Phone Features Improve Speech Translation
Elizabeth Salesky, Alan W Black
Speech and Multimodality Long Paper
Session 4A: Jul 6
(17:00-18:00 GMT)
Session 5A: Jul 6
(20:00-21:00 GMT)
Abstract:
End-to-end models for speech translation (ST) more tightly couple speech recognition (ASR) and machine translation (MT) than a traditional cascade of separate ASR and MT models, with simpler model architectures and the potential for reduced error propagation. Their performance is often assumed to be superior, though in many conditions this is not yet the case. We compare cascaded and end-to-end models across high, medium, and low-resource conditions, and show that cascades remain stronger baselines. Further, we introduce two methods to incorporate phone features into ST models. We show that these features improve both architectures, closing the gap between end-to-end models and cascades, and outperforming previous academic work -- by up to 9 BLEU on our low-resource setting.
You can open the
pre-recorded video
in a separate window.
NOTE: The SlidesLive video may display a random order of the authors.
The correct author list is shown at the top of this webpage.
Similar Papers
Speech Translation and the End-to-End Promise: Taking Stock of Where We Are
Matthias Sperber, Matthias Paulik,
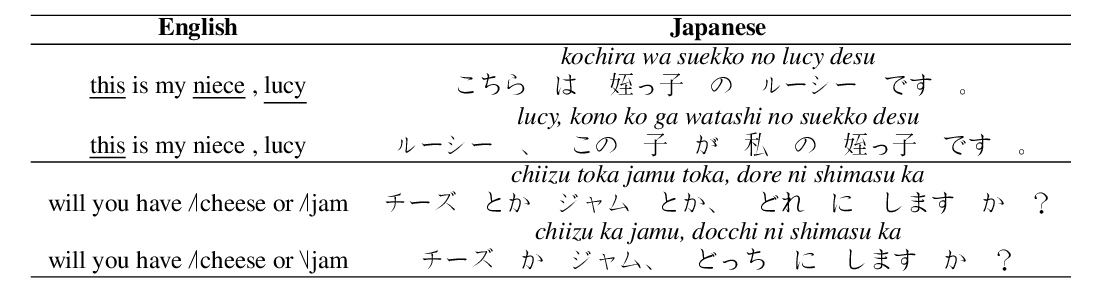
Hypernymy Detection for Low-Resource Languages via Meta Learning
Changlong Yu, Jialong Han, Haisong Zhang, Wilfred Ng,
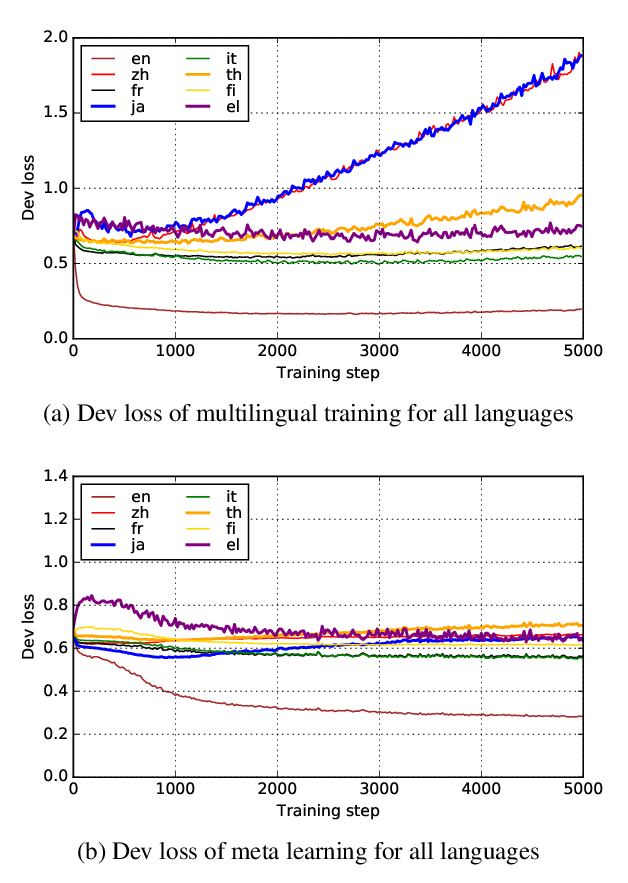
How Accents Confound: Probing for Accent Information in End-to-End Speech Recognition Systems
Archiki Prasad, Preethi Jyothi,
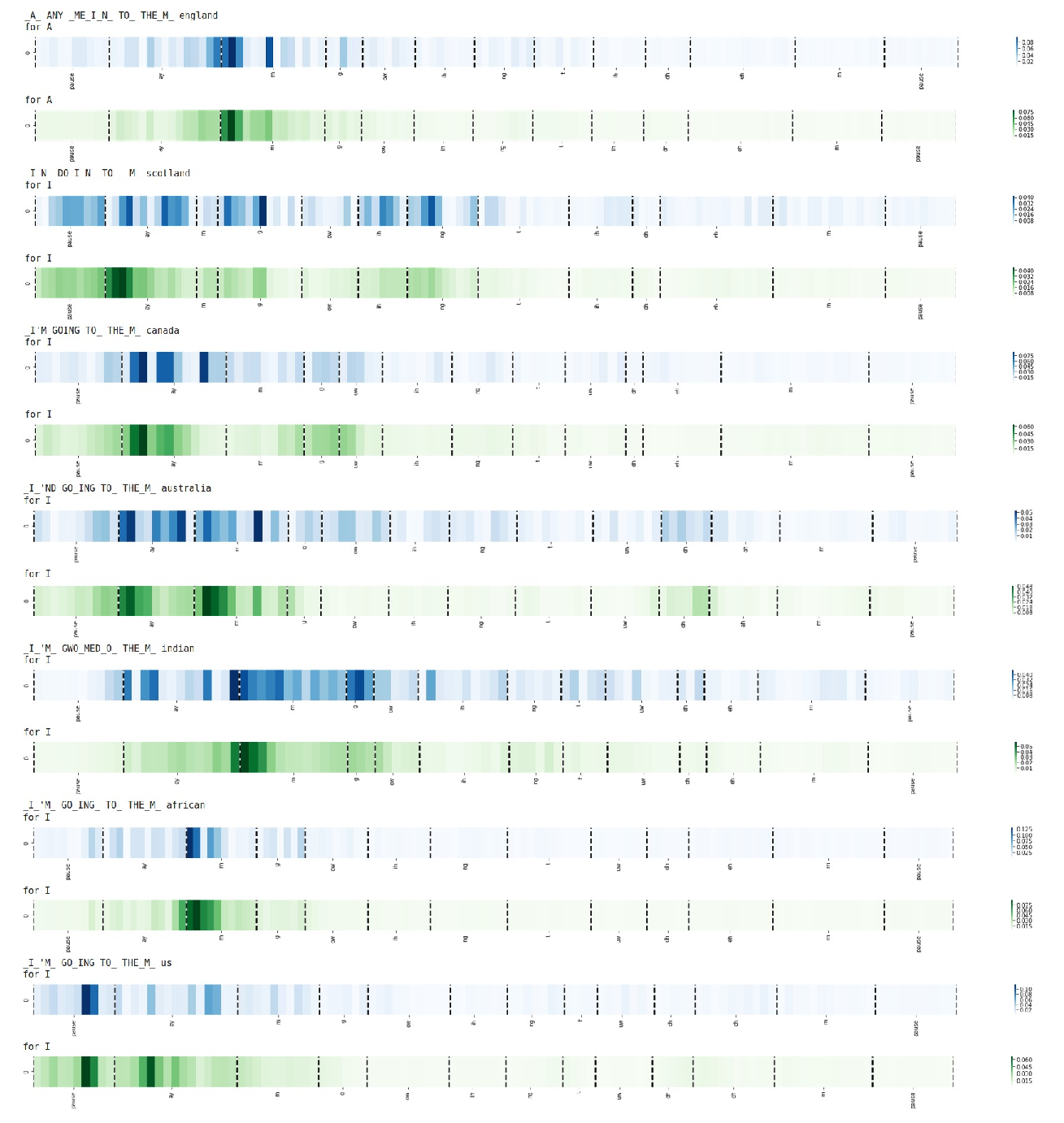