CorefQA: Coreference Resolution as Query-based Span Prediction
Wei Wu, Fei Wang, Arianna Yuan, Fei Wu, Jiwei Li
NLP Applications Long Paper
Session 12A: Jul 8
(08:00-09:00 GMT)
Session 13A: Jul 8
(12:00-13:00 GMT)
Abstract:
In this paper, we present CorefQA, an accurate and extensible approach for the coreference resolution task. We formulate the problem as a span prediction task, like in question answering: A query is generated for each candidate mention using its surrounding context, and a span prediction module is employed to extract the text spans of the coreferences within the document using the generated query. This formulation comes with the following key advantages: (1) The span prediction strategy provides the flexibility of retrieving mentions left out at the mention proposal stage; (2) In the question answering framework, encoding the mention and its context explicitly in a query makes it possible to have a deep and thorough examination of cues embedded in the context of coreferent mentions; and (3) A plethora of existing question answering datasets can be used for data augmentation to improve the model's generalization capability. Experiments demonstrate significant performance boost over previous models, with 83.1 (+3.5) F1 score on the CoNLL-2012 benchmark and 87.5 (+2.5) F1 score on the GAP benchmark.
You can open the
pre-recorded video
in a separate window.
NOTE: The SlidesLive video may display a random order of the authors.
The correct author list is shown at the top of this webpage.
Similar Papers
SpanBERT: Improving Pre-training by Representing and Predicting Spans
Mandar Joshi, Danqi Chen, Yinhan Liu, Daniel S. Weld, Luke Zettlemoyer, Omer Levy,
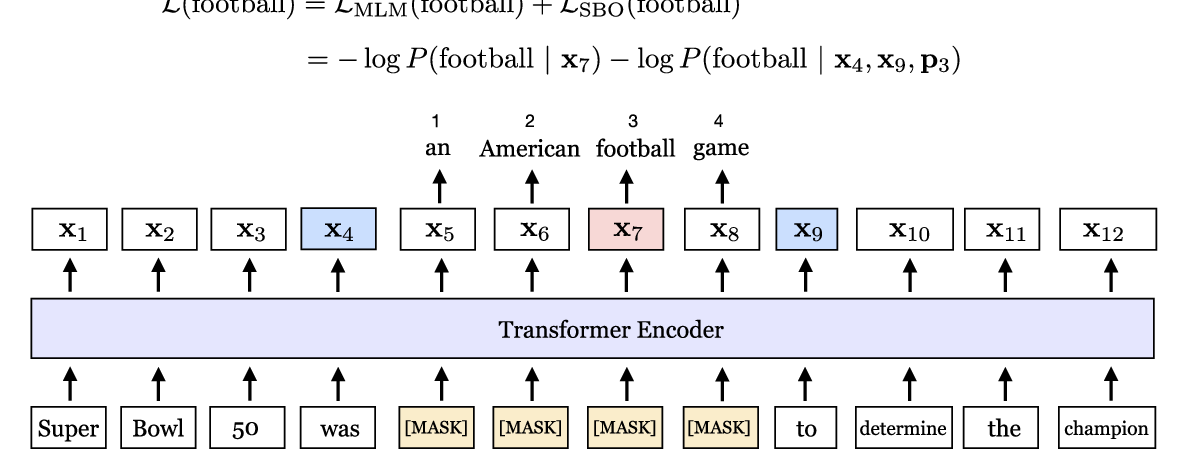
Active Learning for Coreference Resolution using Discrete Annotation
Belinda Z. Li, Gabriel Stanovsky, Luke Zettlemoyer,
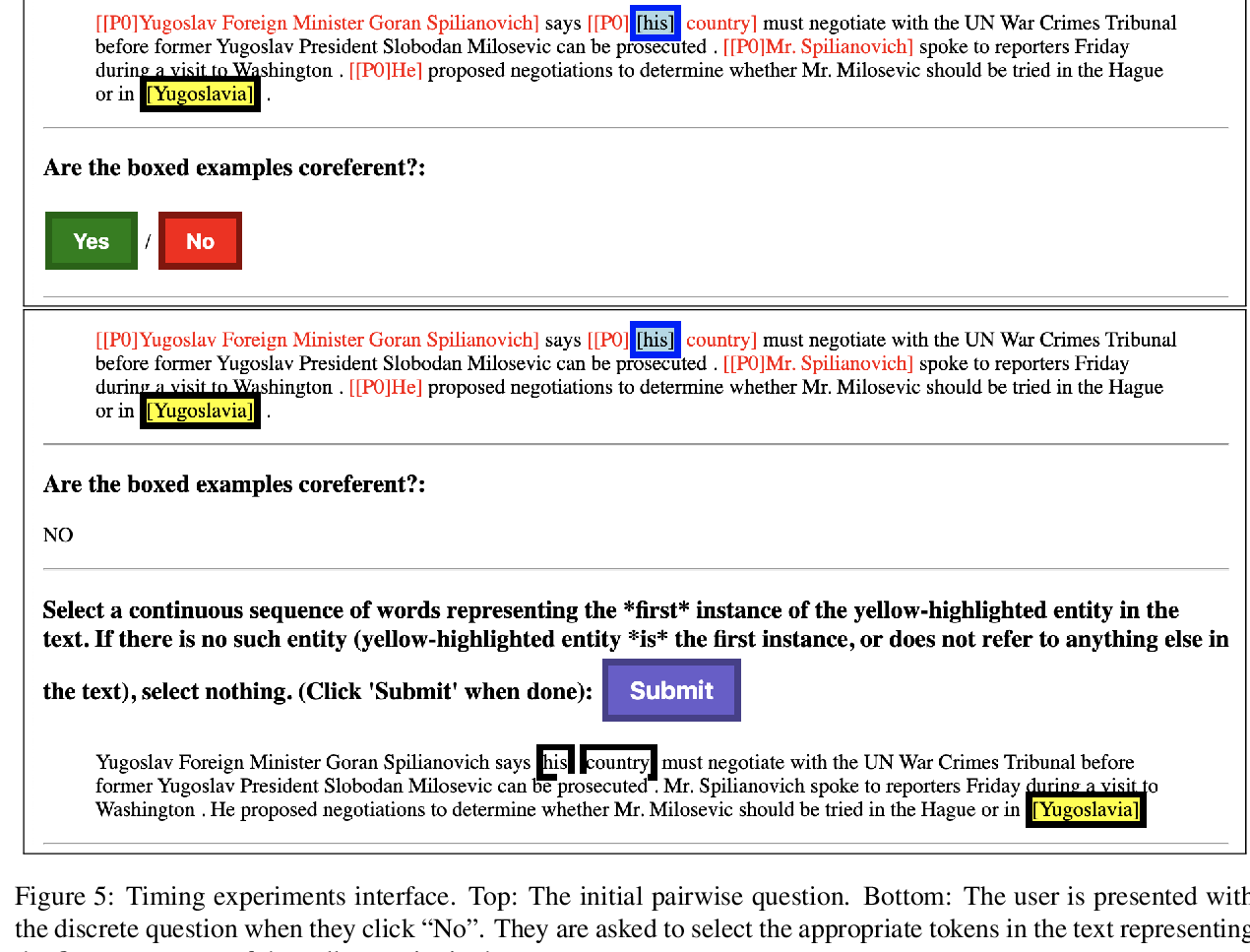
Span-based Localizing Network for Natural Language Video Localization
Hao Zhang, Aixin Sun, Wei Jing, Joey Tianyi Zhou,
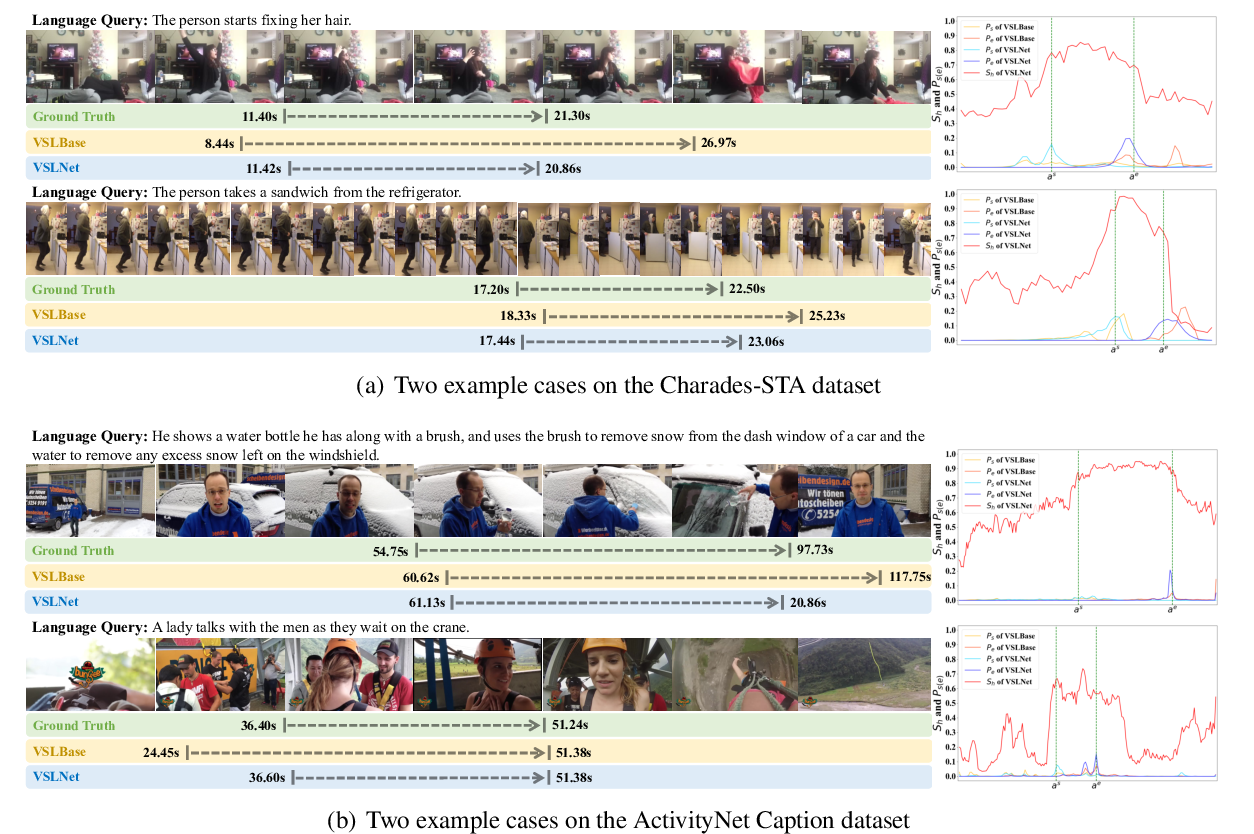