Structured Tuning for Semantic Role Labeling
Tao Li, Parth Anand Jawale, Martha Palmer, Vivek Srikumar
Semantics: Sentence Level Long Paper
Session 14A: Jul 8
(17:00-18:00 GMT)
Session 15B: Jul 8
(21:00-22:00 GMT)
Abstract:
Recent neural network-driven semantic role labeling (SRL) systems have shown impressive improvements in F1 scores. These improvements are due to expressive input representations, which, at least at the surface, are orthogonal to knowledge-rich constrained decoding mechanisms that helped linear SRL models. Introducing the benefits of structure to inform neural models presents a methodological challenge. In this paper, we present a structured tuning framework to improve models using softened constraints only at training time. Our framework leverages the expressiveness of neural networks and provides supervision with structured loss components. We start with a strong baseline (RoBERTa) to validate the impact of our approach, and show that our framework outperforms the baseline by learning to comply with declarative constraints. Additionally, our experiments with smaller training sizes show that we can achieve consistent improvements under low-resource scenarios.
You can open the
pre-recorded video
in a separate window.
NOTE: The SlidesLive video may display a random order of the authors.
The correct author list is shown at the top of this webpage.
Similar Papers
Lexically Constrained Neural Machine Translation with Levenshtein Transformer
Raymond Hendy Susanto, Shamil Chollampatt, Liling Tan,
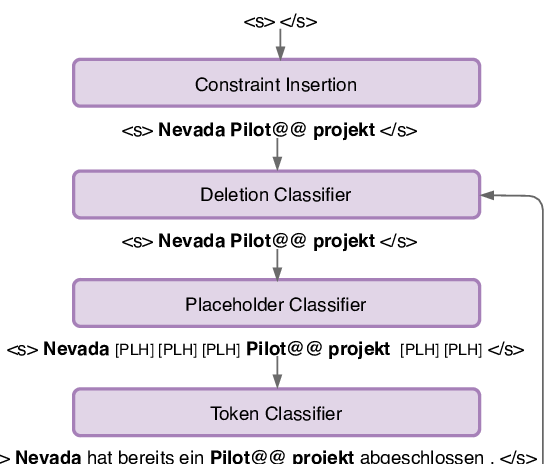
The Right Tool for the Job: Matching Model and Instance Complexities
Roy Schwartz, Gabriel Stanovsky, Swabha Swayamdipta, Jesse Dodge, Noah A. Smith,
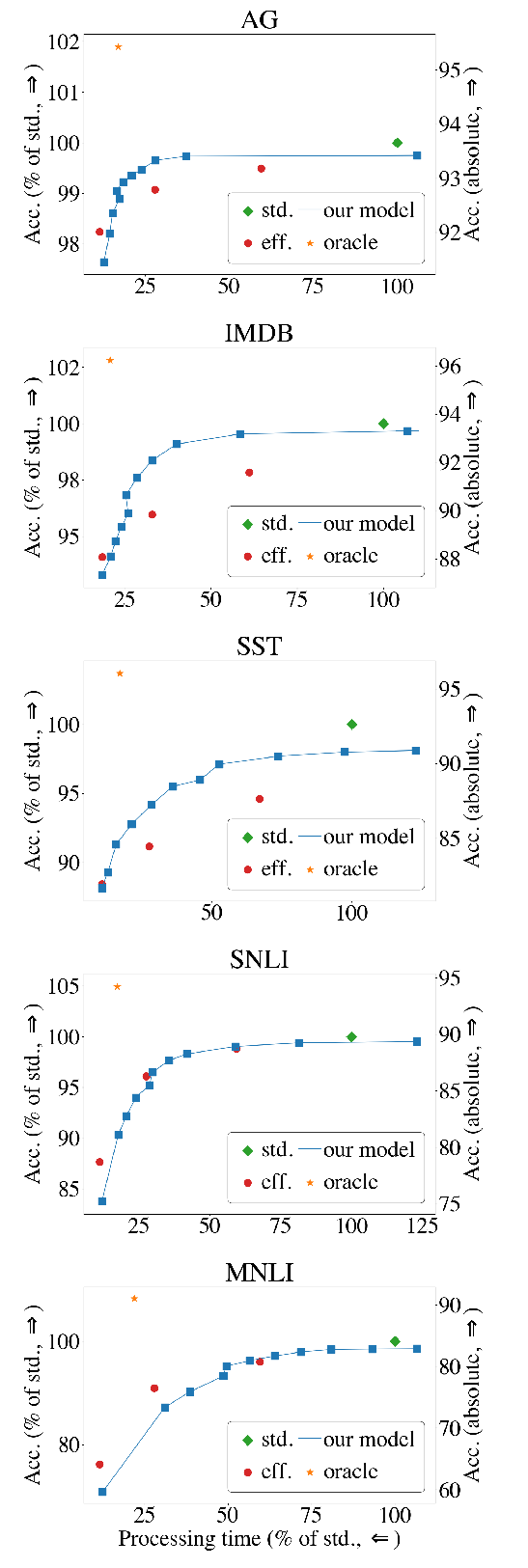
Learning Constraints for Structured Prediction Using Rectifier Networks
Xingyuan Pan, Maitrey Mehta, Vivek Srikumar,
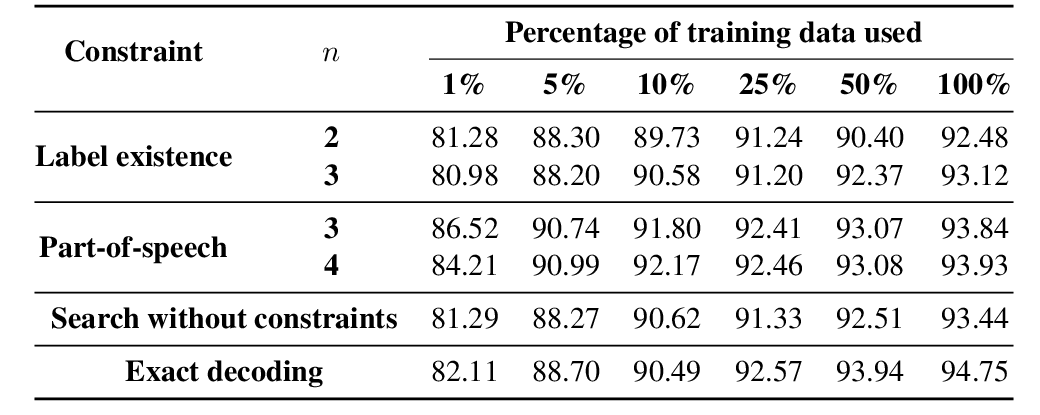
Generating Informative Conversational Response using Recurrent Knowledge-Interaction and Knowledge-Copy
Xiexiong Lin, Weiyu Jian, Jianshan He, Taifeng Wang, Wei Chu,
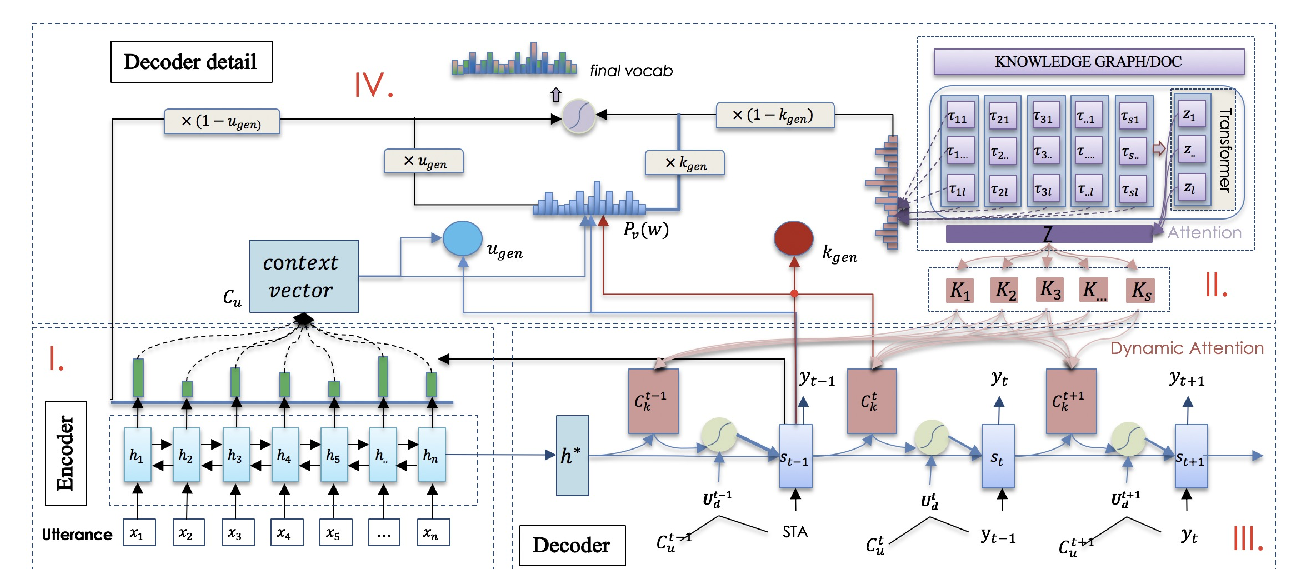