Span-based Localizing Network for Natural Language Video Localization
Hao Zhang, Aixin Sun, Wei Jing, Joey Tianyi Zhou
Language Grounding to Vision, Robotics and Beyond Long Paper
Session 11B: Jul 8
(06:00-07:00 GMT)
Session 12B: Jul 8
(09:00-10:00 GMT)
Abstract:
Given an untrimmed video and a text query, natural language video localization (NLVL) is to locate a matching span from the video that semantically corresponds to the query. Existing solutions formulate NLVL either as a ranking task and apply multimodal matching architecture, or as a regression task to directly regress the target video span. In this work, we address NLVL task with a span-based QA approach by treating the input video as text passage. We propose a video span localizing network (VSLNet), on top of the standard span-based QA framework, to address NLVL. The proposed VSLNet tackles the differences between NLVL and span-based QA through a simple and yet effective query-guided highlighting (QGH) strategy. The QGH guides VSLNet to search for matching video span within a highlighted region. Through extensive experiments on three benchmark datasets, we show that the proposed VSLNet outperforms the state-of-the-art methods; and adopting span-based QA framework is a promising direction to solve NLVL.
You can open the
pre-recorded video
in a separate window.
NOTE: The SlidesLive video may display a random order of the authors.
The correct author list is shown at the top of this webpage.
Similar Papers
Template-Based Question Generation from Retrieved Sentences for Improved Unsupervised Question Answering
Alexander Fabbri, Patrick Ng, Zhiguo Wang, Ramesh Nallapati, Bing Xiang,
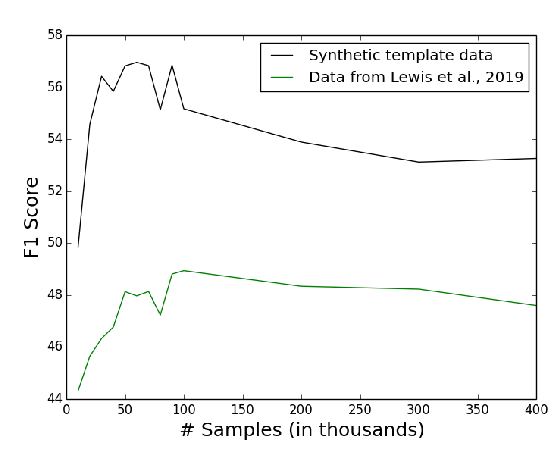
On the Importance of Diversity in Question Generation for QA
Md Arafat Sultan, Shubham Chandel, Ramón Fernandez Astudillo, Vittorio Castelli,
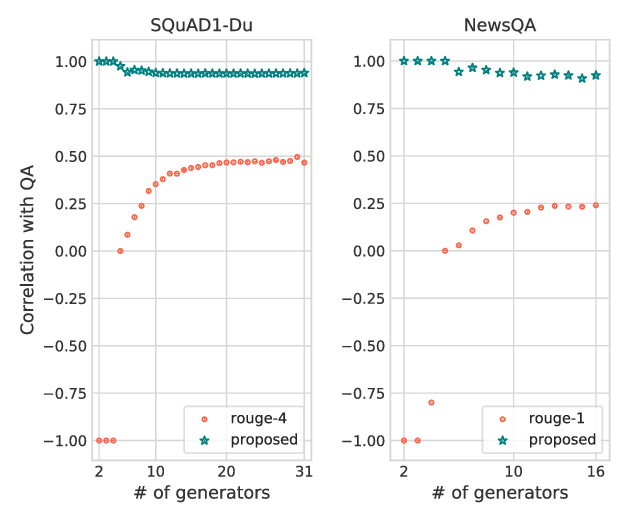
CorefQA: Coreference Resolution as Query-based Span Prediction
Wei Wu, Fei Wang, Arianna Yuan, Fei Wu, Jiwei Li,
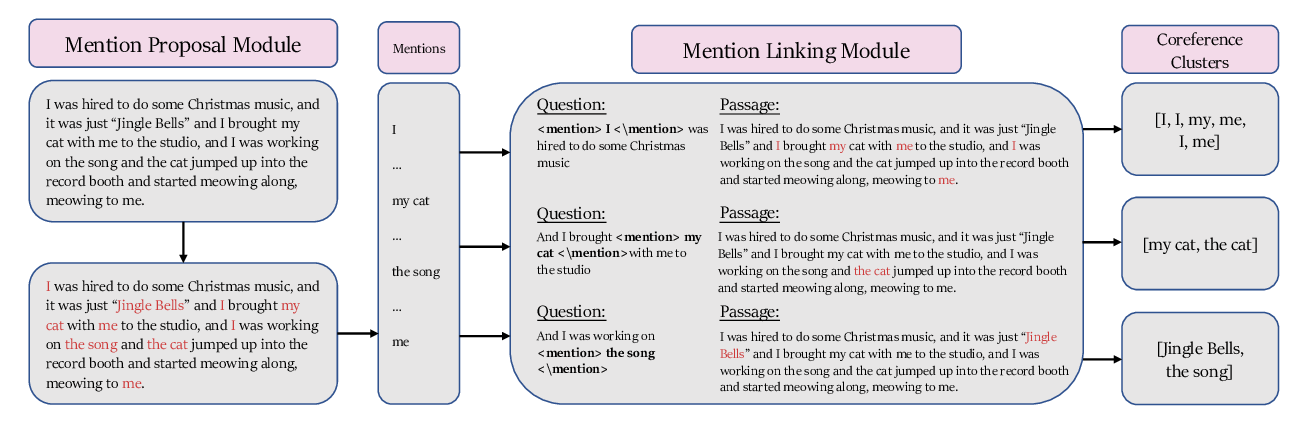
SpanBERT: Improving Pre-training by Representing and Predicting Spans
Mandar Joshi, Danqi Chen, Yinhan Liu, Daniel S. Weld, Luke Zettlemoyer, Omer Levy,
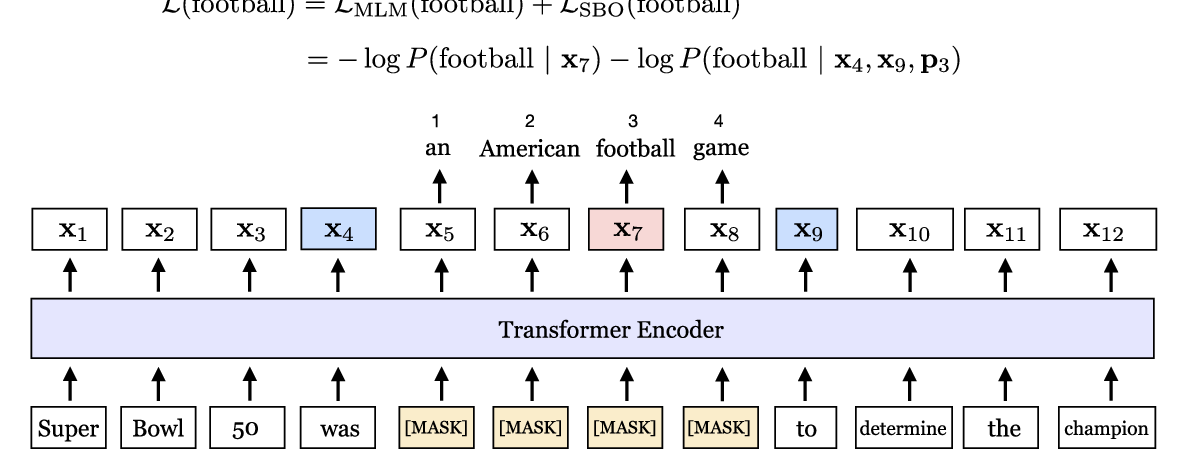