Aligned Dual Channel Graph Convolutional Network for Visual Question Answering
Qingbao Huang, Jielong Wei, Yi Cai, Changmeng Zheng, Junying Chen, Ho-fung Leung, Qing Li
Language Grounding to Vision, Robotics and Beyond Long Paper
Session 12B: Jul 8
(09:00-10:00 GMT)
Session 13B: Jul 8
(13:00-14:00 GMT)
Abstract:
Visual question answering aims to answer the natural language question about a given image. Existing graph-based methods only focus on the relations between objects in an image and neglect the importance of the syntactic dependency relations between words in a question. To simultaneously capture the relations between objects in an image and the syntactic dependency relations between words in a question, we propose a novel dual channel graph convolutional network (DC-GCN) for better combining visual and textual advantages. The DC-GCN model consists of three parts: an I-GCN module to capture the relations between objects in an image, a Q-GCN module to capture the syntactic dependency relations between words in a question, and an attention alignment module to align image representations and question representations. Experimental results show that our model achieves comparable performance with the state-of-the-art approaches.
You can open the
pre-recorded video
in a separate window.
NOTE: The SlidesLive video may display a random order of the authors.
The correct author list is shown at the top of this webpage.
Similar Papers
Cross-modal Coherence Modeling for Caption Generation
Malihe Alikhani, Piyush Sharma, Shengjie Li, Radu Soricut, Matthew Stone,
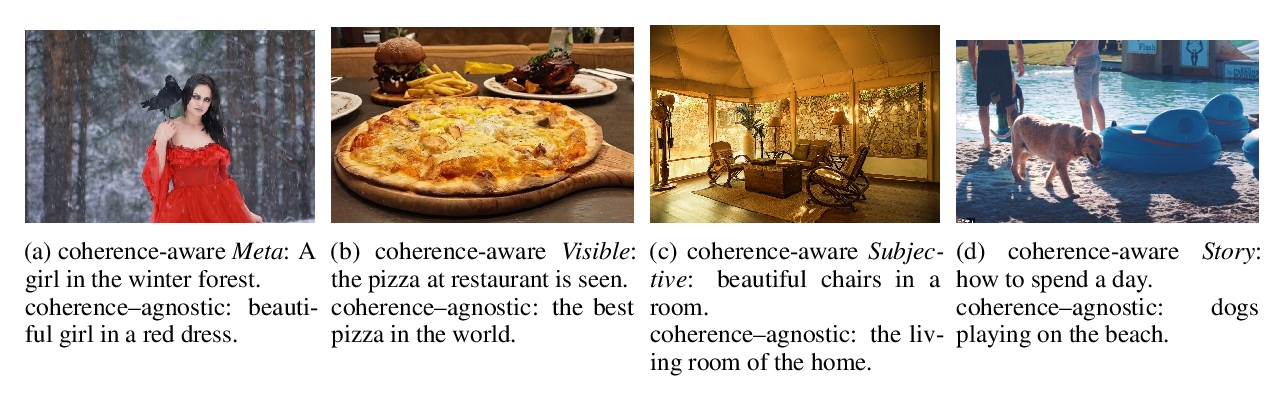
Heterogeneous Graph Transformer for Graph-to-Sequence Learning
Shaowei Yao, Tianming Wang, Xiaojun Wan,
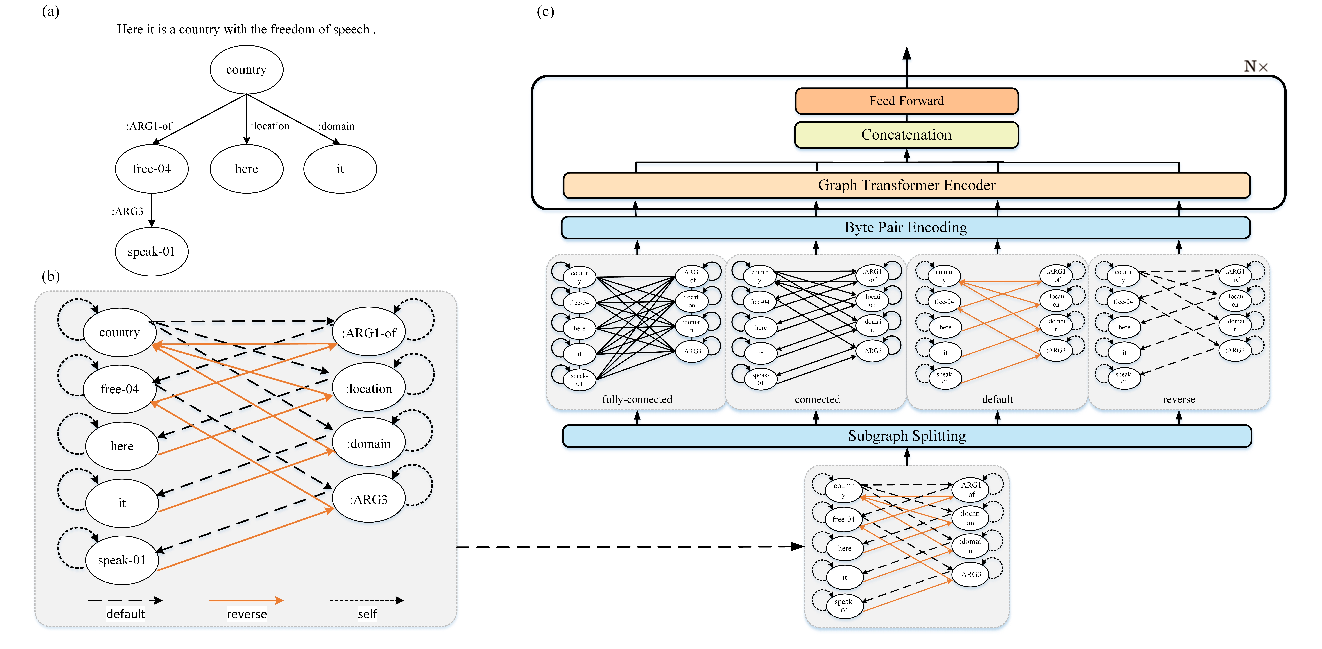