Learning Robust Models for e-Commerce Product Search
Thanh Nguyen, Nikhil Rao, Karthik Subbian
Information Retrieval and Text Mining Short Paper
Session 12A: Jul 8
(08:00-09:00 GMT)
Session 15A: Jul 8
(20:00-21:00 GMT)
Abstract:
Showing items that do not match search query intent degrades customer experience in e-commerce. These mismatches result from counterfactual biases of the ranking algorithms toward noisy behavioral signals such as clicks and purchases in the search logs. Mitigating the problem requires a large labeled dataset, which is expensive and time-consuming to obtain. In this paper, we develop a deep, end-to-end model that learns to effectively classify mismatches and to generate hard mismatched examples to improve the classifier. We train the model end-to-end by introducing a latent variable into the cross-entropy loss that alternates between using the real and generated samples. This not only makes the classifier more robust but also boosts the overall ranking performance. Our model achieves a relative gain compared to baselines by over 26% in F-score, and over 17% in Area Under PR curve. On live search traffic, our model gains significant improvement in multiple countries.
You can open the
pre-recorded video
in a separate window.
NOTE: The SlidesLive video may display a random order of the authors.
The correct author list is shown at the top of this webpage.
Similar Papers
Let Me Choose: From Verbal Context to Font Selection
Amirreza Shirani, Franck Dernoncourt, Jose Echevarria, Paul Asente, Nedim Lipka, Thamar Solorio,

Dynamic Fusion Network for Multi-Domain End-to-end Task-Oriented Dialog
Libo Qin, Xiao Xu, Wanxiang Che, Yue Zhang, Ting Liu,
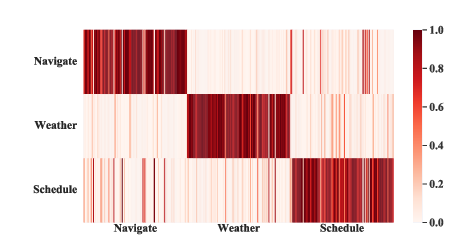