tBERT: Topic Models and BERT Joining Forces for Semantic Similarity Detection
Nicole Peinelt, Dong Nguyen, Maria Liakata
Semantics: Sentence Level Short Paper
Session 12A: Jul 8
(08:00-09:00 GMT)
Session 13A: Jul 8
(12:00-13:00 GMT)
Abstract:
Semantic similarity detection is a fundamental task in natural language understanding. Adding topic information has been useful for previous feature-engineered semantic similarity models as well as neural models for other tasks. There is currently no standard way of combining topics with pretrained contextual representations such as BERT. We propose a novel topic-informed BERT-based architecture for pairwise semantic similarity detection and show that our model improves performance over strong neural baselines across a variety of English language datasets. We find that the addition of topics to BERT helps particularly with resolving domain-specific cases.
You can open the
pre-recorded video
in a separate window.
NOTE: The SlidesLive video may display a random order of the authors.
The correct author list is shown at the top of this webpage.
Similar Papers
Distilling Knowledge Learned in BERT for Text Generation
Yen-Chun Chen, Zhe Gan, Yu Cheng, Jingzhou Liu, Jingjing Liu,
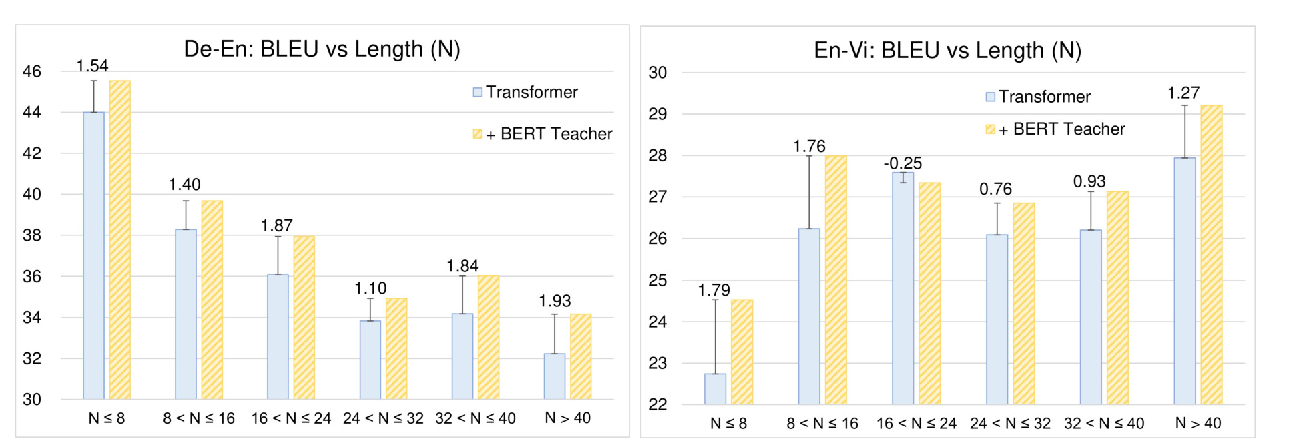
Fast and Accurate Deep Bidirectional Language Representations for Unsupervised Learning
Joongbo Shin, Yoonhyung Lee, Seunghyun Yoon, Kyomin Jung,
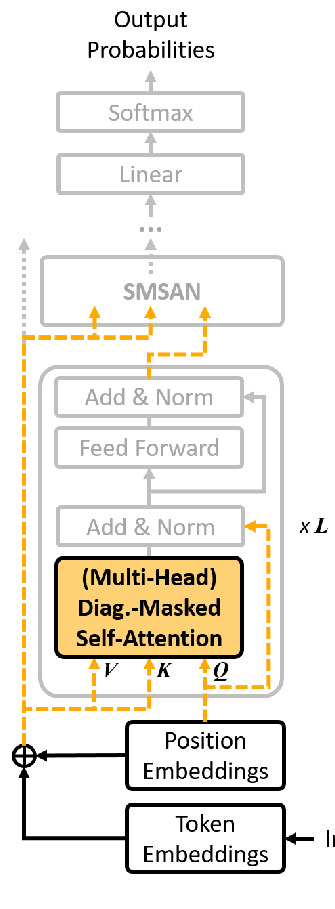
Span Selection Pre-training for Question Answering
Michael Glass, Alfio Gliozzo, Rishav Chakravarti, Anthony Ferritto, Lin Pan, G P Shrivatsa Bhargav, Dinesh Garg, Avi Sil,
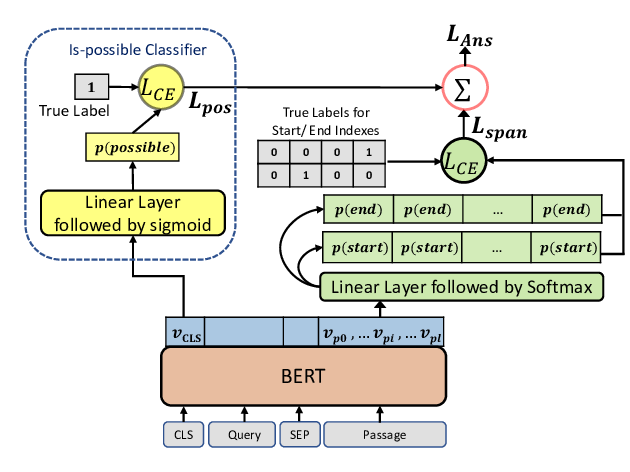
Tree-Structured Neural Topic Model
Masaru Isonuma, Junichiro Mori, Danushka Bollegala, Ichiro Sakata,
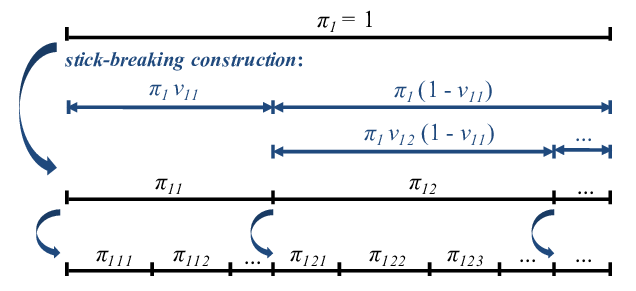