Understanding Advertisements with BERT
Kanika Kalra, Bhargav Kurma, Silpa Vadakkeeveetil Sreelatha, Manasi Patwardhan, Shirish Karande
NLP Applications Short Paper
Session 13A: Jul 8
(12:00-13:00 GMT)
Session 14B: Jul 8
(18:00-19:00 GMT)
Abstract:
We consider a task based on CVPR 2018 challenge dataset on advertisement (Ad) understanding. The task involves detecting the viewer's interpretation of an Ad image captured as text. Recent results have shown that the embedded scene-text in the image holds a vital cue for this task. Motivated by this, we fine-tune the base BERT model for a sentence-pair classification task. Despite utilizing the scene-text as the only source of visual information, we could achieve a hit-or-miss accuracy of 84.95% on the challenge test data. To enable BERT to process other visual information, we append image captions to the scene-text. This achieves an accuracy of 89.69%, which is an improvement of 4.7%. This is the best reported result for this task.
You can open the
pre-recorded video
in a separate window.
NOTE: The SlidesLive video may display a random order of the authors.
The correct author list is shown at the top of this webpage.
Similar Papers
Improving Image Captioning Evaluation by Considering Inter References Variance
Yanzhi Yi, Hangyu Deng, Jinglu Hu,
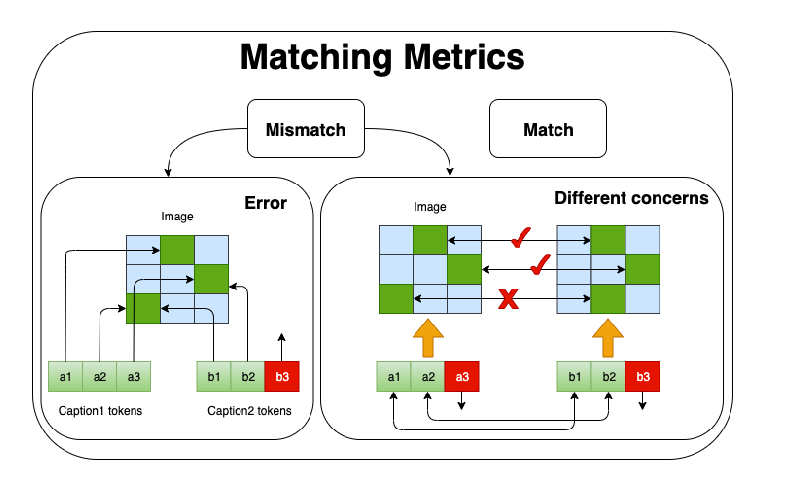
Cross-modal Coherence Modeling for Caption Generation
Malihe Alikhani, Piyush Sharma, Shengjie Li, Radu Soricut, Matthew Stone,
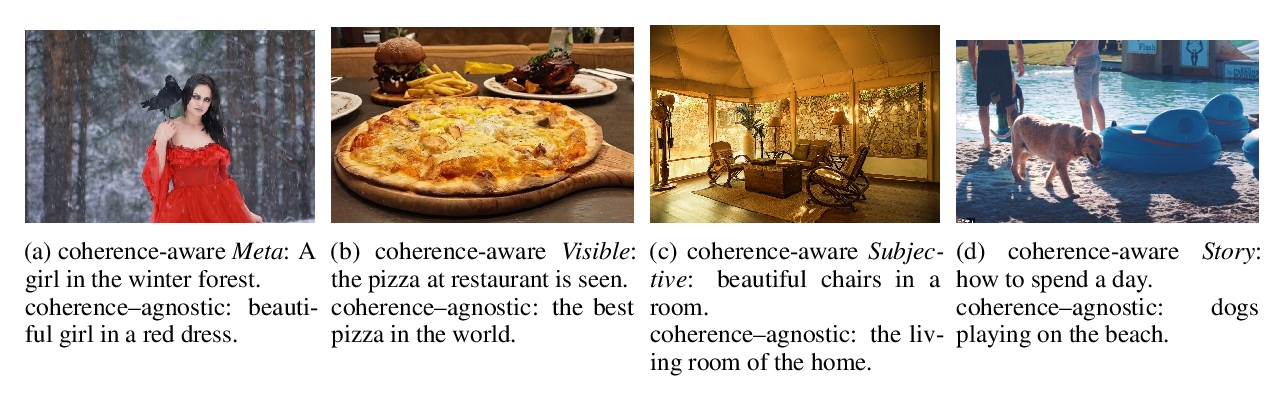
Aligned Dual Channel Graph Convolutional Network for Visual Question Answering
Qingbao Huang, Jielong Wei, Yi Cai, Changmeng Zheng, Junying Chen, Ho-fung Leung, Qing Li,
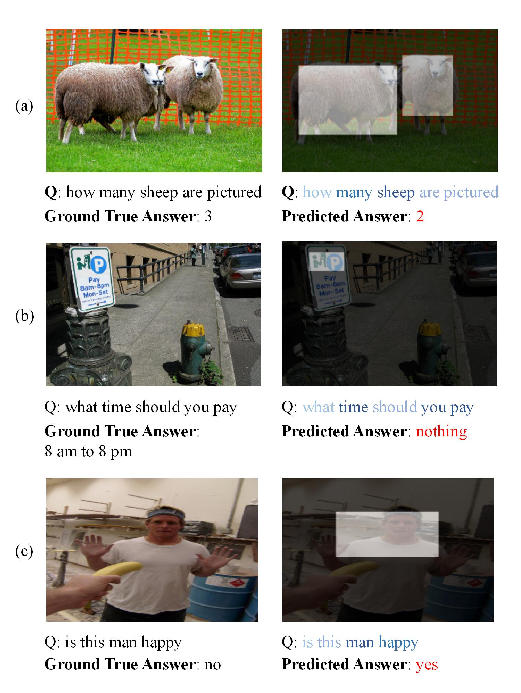