Abstract:
Semantic dependency parsing, which aims to find rich bi-lexical relationships, allows words to have multiple dependency heads, resulting in graph-structured representations. We propose an approach to semi-supervised learning of semantic dependency parsers based on the CRF autoencoder framework. Our encoder is a discriminative neural semantic dependency parser that predicts the latent parse graph of the input sentence. Our decoder is a generative neural model that reconstructs the input sentence conditioned on the latent parse graph. Our model is arc-factored and therefore parsing and learning are both tractable. Experiments show our model achieves significant and consistent improvement over the supervised baseline.
You can open the
pre-recorded video
in a separate window.
NOTE: The SlidesLive video may display a random order of the authors.
The correct author list is shown at the top of this webpage.
Similar Papers
Dependency Graph Enhanced Dual-transformer Structure for Aspect-based Sentiment Classification
Hao Tang, Donghong Ji, Chenliang Li, Qiji Zhou,
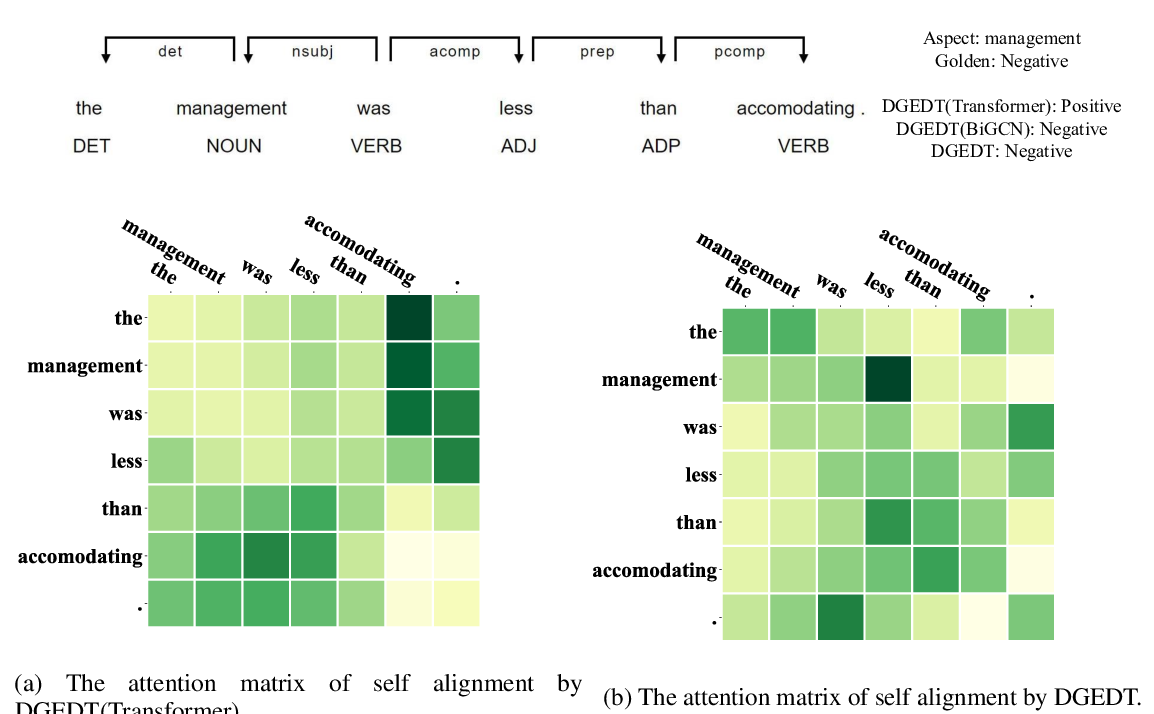
A Graph-based Model for Joint Chinese Word Segmentation and Dependency Parsing
Hang Yan, Xipeng Qiu, Xuanjing Huang,
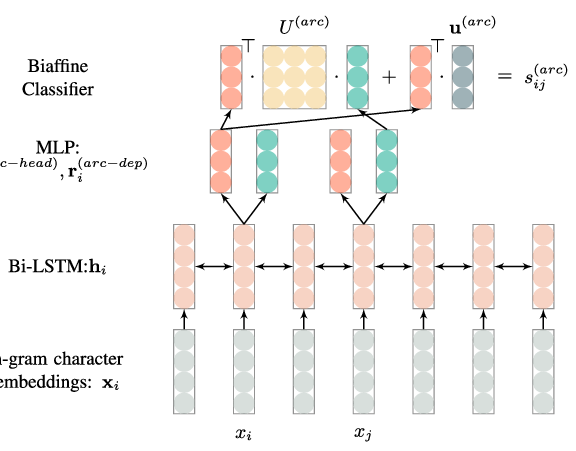