Semantic Scaffolds for Pseudocode-to-Code Generation
Ruiqi Zhong, Mitchell Stern, Dan Klein
NLP Applications Long Paper
Session 4A: Jul 6
(17:00-18:00 GMT)
Session 5B: Jul 6
(21:00-22:00 GMT)
Abstract:
We propose a method for program generation based on semantic scaffolds, lightweight structures representing the high-level semantic and syntactic composition of a program. By first searching over plausible scaffolds then using these as constraints for a beam search over programs, we achieve better coverage of the search space when compared with existing techniques. We apply our hierarchical search method to the SPoC dataset for pseudocode-to-code generation, in which we are given line-level natural language pseudocode annotations and aim to produce a program satisfying execution-based test cases. By using semantic scaffolds during inference, we achieve a 10% absolute improvement in top-100 accuracy over the previous state-of-the-art. Additionally, we require only 11 candidates to reach the top-3000 performance of the previous best approach when tested against unseen problems, demonstrating a substantial improvement in efficiency.
You can open the
pre-recorded video
in a separate window.
NOTE: The SlidesLive video may display a random order of the authors.
The correct author list is shown at the top of this webpage.
Similar Papers
Iterative Edit-Based Unsupervised Sentence Simplification
Dhruv Kumar, Lili Mou, Lukasz Golab, Olga Vechtomova,
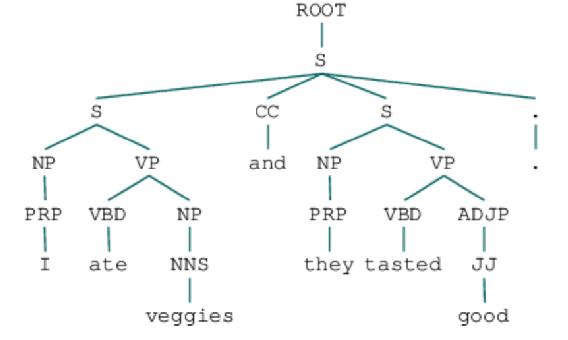
Efficient Strategies for Hierarchical Text Classification: External Knowledge and Auxiliary Tasks
Kervy Rivas Rojas, Gina Bustamante, Arturo Oncevay, Marco Antonio Sobrevilla Cabezudo,
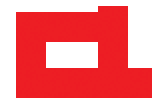
RAT-SQL: Relation-Aware Schema Encoding and Linking for Text-to-SQL Parsers
Bailin Wang, Richard Shin, Xiaodong Liu, Oleksandr Polozov, Matthew Richardson,
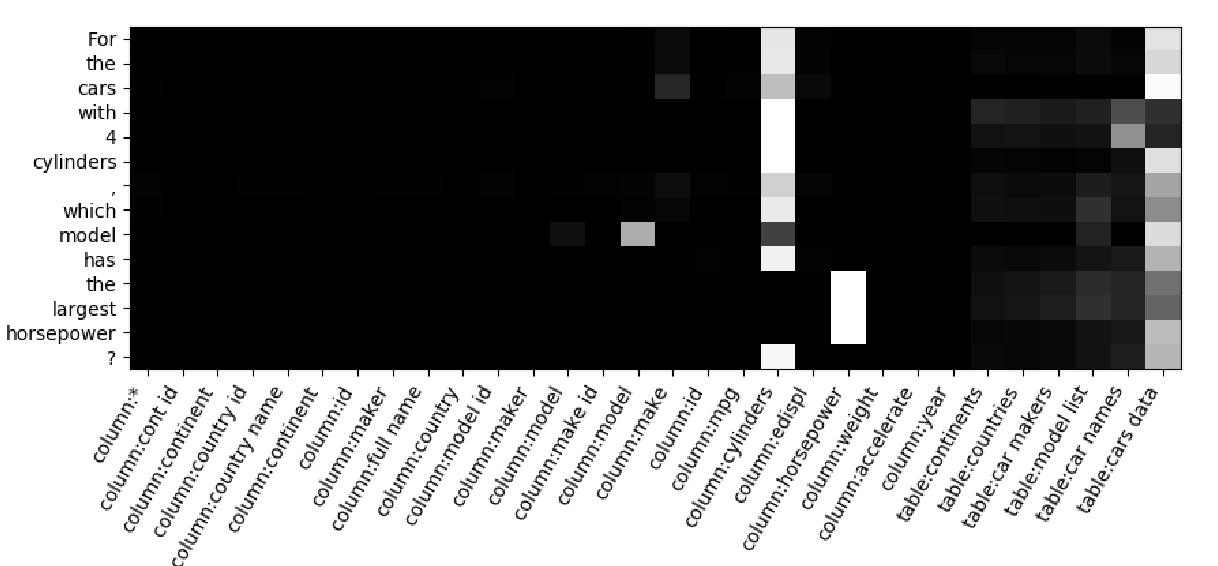