Temporally-Informed Analysis of Named Entity Recognition
Shruti Rijhwani, Daniel Preotiuc-Pietro
Information Extraction Long Paper
Session 13B: Jul 8
(13:00-14:00 GMT)
Session 15A: Jul 8
(20:00-21:00 GMT)
Abstract:
Natural language processing models often have to make predictions on text data that evolves over time as a result of changes in language use or the information described in the text. However, evaluation results on existing data sets are seldom reported by taking the timestamp of the document into account. We analyze and propose methods that make better use of temporally-diverse training data, with a focus on the task of named entity recognition. To support these experiments, we introduce a novel data set of English tweets annotated with named entities. We empirically demonstrate the effect of temporal drift on performance, and how the temporal information of documents can be used to obtain better models compared to those that disregard temporal information. Our analysis gives insights into why this information is useful, in the hope of informing potential avenues of improvement for named entity recognition as well as other NLP tasks under similar experimental setups.
You can open the
pre-recorded video
in a separate window.
NOTE: The SlidesLive video may display a random order of the authors.
The correct author list is shown at the top of this webpage.
Similar Papers
Soft Gazetteers for Low-Resource Named Entity Recognition
Shruti Rijhwani, Shuyan Zhou, Graham Neubig, Jaime Carbonell,
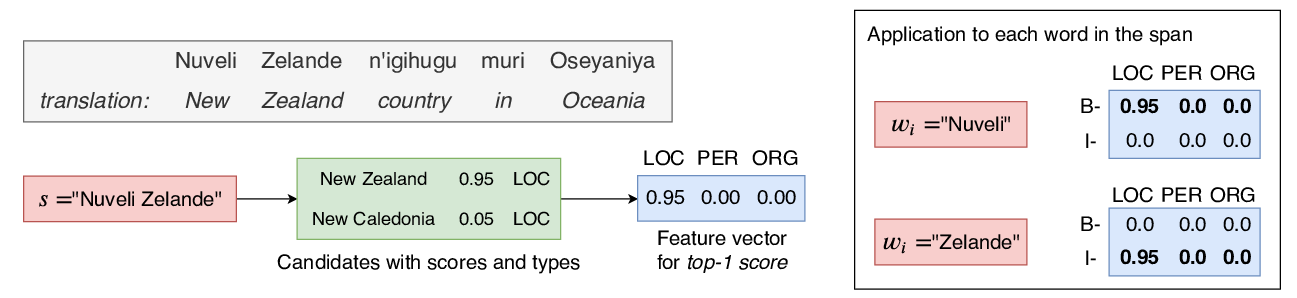
Predicting Performance for Natural Language Processing Tasks
Mengzhou Xia, Antonios Anastasopoulos, Ruochen Xu, Yiming Yang, Graham Neubig,
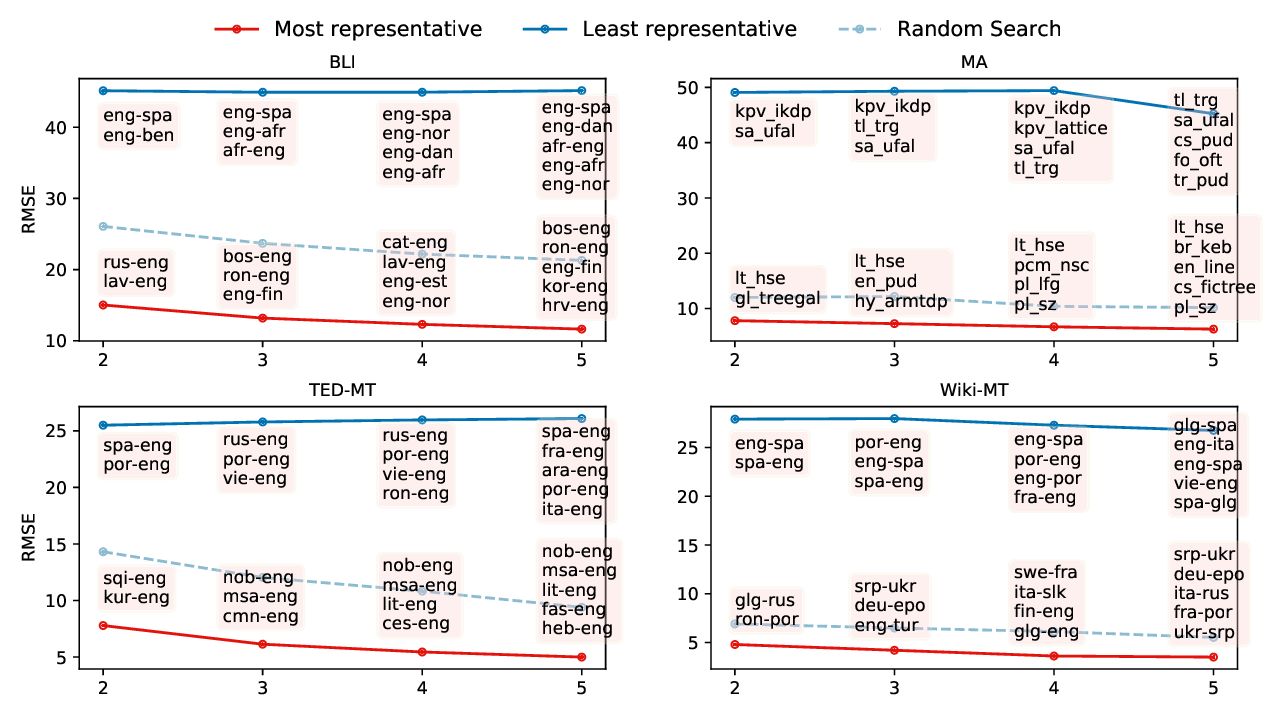
CamemBERT: a Tasty French Language Model
Louis Martin, Benjamin Muller, Pedro Javier Ortiz Suárez, Yoann Dupont, Laurent Romary, Éric de la Clergerie, Djamé Seddah, Benoît Sagot,
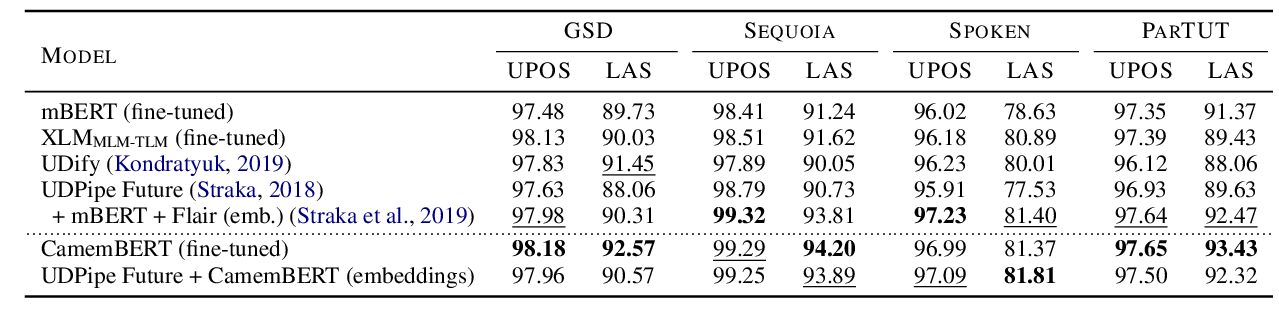
Temporal Common Sense Acquisition with Minimal Supervision
Ben Zhou, Qiang Ning, Daniel Khashabi, Dan Roth,
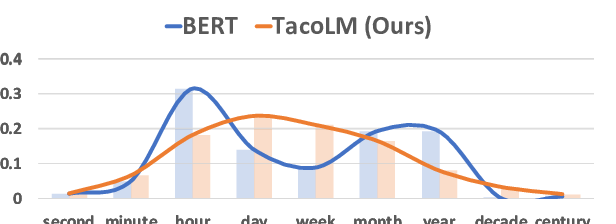