Soft Gazetteers for Low-Resource Named Entity Recognition
Shruti Rijhwani, Shuyan Zhou, Graham Neubig, Jaime Carbonell
Information Extraction Short Paper
Session 14A: Jul 8
(17:00-18:00 GMT)
Session 15B: Jul 8
(21:00-22:00 GMT)
Abstract:
Traditional named entity recognition models use gazetteers (lists of entities) as features to improve performance. Although modern neural network models do not require such hand-crafted features for strong performance, recent work has demonstrated their utility for named entity recognition on English data. However, designing such features for low-resource languages is challenging, because exhaustive entity gazetteers do not exist in these languages. To address this problem, we propose a method of ``soft gazetteers'' that incorporates ubiquitously available information from English knowledge bases, such as Wikipedia, into neural named entity recognition models through cross-lingual entity linking. Our experiments on four low-resource languages show an average improvement of 4 points in F1 score.
You can open the
pre-recorded video
in a separate window.
NOTE: The SlidesLive video may display a random order of the authors.
The correct author list is shown at the top of this webpage.
Similar Papers
Improving Candidate Generation for Low-resource Cross-lingual Entity Linking
Shuyan Zhou, Shruti Rijhwani, John Wieting, Jaime Carbonell, Graham Neubig,
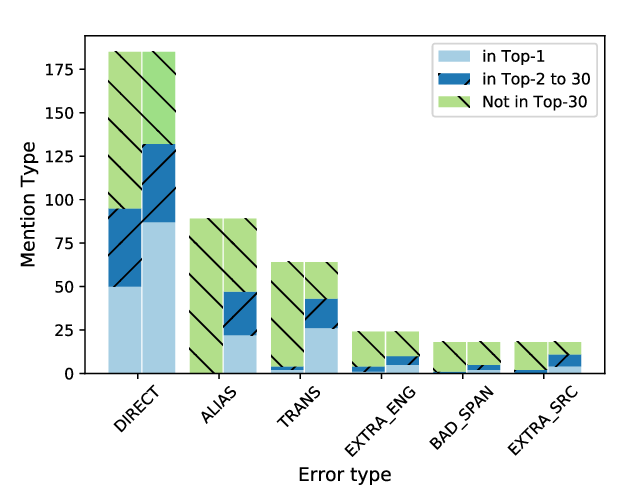
Improving Entity Linking through Semantic Reinforced Entity Embeddings
Feng Hou, Ruili Wang, Jun He, Yi Zhou,
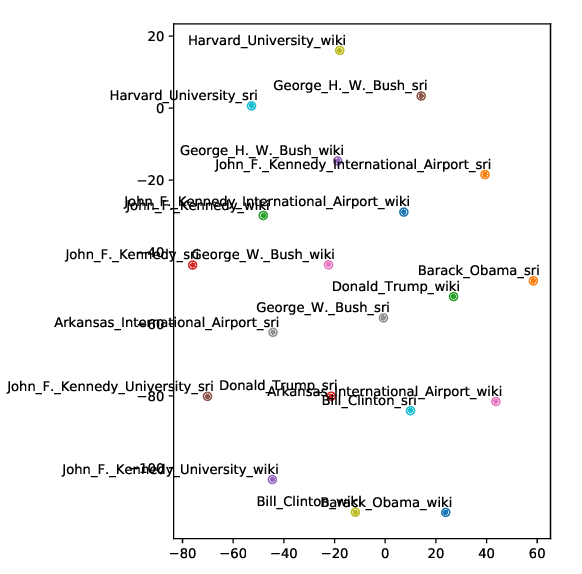