uBLEU: Uncertainty-Aware Automatic Evaluation Method for Open-Domain Dialogue Systems
Tsuta Yuma, Naoki Yoshinaga, Masashi Toyoda
Student Research Workshop SRW Paper
Session 6A: Jul 7
(05:00-06:00 GMT)
Session 12A: Jul 8
(08:00-09:00 GMT)
Abstract:
Because open-domain dialogues allow diverse responses, basic reference-based metrics such as BLEU do not work well unless we prepare a massive reference set of high-quality responses for input utterances. To reduce this burden, a human-aided, uncertainty-aware metric, ΔBLEU, has been proposed; it embeds human judgment on the quality of reference outputs into the computation of multiple-reference BLEU. In this study, we instead propose a fully automatic, uncertainty-aware evaluation method for open-domain dialogue systems, υBLEU. This method first collects diverse reference responses from massive dialogue data and then annotates their quality judgments by using a neural network trained on automatically collected training data. Experimental results on massive Twitter data confirmed that υBLEU is comparable to ΔBLEU in terms of its correlation with human judgment and that the state of the art automatic evaluation method, RUBER, is improved by integrating υBLEU.
You can open the
pre-recorded video
in a separate window.
NOTE: The SlidesLive video may display a random order of the authors.
The correct author list is shown at the top of this webpage.
Similar Papers
Learning an Unreferenced Metric for Online Dialogue Evaluation
Koustuv Sinha, Prasanna Parthasarathi, Jasmine Wang, Ryan Lowe, William L. Hamilton, Joelle Pineau,
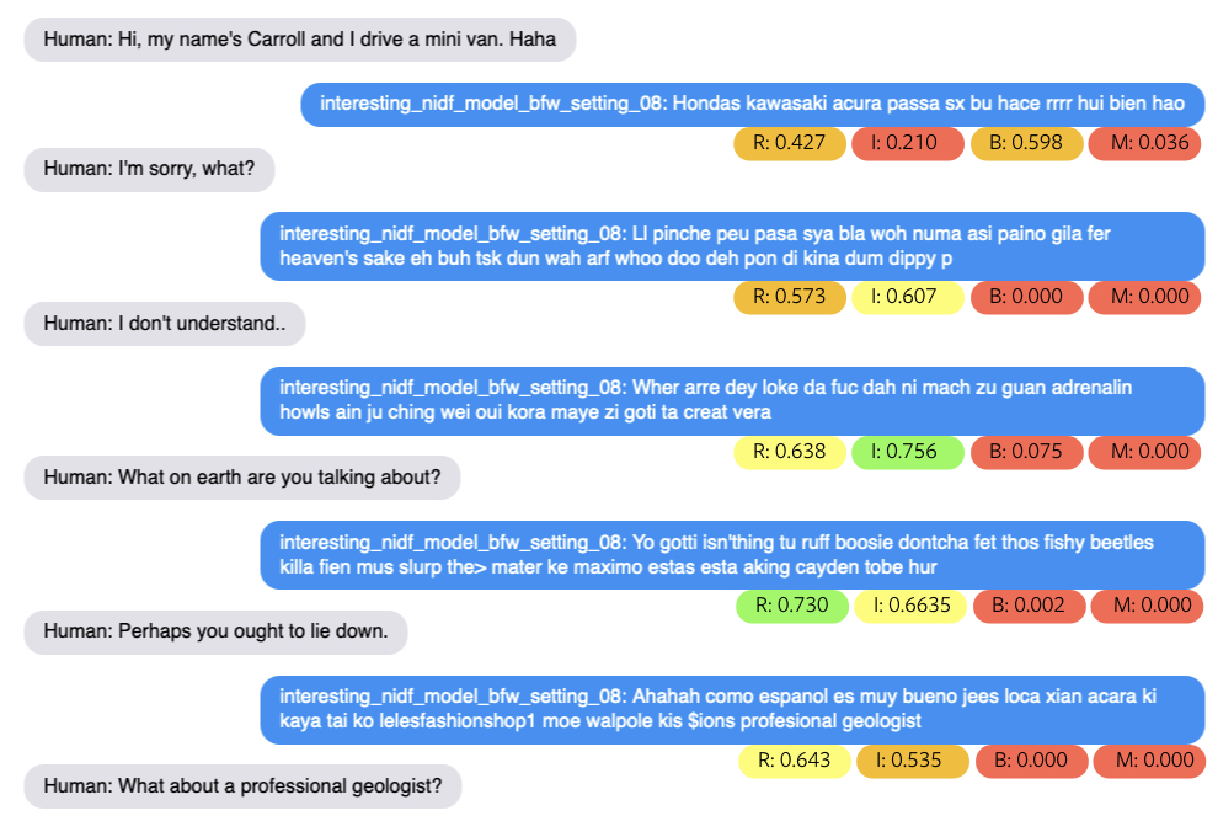
Evaluating Dialogue Generation Systems via Response Selection
Shiki Sato, Reina Akama, Hiroki Ouchi, Jun Suzuki, Kentaro Inui,
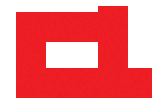
Designing Precise and Robust Dialogue Response Evaluators
Tianyu Zhao, Divesh Lala, Tatsuya Kawahara,
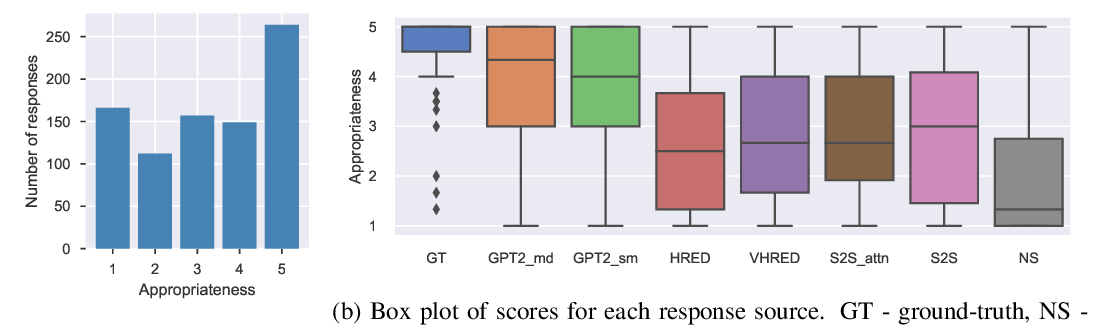