Improved Natural Language Generation via Loss Truncation
Daniel Kang, Tatsunori Hashimoto
Generation Long Paper
Session 1B: Jul 6
(06:00-07:00 GMT)
Session 5A: Jul 6
(20:00-21:00 GMT)
Abstract:
Neural language models are usually trained to match the distributional properties of large-scale corpora by minimizing the log loss. While straightforward to optimize, this approach forces the model to reproduce all variations in the dataset, including noisy and invalid references (e.g., misannotations and hallucinated facts). Even a small fraction of noisy data can degrade the performance of log loss. As an alternative, prior work has shown that minimizing the distinguishability of generated samples is a principled and robust loss that can handle invalid references. However, distinguishability has not been used in practice due to challenges in optimization and estimation. We propose loss truncation: a simple and scalable procedure which adaptively removes high log loss examples as a way to optimize for distinguishability. Empirically, we demonstrate that loss truncation outperforms existing baselines on distinguishability on a summarization task. Furthermore, we show that samples generated by the loss truncation model have factual accuracy ratings that exceed those of baselines and match human references.
You can open the
pre-recorded video
in a separate window.
NOTE: The SlidesLive video may display a random order of the authors.
The correct author list is shown at the top of this webpage.
Similar Papers
Don’t Say That! Making Inconsistent Dialogue Unlikely with Unlikelihood Training
Margaret Li, Stephen Roller, Ilia Kulikov, Sean Welleck, Y-Lan Boureau, Kyunghyun Cho, Jason Weston,
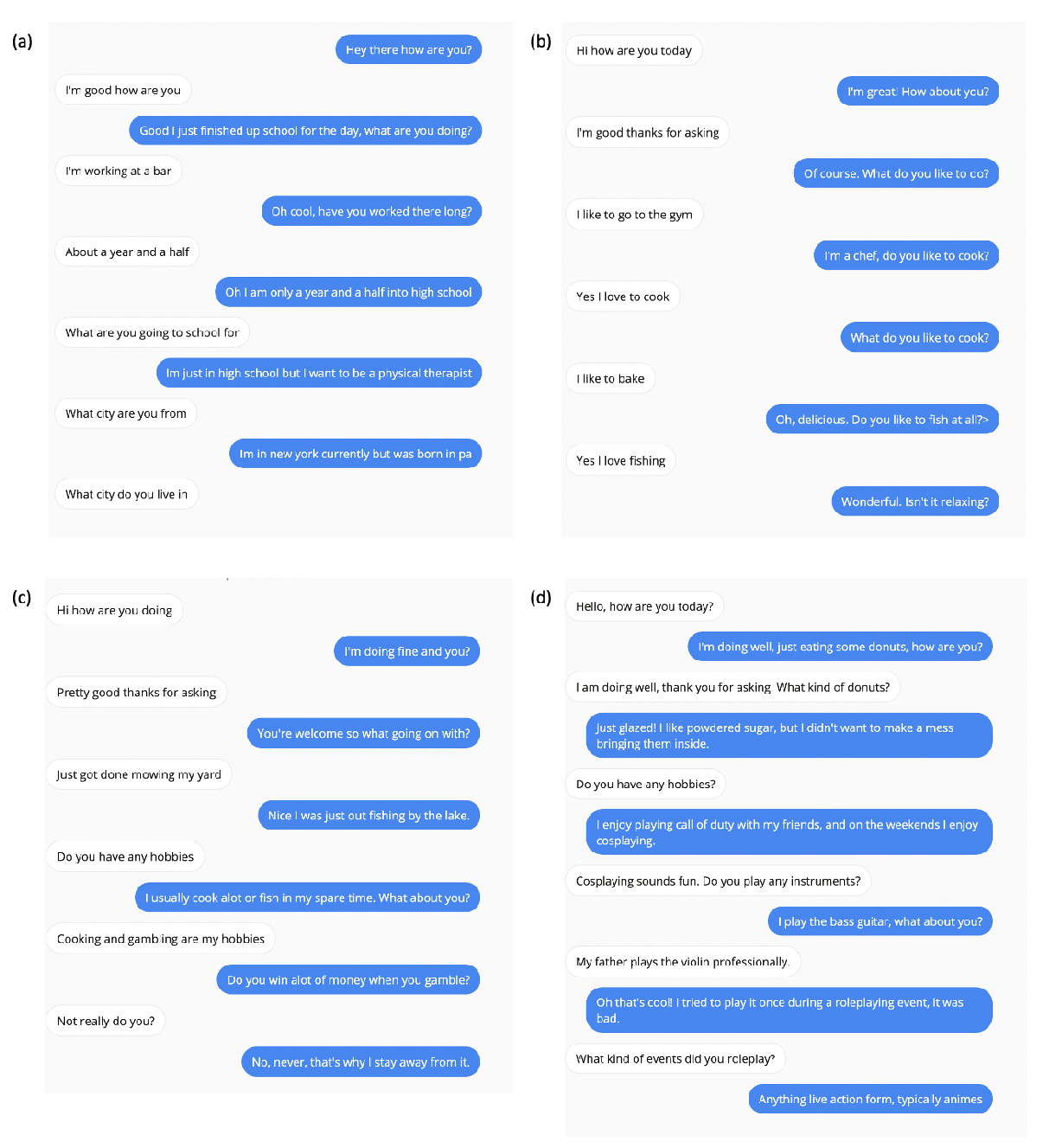
Data Manipulation: Towards Effective Instance Learning for Neural Dialogue Generation via Learning to Augment and Reweight
Hengyi Cai, Hongshen Chen, Yonghao Song, Cheng Zhang, Xiaofang Zhao, Dawei Yin,
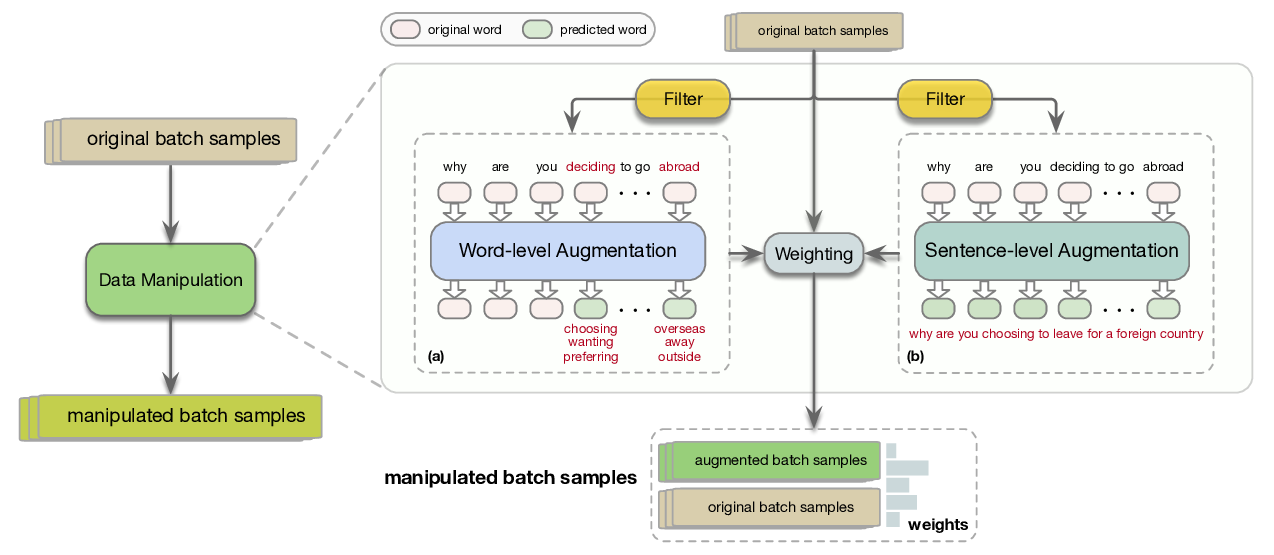
Evaluating Explainable AI: Which Algorithmic Explanations Help Users Predict Model Behavior?
Peter Hase, Mohit Bansal,
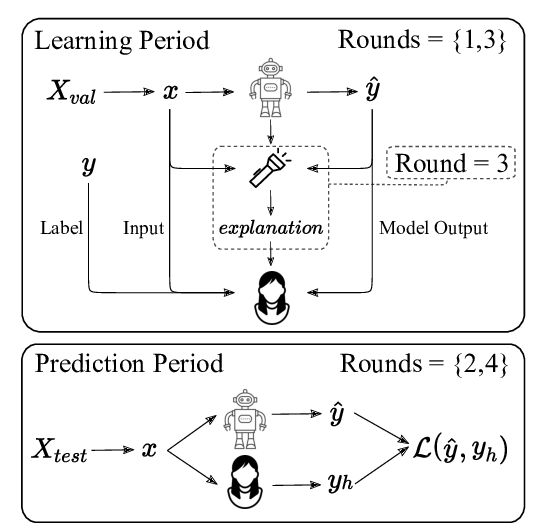
Learning to Deceive with Attention-Based Explanations
Danish Pruthi, Mansi Gupta, Bhuwan Dhingra, Graham Neubig, Zachary C. Lipton,
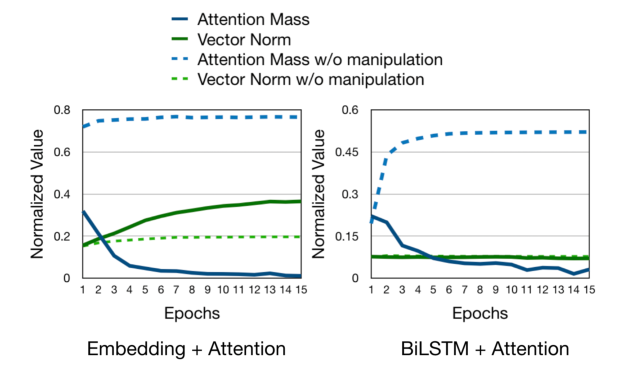