Feature Difference Makes Sense: A medical image captioning model exploiting feature difference and tag information
Hyeryun Park, Kyungmo Kim, Jooyoung Yoon, Seongkeun Park, Jinwook Choi
Student Research Workshop SRW Paper
Session 2B: Jul 6
(09:00-10:00 GMT)
Session 11B: Jul 8
(06:00-07:00 GMT)
Abstract:
Medical image captioning can reduce the workload of physicians and save time and expense by automatically generating reports. However, current datasets are small and limited, creating additional challenges for researchers. In this study, we propose a feature difference and tag information combined long short-term memory (LSTM) model for chest x-ray report generation. A feature vector extracted from the image conveys visual information, but its ability to describe the image is limited. Other image captioning studies exhibited improved performance by exploiting feature differences, so the proposed model also utilizes them. First, we propose a difference and tag (DiTag) model containing the difference between the patient and normal images. Then, we propose a multi-difference and tag (mDiTag) model that also contains information about low-level differences, such as contrast, texture, and localized area. Evaluation of the proposed models demonstrates that the mDiTag model provides more information to generate captions and outperforms all other models.
You can open the
pre-recorded video
in a separate window.
NOTE: The SlidesLive video may display a random order of the authors.
The correct author list is shown at the top of this webpage.
Similar Papers
Cross-modal Coherence Modeling for Caption Generation
Malihe Alikhani, Piyush Sharma, Shengjie Li, Radu Soricut, Matthew Stone,
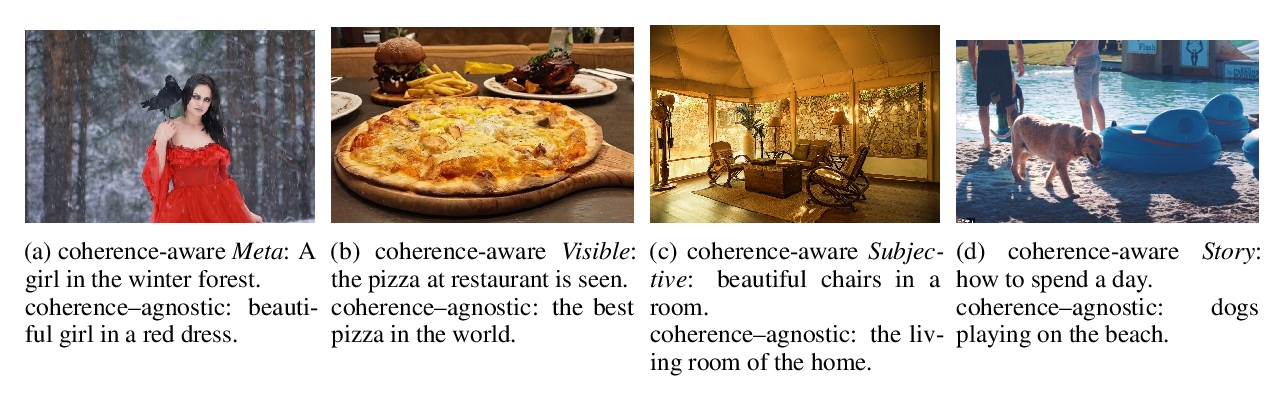
Aligned Dual Channel Graph Convolutional Network for Visual Question Answering
Qingbao Huang, Jielong Wei, Yi Cai, Changmeng Zheng, Junying Chen, Ho-fung Leung, Qing Li,
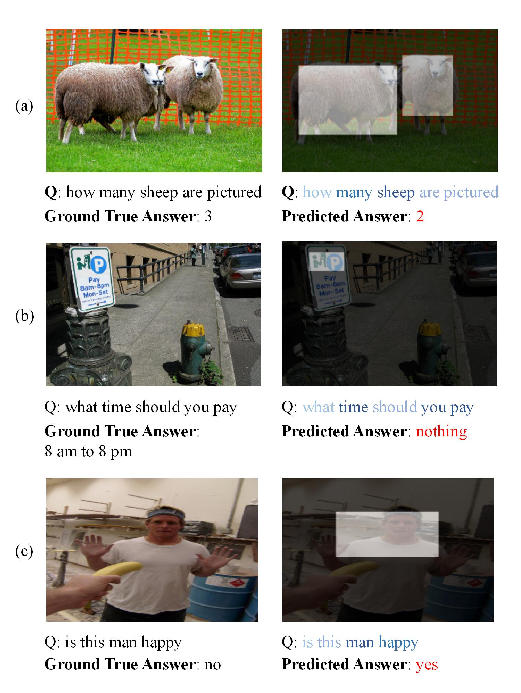
Dense-Caption Matching and Frame-Selection Gating for Temporal Localization in VideoQA
Hyounghun Kim, Zineng Tang, Mohit Bansal,
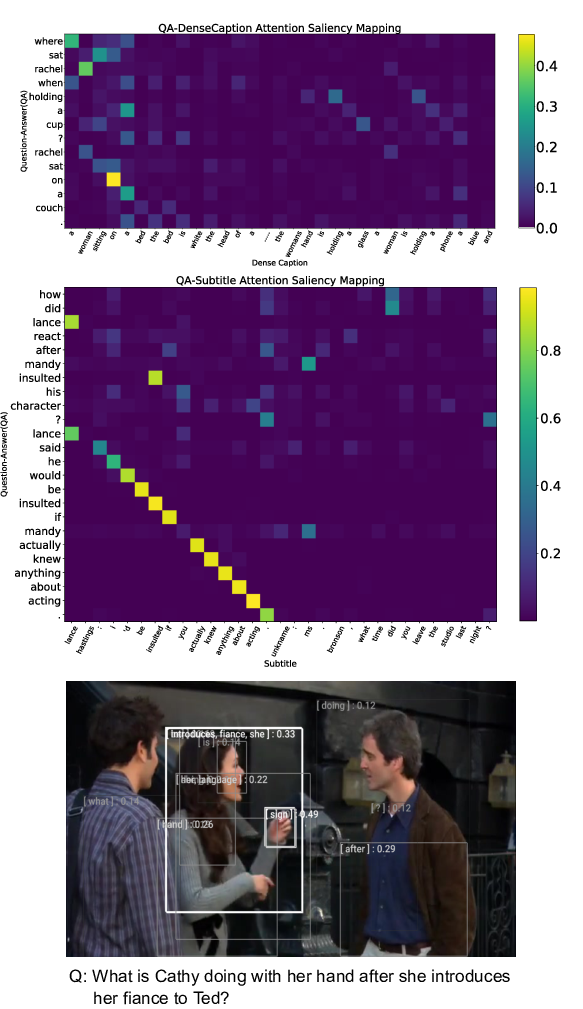