Active Learning for Coreference Resolution using Discrete Annotation
Belinda Z. Li, Gabriel Stanovsky, Luke Zettlemoyer
Semantics: Sentence Level Short Paper
Session 14A: Jul 8
(17:00-18:00 GMT)
Session 15A: Jul 8
(20:00-21:00 GMT)
Abstract:
We improve upon pairwise annotation for active learning in coreference resolution, by asking annotators to identify mention antecedents if a presented mention pair is deemed not coreferent. This simple modification, when combined with a novel mention clustering algorithm for selecting which examples to label, is much more efficient in terms of the performance obtained per annotation budget. In experiments with existing benchmark coreference datasets, we show that the signal from this additional question leads to significant performance gains per human-annotation hour. Future work can use our annotation protocol to effectively develop coreference models for new domains. Our code is publicly available.
You can open the
pre-recorded video
in a separate window.
NOTE: The SlidesLive video may display a random order of the authors.
The correct author list is shown at the top of this webpage.
Similar Papers
CorefQA: Coreference Resolution as Query-based Span Prediction
Wei Wu, Fei Wang, Arianna Yuan, Fei Wu, Jiwei Li,
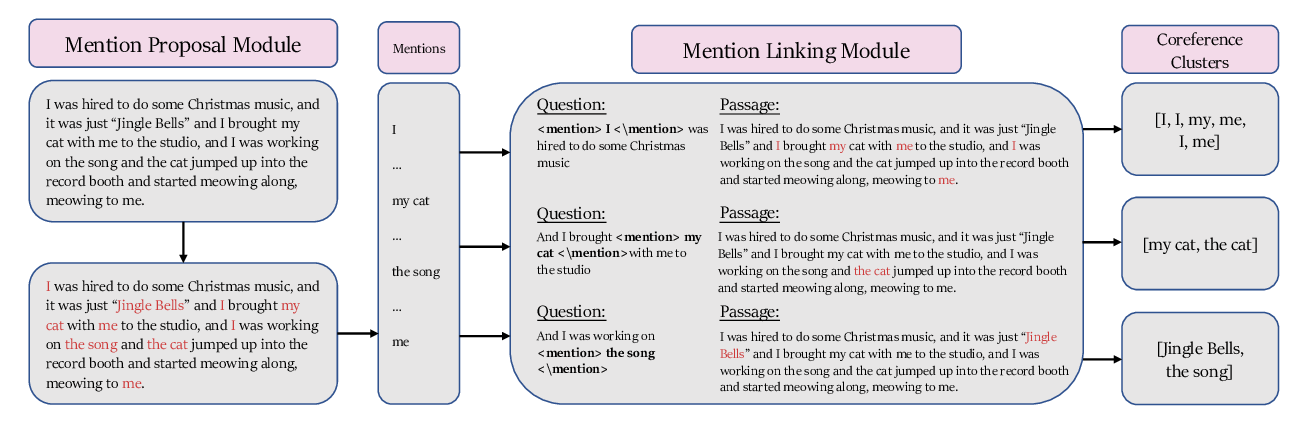
An Effective Transition-based Model for Discontinuous NER
Xiang Dai, Sarvnaz Karimi, Ben Hachey, Cecile Paris,
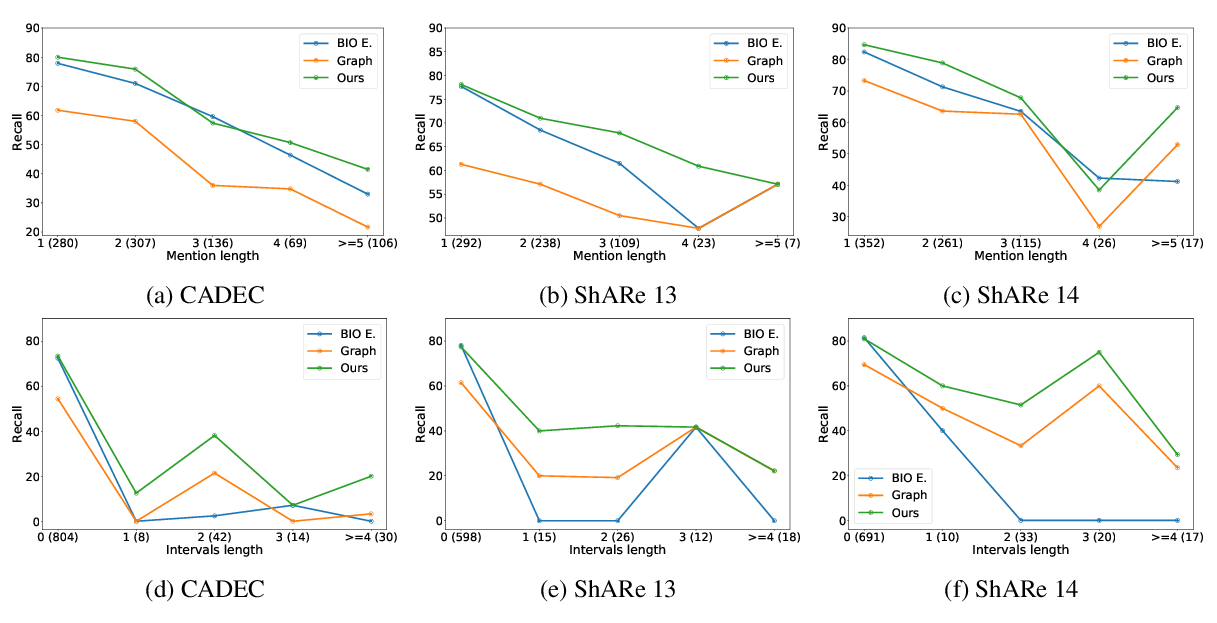
Learning to Contextually Aggregate Multi-Source Supervision for Sequence Labeling
Ouyu Lan, Xiao Huang, Bill Yuchen Lin, He Jiang, Liyuan Liu, Xiang Ren,
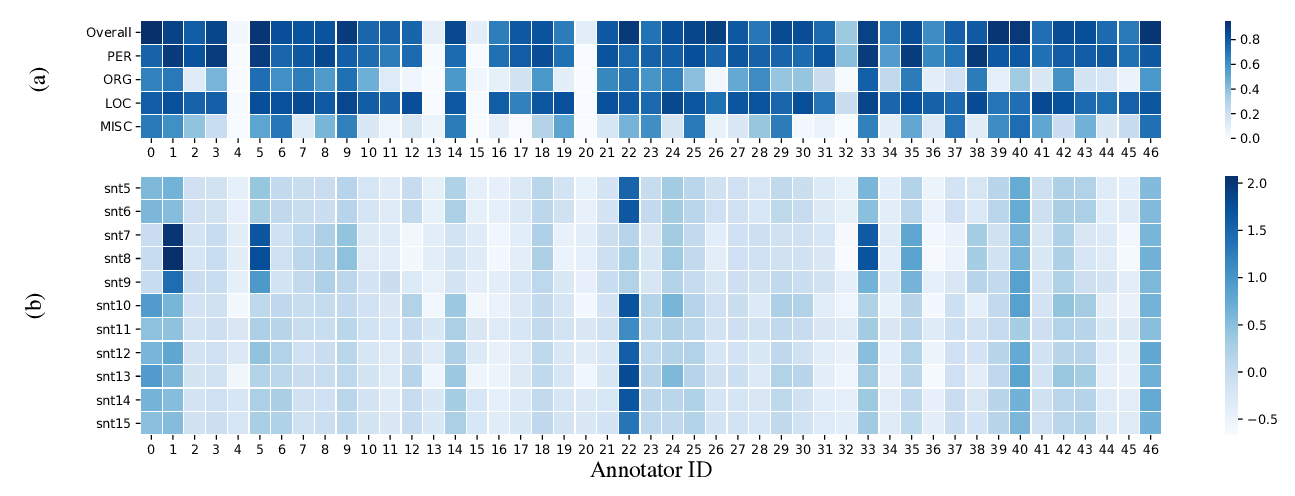