Learning Interpretable Relationships between Entities, Relations and Concepts via Bayesian Structure Learning on Open Domain Facts
Jingyuan Zhang, Mingming Sun, Yue Feng, Ping Li
Information Extraction Long Paper
Session 14A: Jul 8
(17:00-18:00 GMT)
Session 15A: Jul 8
(20:00-21:00 GMT)
Abstract:
Concept graphs are created as universal taxonomies for text understanding in the open-domain knowledge. The nodes in concept graphs include both entities and concepts. The edges are from entities to concepts, showing that an entity is an instance of a concept. In this paper, we propose the task of learning interpretable relationships from open-domain facts to enrich and refine concept graphs. The Bayesian network structures are learned from open-domain facts as the interpretable relationships between relations of facts and concepts of entities. We conduct extensive experiments on public English and Chinese datasets. Compared to the state-of-the-art methods, the learned network structures help improving the identification of concepts for entities based on the relations of entities on both datasets.
You can open the
pre-recorded video
in a separate window.
NOTE: The SlidesLive video may display a random order of the authors.
The correct author list is shown at the top of this webpage.
Similar Papers
A Generate-and-Rank Framework with Semantic Type Regularization for Biomedical Concept Normalization
Dongfang Xu, Zeyu Zhang, Steven Bethard,
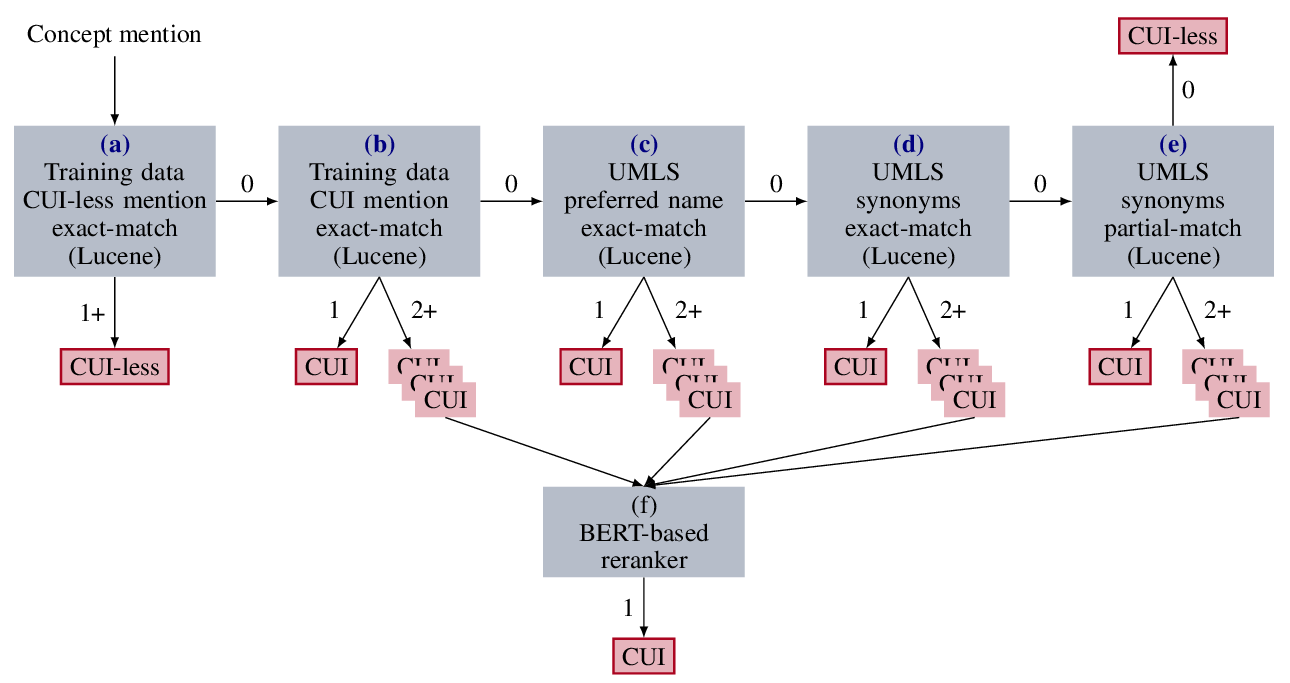
Grounded Conversation Generation as Guided Traverses in Commonsense Knowledge Graphs
Houyu Zhang, Zhenghao Liu, Chenyan Xiong, Zhiyuan Liu,
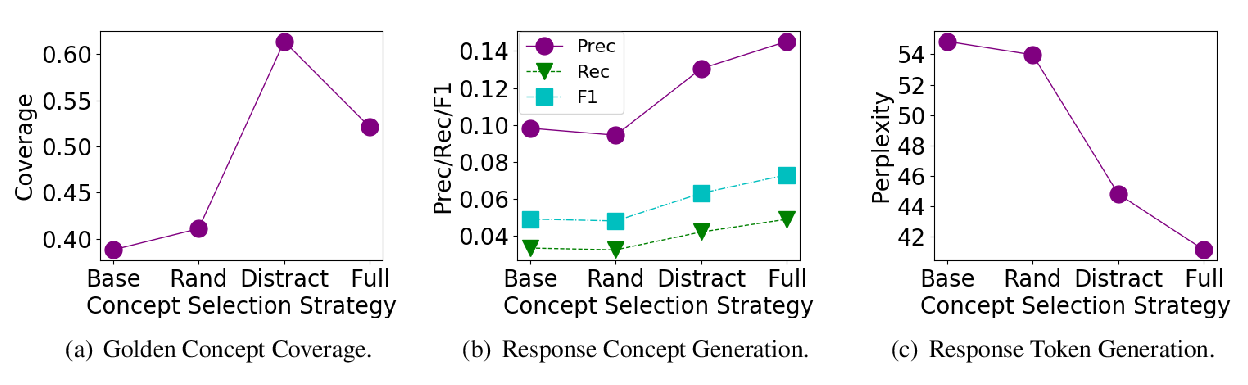
KinGDOM: Knowledge-Guided DOMain Adaptation for Sentiment Analysis
Deepanway Ghosal, Devamanyu Hazarika, Abhinaba Roy, Navonil Majumder, Rada Mihalcea, Soujanya Poria,
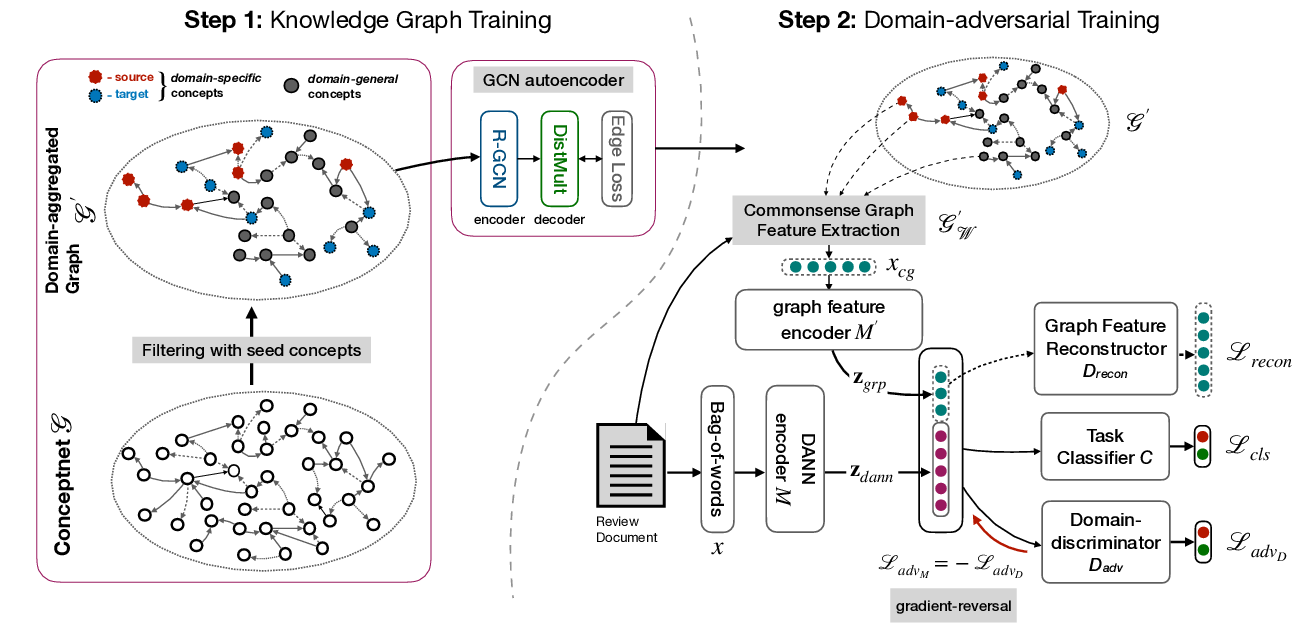
Clinical Concept Linking with Contextualized Neural Representations
Elliot Schumacher, Andriy Mulyar, Mark Dredze,
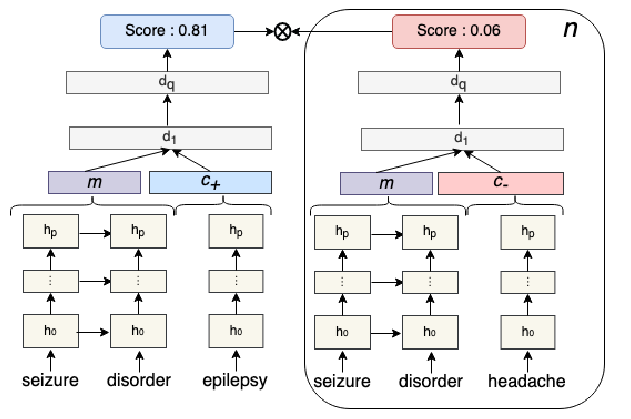