Tagged Back-translation Revisited: Why Does It Really Work?
Benjamin Marie, Raphael Rubino, Atsushi Fujita
Machine Translation Short Paper
Session 11A: Jul 8
(05:00-06:00 GMT)
Session 15A: Jul 8
(20:00-21:00 GMT)
Abstract:
In this paper, we show that neural machine translation (NMT) systems trained on large back-translated data overfit some of the characteristics of machine-translated texts. Such NMT systems better translate human-produced translations, i.e., translationese, but may largely worsen the translation quality of original texts. Our analysis reveals that adding a simple tag to back-translations prevents this quality degradation and improves on average the overall translation quality by helping the NMT system to distinguish back-translated data from original parallel data during training. We also show that, in contrast to high-resource configurations, NMT systems trained in low-resource settings are much less vulnerable to overfit back-translations. We conclude that the back-translations in the training data should always be tagged especially when the origin of the text to be translated is unknown.
You can open the
pre-recorded video
in a separate window.
NOTE: The SlidesLive video may display a random order of the authors.
The correct author list is shown at the top of this webpage.
Similar Papers
On The Evaluation of Machine Translation SystemsTrained With Back-Translation
Sergey Edunov, Myle Ott, Marc'Aurelio Ranzato, Michael Auli,
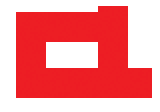
Leveraging Monolingual Data with Self-Supervision for Multilingual Neural Machine Translation
Aditya Siddhant, Ankur Bapna, Yuan Cao, Orhan Firat, Mia Chen, Sneha Kudugunta, Naveen Arivazhagan, Yonghui Wu,
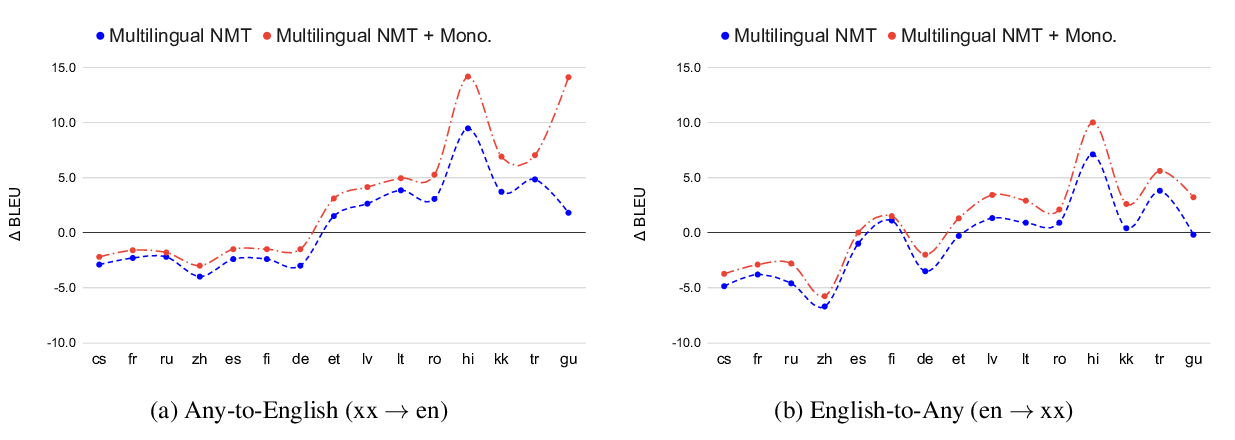
Translationese as a Language in "Multilingual" NMT
Parker Riley, Isaac Caswell, Markus Freitag, David Grangier,
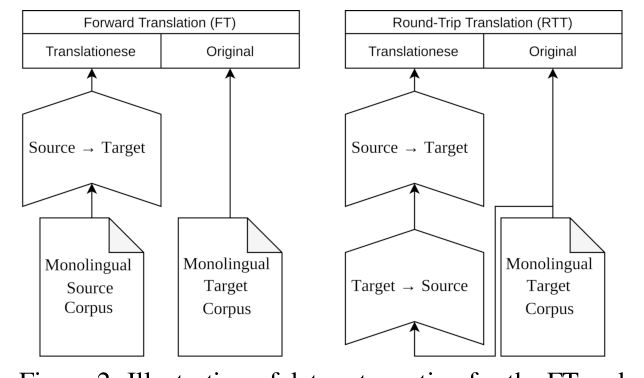
Multi-Task Neural Model for Agglutinative Language Translation
Yirong Pan, Xiao Li, Yating Yang, Rui Dong,
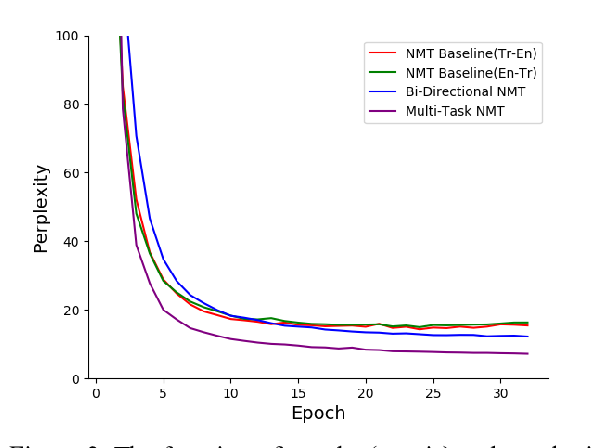