Instance-Based Learning of Span Representations: A Case Study through Named Entity Recognition
Hiroki Ouchi, Jun Suzuki, Sosuke Kobayashi, Sho Yokoi, Tatsuki Kuribayashi, Ryuto Konno, Kentaro Inui
Information Extraction Short Paper
Session 11B: Jul 8
(06:00-07:00 GMT)
Session 12B: Jul 8
(09:00-10:00 GMT)
Abstract:
Interpretable rationales for model predictions play a critical role in practical applications. In this study, we develop models possessing interpretable inference process for structured prediction. Specifically, we present a method of instance-based learning that learns similarities between spans. At inference time, each span is assigned a class label based on its similar spans in the training set, where it is easy to understand how much each training instance contributes to the predictions. Through empirical analysis on named entity recognition, we demonstrate that our method enables to build models that have high interpretability without sacrificing performance.
You can open the
pre-recorded video
in a separate window.
NOTE: The SlidesLive video may display a random order of the authors.
The correct author list is shown at the top of this webpage.
Similar Papers
On the Inference Calibration of Neural Machine Translation
Shuo Wang, Zhaopeng Tu, Shuming Shi, Yang Liu,
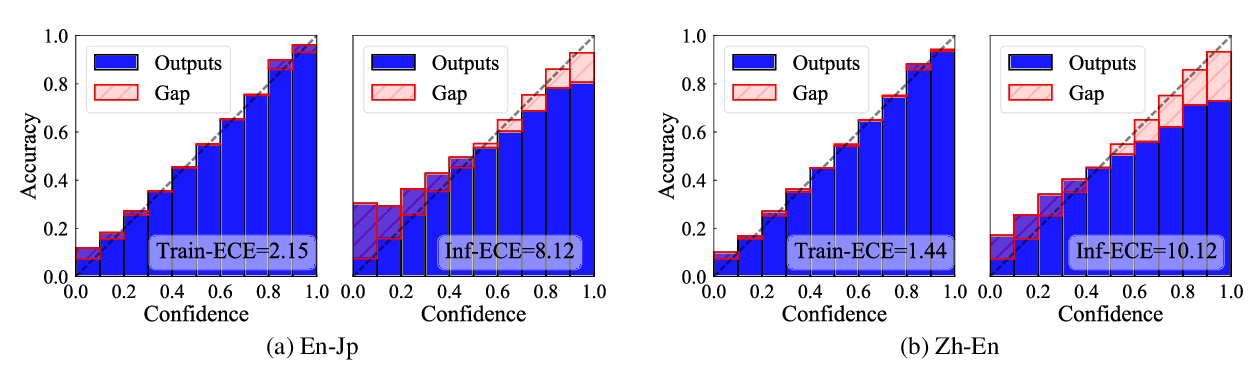
Do Neural Models Learn Systematicity of Monotonicity Inference in Natural Language?
Hitomi Yanaka, Koji Mineshima, Daisuke Bekki, Kentaro Inui,
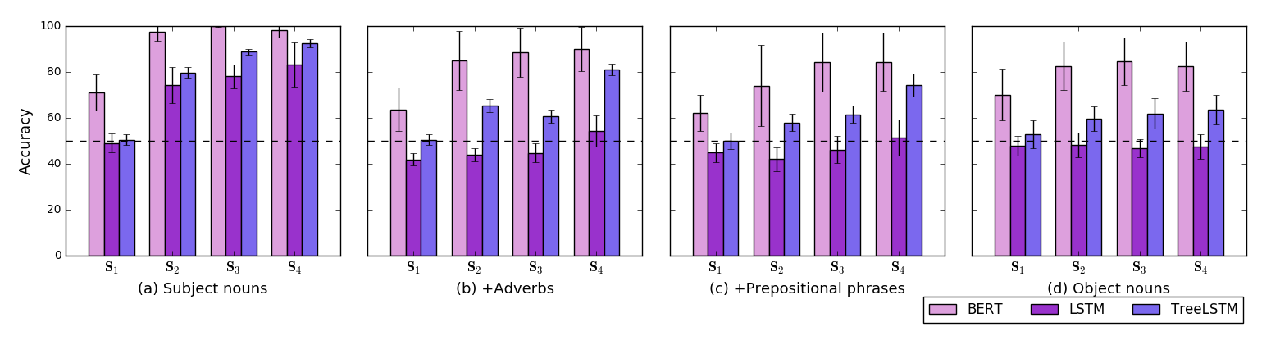
SpanBERT: Improving Pre-training by Representing and Predicting Spans
Mandar Joshi, Danqi Chen, Yinhan Liu, Daniel S. Weld, Luke Zettlemoyer, Omer Levy,
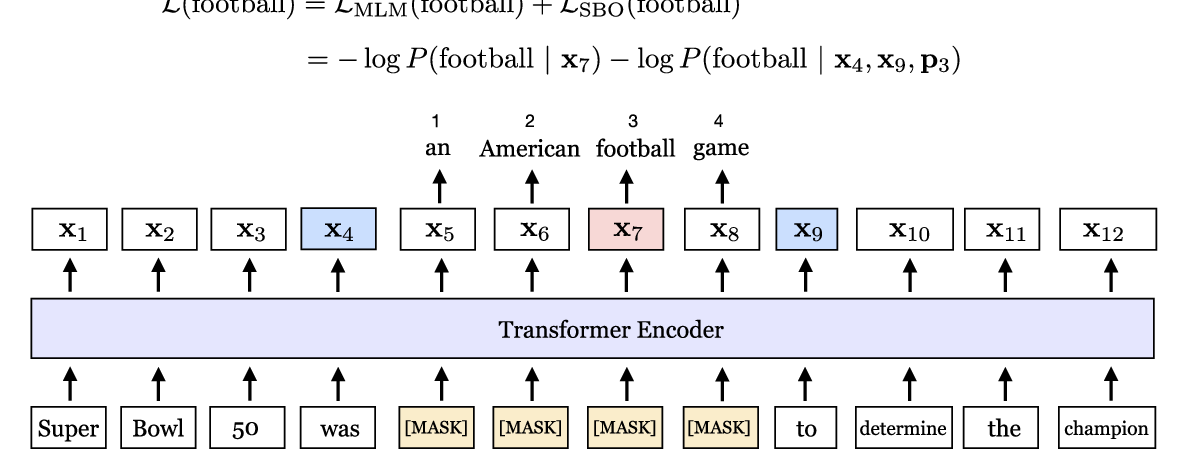