The Sensitivity of Language Models and Humans to Winograd Schema Perturbations
Mostafa Abdou, Vinit Ravishankar, Maria Barrett, Yonatan Belinkov, Desmond Elliott, Anders Søgaard
Semantics: Textual Inference and Other Areas of Semantics Long Paper
Session 13A: Jul 8
(12:00-13:00 GMT)
Session 14B: Jul 8
(18:00-19:00 GMT)
Abstract:
Large-scale pretrained language models are the major driving force behind recent improvements in perfromance on the Winograd Schema Challenge, a widely employed test of commonsense reasoning ability. We show, however, with a new diagnostic dataset, that these models are sensitive to linguistic perturbations of the Winograd examples that minimally affect human understanding. Our results highlight interesting differences between humans and language models: language models are more sensitive to number or gender alternations and synonym replacements than humans, and humans are more stable and consistent in their predictions, maintain a much higher absolute performance, and perform better on non-associative instances than associative ones.
You can open the
pre-recorded video
in a separate window.
NOTE: The SlidesLive video may display a random order of the authors.
The correct author list is shown at the top of this webpage.
Similar Papers
End-to-End Bias Mitigation by Modelling Biases in Corpora
Rabeeh Karimi Mahabadi, Yonatan Belinkov, James Henderson,
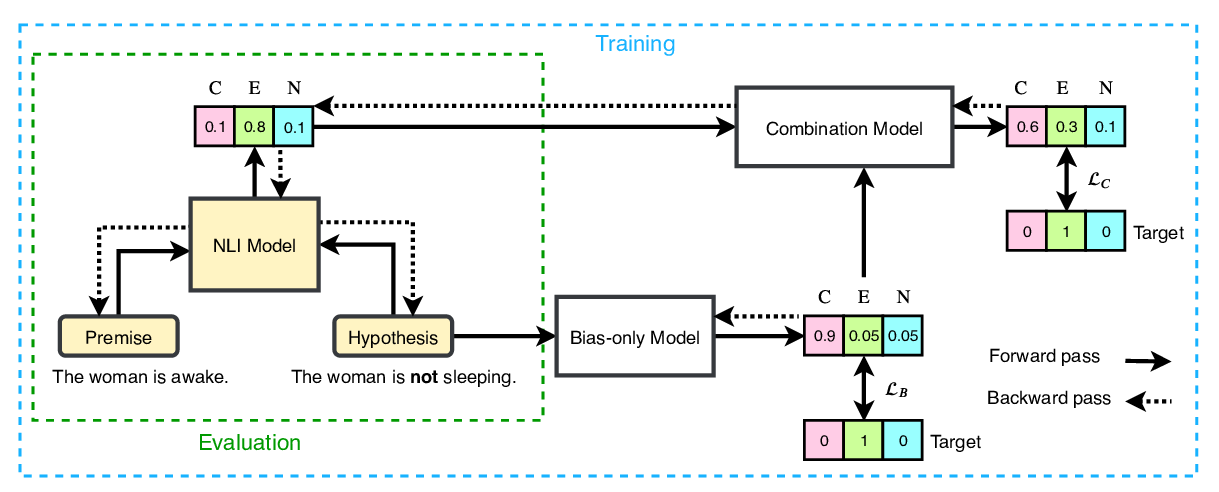
Pretrained Transformers Improve Out-of-Distribution Robustness
Dan Hendrycks, Xiaoyuan Liu, Eric Wallace, Adam Dziedzic, Rishabh Krishnan, Dawn Song,
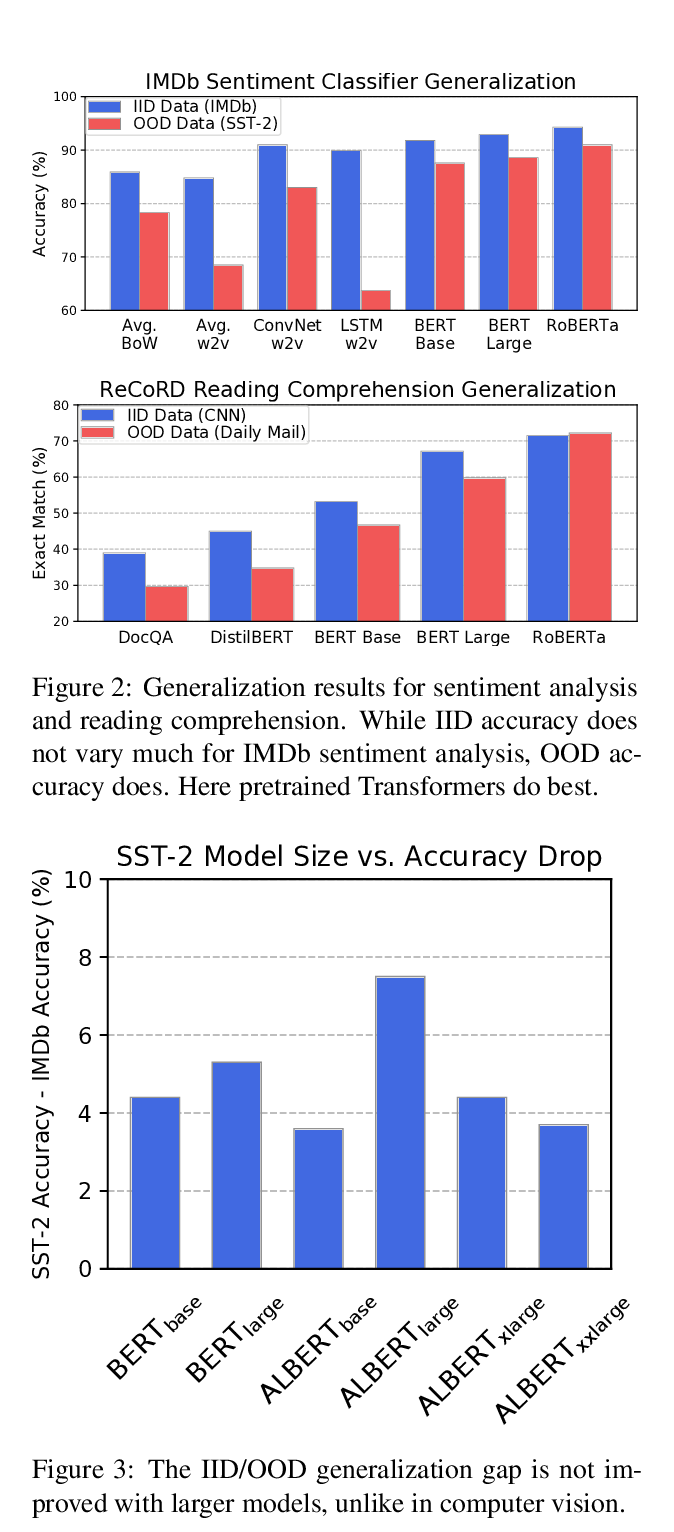
Reducing Gender Bias in Neural Machine Translation as a Domain Adaptation Problem
Danielle Saunders, Bill Byrne,
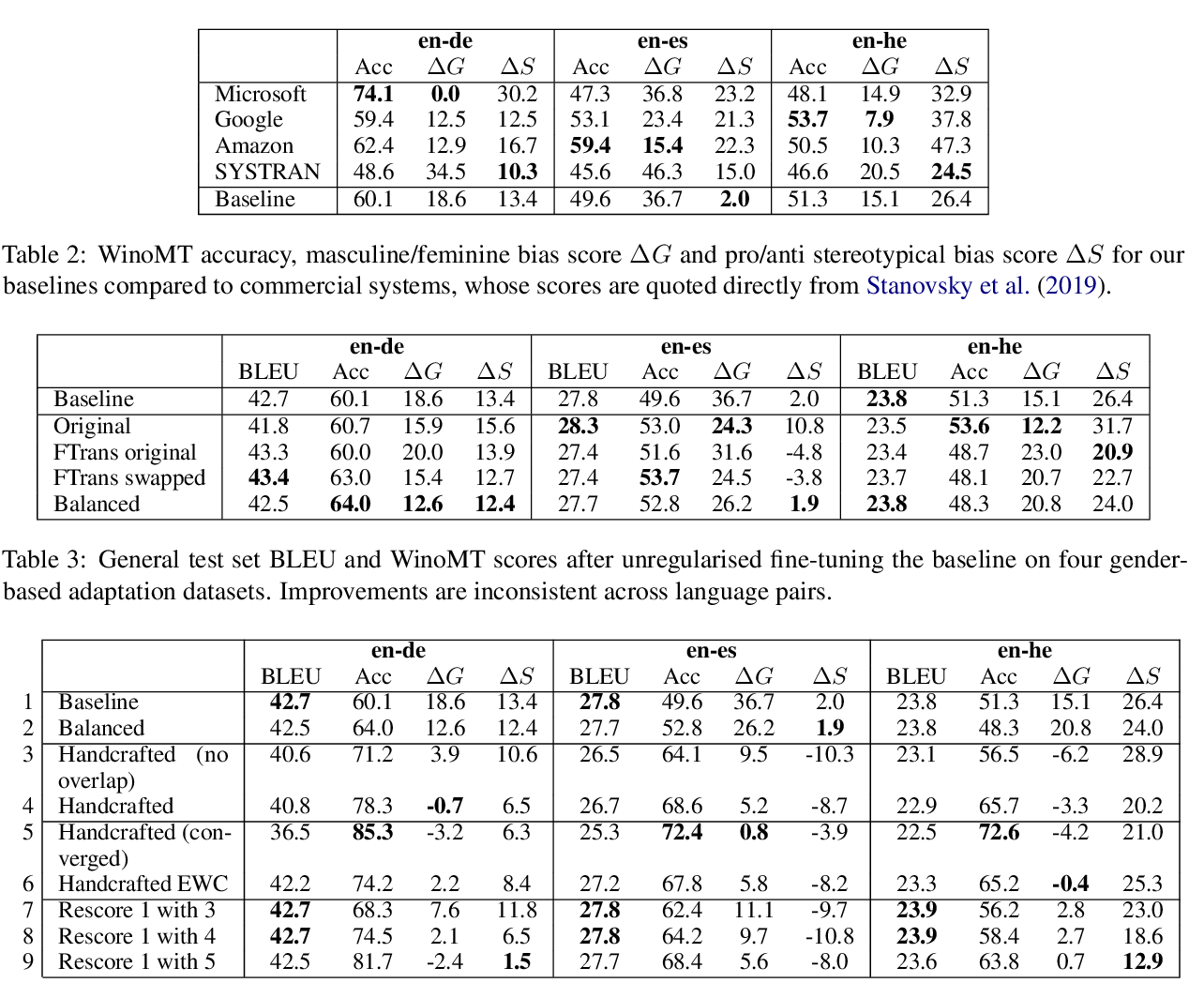
Knowledge Graph-Augmented Abstractive Summarization with Semantic-Driven Cloze Reward
Luyang Huang, Lingfei Wu, Lu Wang,
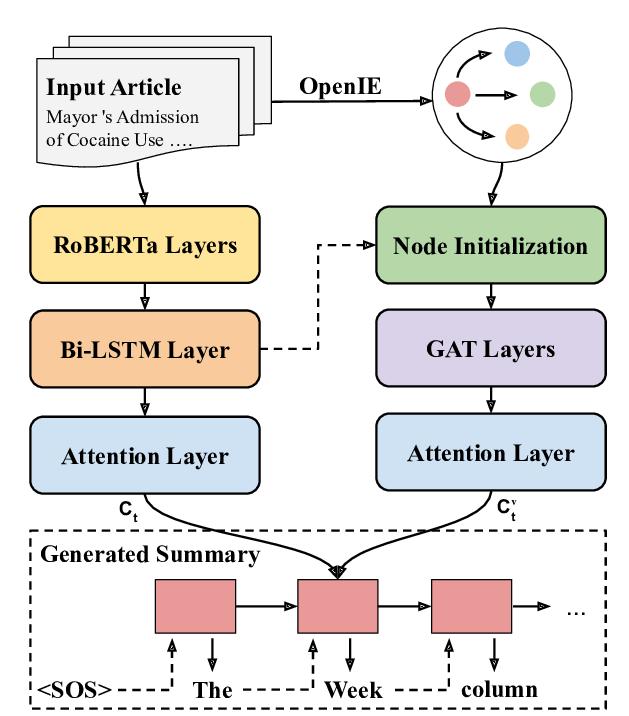