A Generate-and-Rank Framework with Semantic Type Regularization for Biomedical Concept Normalization
Dongfang Xu, Zeyu Zhang, Steven Bethard
Information Extraction Long Paper
Session 14B: Jul 8
(18:00-19:00 GMT)
Session 15A: Jul 8
(20:00-21:00 GMT)
Abstract:
Concept normalization, the task of linking textual mentions of concepts to concepts in an ontology, is challenging because ontologies are large. In most cases, annotated datasets cover only a small sample of the concepts, yet concept normalizers are expected to predict all concepts in the ontology. In this paper, we propose an architecture consisting of a candidate generator and a list-wise ranker based on BERT. The ranker considers pairings of concept mentions and candidate concepts, allowing it to make predictions for any concept, not just those seen during training. We further enhance this list-wise approach with a semantic type regularizer that allows the model to incorporate semantic type information from the ontology during training. Our proposed concept normalization framework achieves state-of-the-art performance on multiple datasets.
You can open the
pre-recorded video
in a separate window.
NOTE: The SlidesLive video may display a random order of the authors.
The correct author list is shown at the top of this webpage.
Similar Papers
Learning Interpretable Relationships between Entities, Relations and Concepts via Bayesian Structure Learning on Open Domain Facts
Jingyuan Zhang, Mingming Sun, Yue Feng, Ping Li,
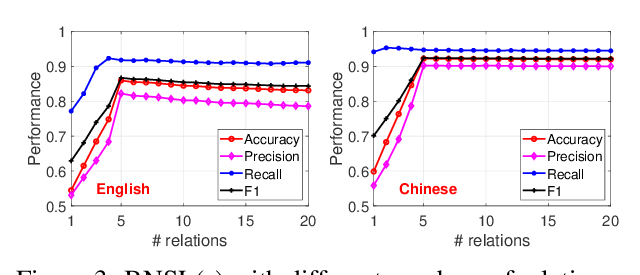
Clinical Concept Linking with Contextualized Neural Representations
Elliot Schumacher, Andriy Mulyar, Mark Dredze,
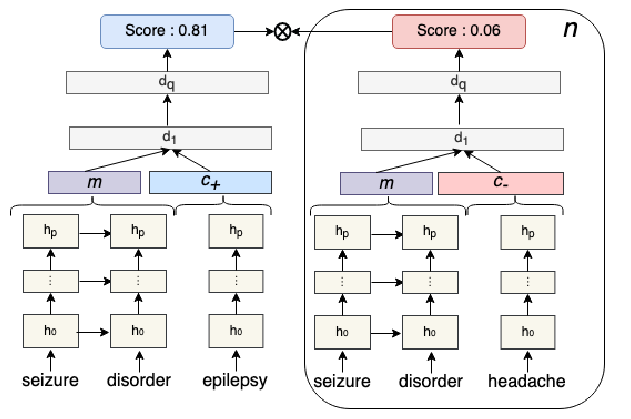
Grounded Conversation Generation as Guided Traverses in Commonsense Knowledge Graphs
Houyu Zhang, Zhenghao Liu, Chenyan Xiong, Zhiyuan Liu,
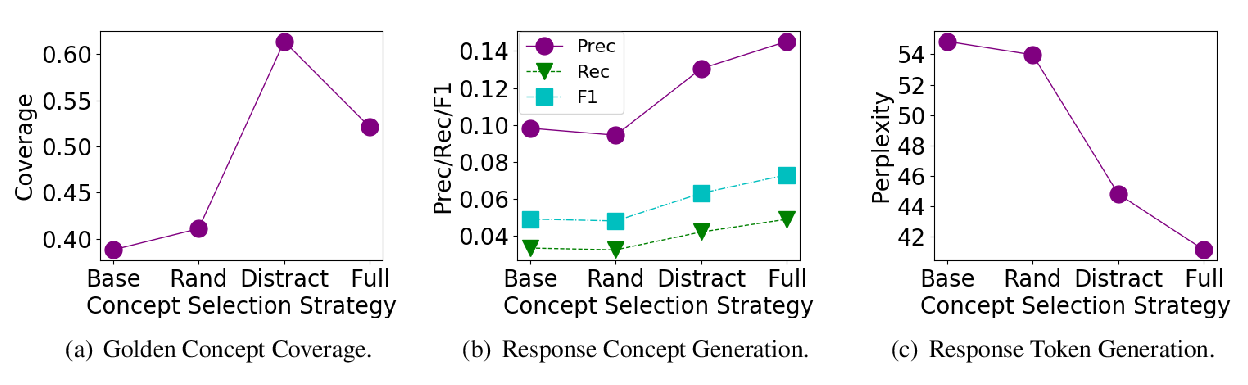
KinGDOM: Knowledge-Guided DOMain Adaptation for Sentiment Analysis
Deepanway Ghosal, Devamanyu Hazarika, Abhinaba Roy, Navonil Majumder, Rada Mihalcea, Soujanya Poria,
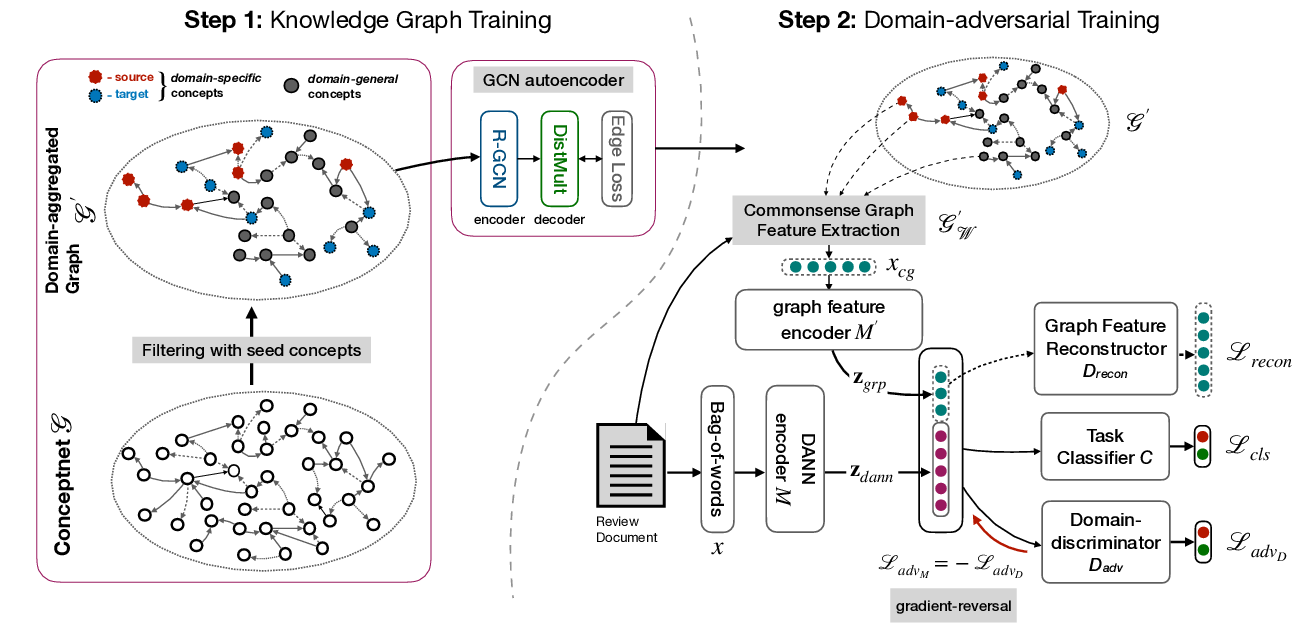