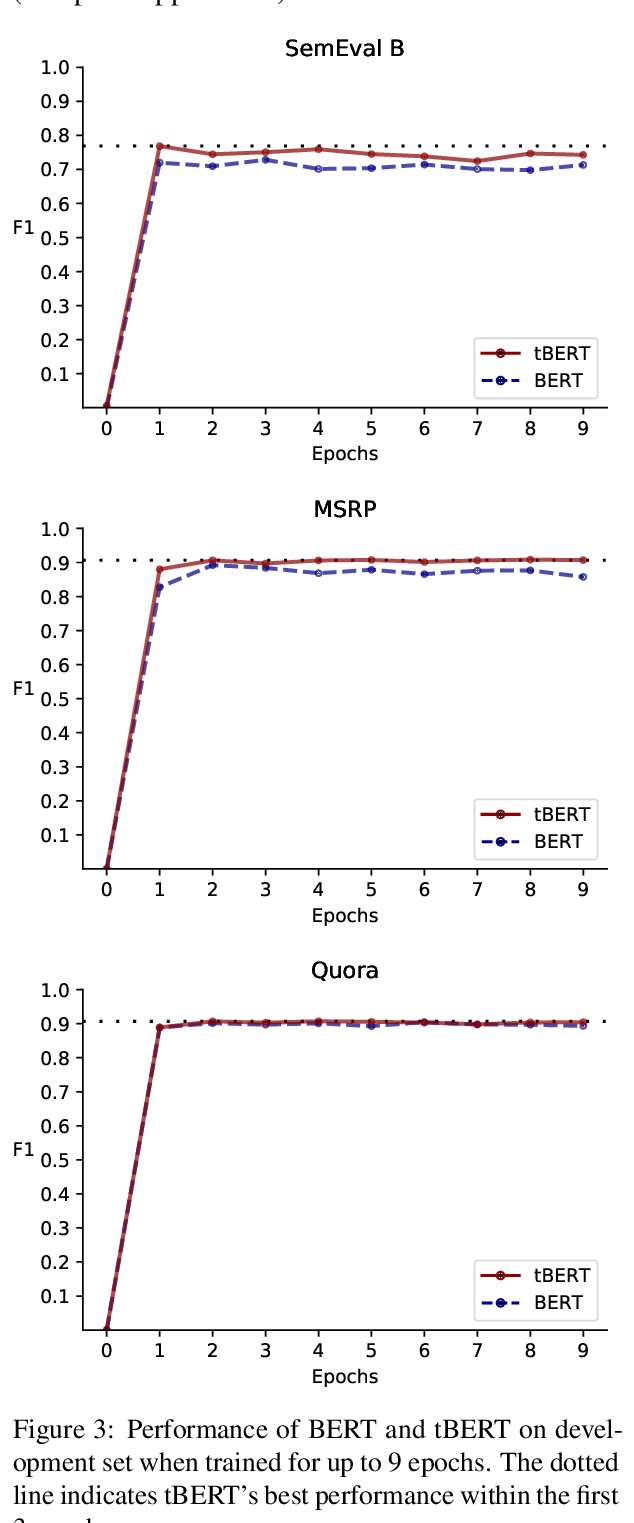
Neural Mixed Counting Models for Dispersed Topic Discovery
Jiemin Wu, Yanghui Rao, Zusheng Zhang, Haoran Xie, Qing Li, Fu Lee Wang, Ziye Chen,
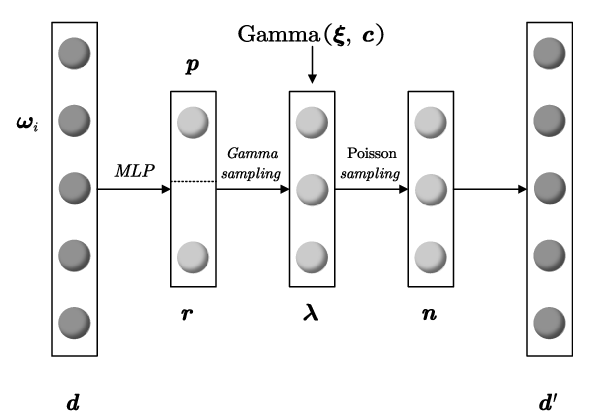
Topic Balancing with Additive Regularization of Topic Models
Eugeniia Veselova, Konstantin Vorontsov,
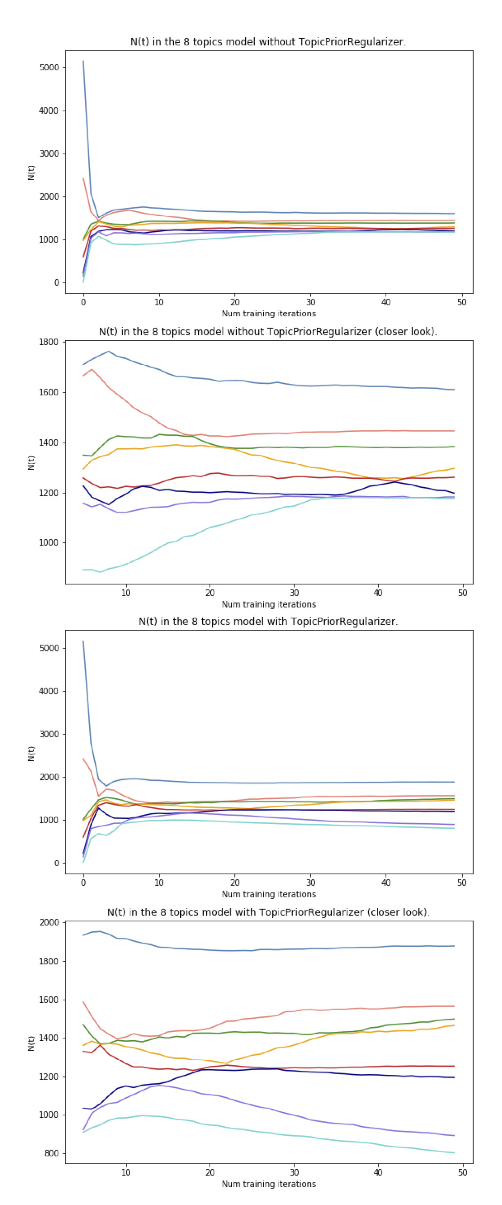