Improving Candidate Generation for Low-resource Cross-lingual Entity Linking
Shuyan Zhou, Shruti Rijhwani, John Wieting, Jaime Carbonell, Graham Neubig
Information Extraction TACL Paper
Session 13A: Jul 8
(12:00-13:00 GMT)
Session 14A: Jul 8
(17:00-18:00 GMT)
Abstract:
Cross-lingual entity linking (XEL) is the task of finding referents in a target-language knowledge base (KB) for mentions extracted from source-language texts. The first step of (X)EL is candidate generation, which retrieves a list of plausible candidate entities from the target-language KB for each mention. Approaches based on resources from Wikipedia have proven successful in the realm of relatively high-resource languages (HRL), but these do not extend well to low-resource languages (LRL) with few, if any, Wikipedia pages. Recently, transfer learning methods have been shown to reduce the demand for resources in the LRL by utilizing resources in closely-related languages, but the performance still lags far behind their high-resource counterparts. In this paper, we first assess the problems faced by current entity candidate generation methods for low-resource XEL, then propose three improvements that (1) reduce the disconnect between entity mentions and KB entries, and (2) improve the robustness of the model to low-resource scenarios. The methods are simple, but effective: we experiment with our approach on seven XEL datasets and find that they yield an average gain of 16.9% in Top-30 gold candidate recall, compared to state-of-the-art baselines. Our improved model also yields an average gain of 7.9% in in-KB accuracy of end-to-end XEL.
You can open the
pre-recorded video
in a separate window.
NOTE: The SlidesLive video may display a random order of the authors.
The correct author list is shown at the top of this webpage.
Similar Papers
Hypernymy Detection for Low-Resource Languages via Meta Learning
Changlong Yu, Jialong Han, Haisong Zhang, Wilfred Ng,
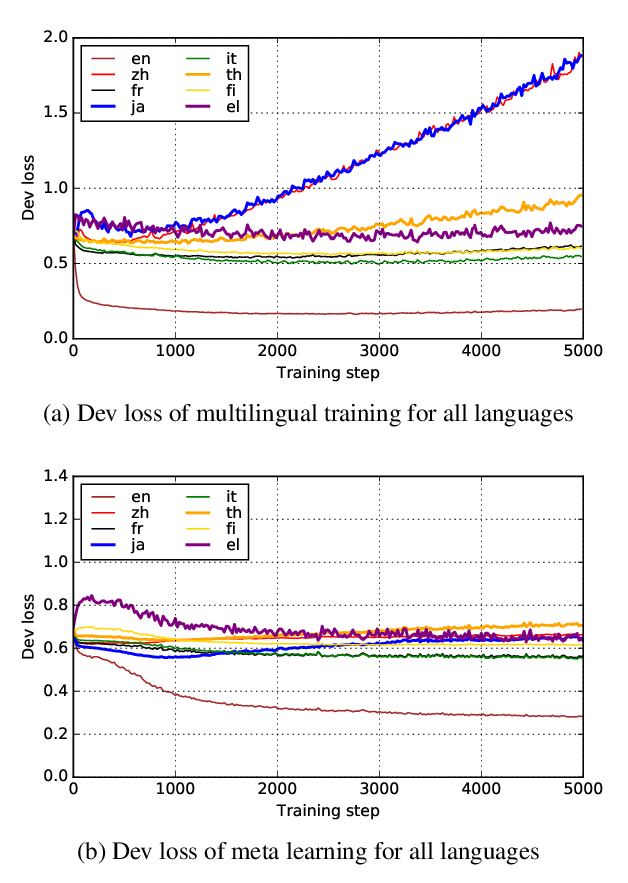
Soft Gazetteers for Low-Resource Named Entity Recognition
Shruti Rijhwani, Shuyan Zhou, Graham Neubig, Jaime Carbonell,
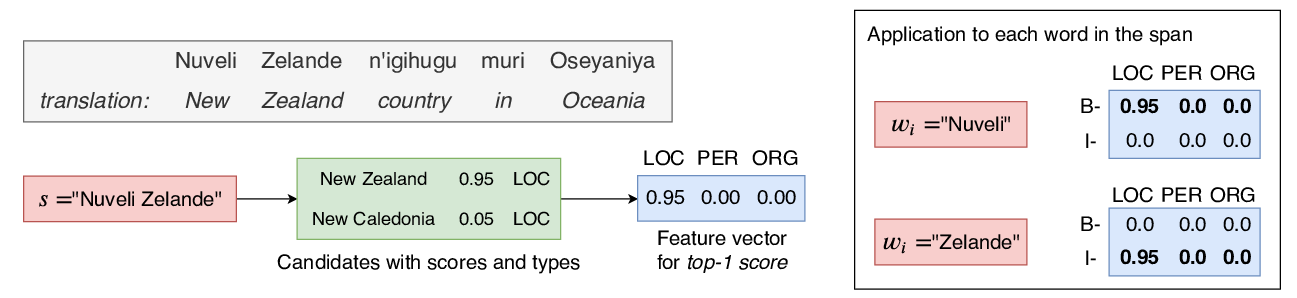
Clinical Concept Linking with Contextualized Neural Representations
Elliot Schumacher, Andriy Mulyar, Mark Dredze,
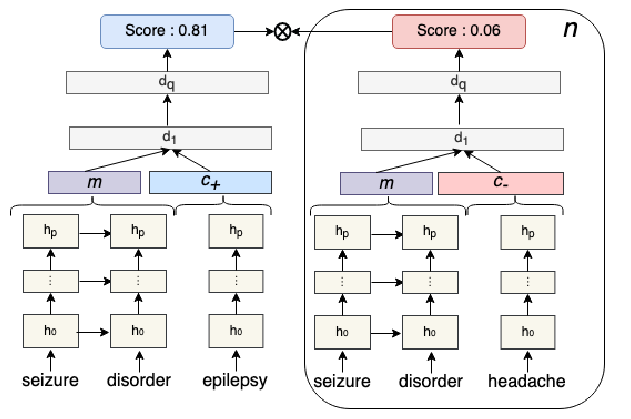
Leveraging Monolingual Data with Self-Supervision for Multilingual Neural Machine Translation
Aditya Siddhant, Ankur Bapna, Yuan Cao, Orhan Firat, Mia Chen, Sneha Kudugunta, Naveen Arivazhagan, Yonghui Wu,
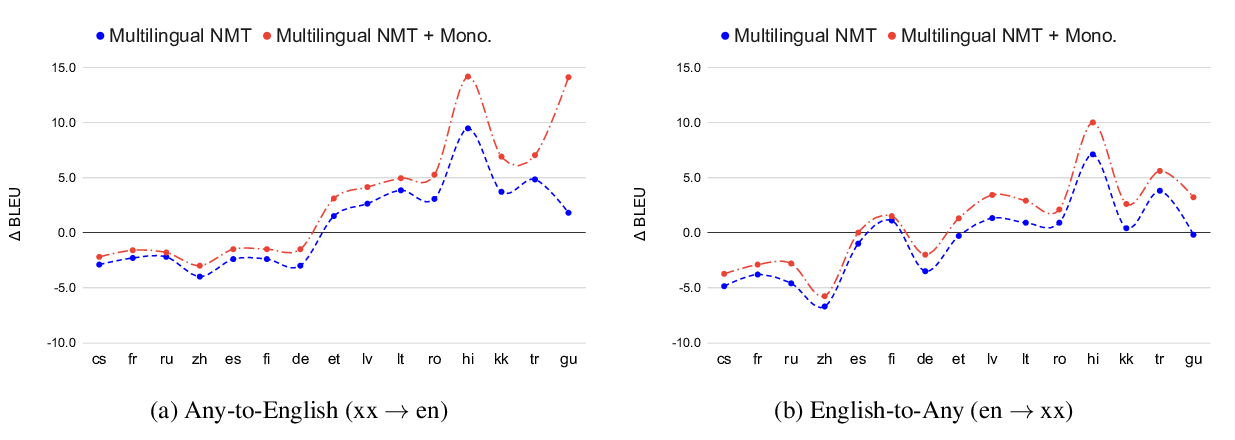