Integrating Multimodal Information in Large Pretrained Transformers
Wasifur Rahman, Md Kamrul Hasan, Sangwu Lee, AmirAli Bagher Zadeh, Chengfeng Mao, Louis-Philippe Morency, Ehsan Hoque
Speech and Multimodality Long Paper
Session 4A: Jul 6
(17:00-18:00 GMT)
Session 5A: Jul 6
(20:00-21:00 GMT)
Abstract:
Recent Transformer-based contextual word representations, including BERT and XLNet, have shown state-of-the-art performance in multiple disciplines within NLP. Fine-tuning the trained contextual models on task-specific datasets has been the key to achieving superior performance downstream. While fine-tuning these pre-trained models is straightforward for lexical applications (applications with only language modality), it is not trivial for multimodal language (a growing area in NLP focused on modeling face-to-face communication). More specifically, this is due to the fact that pre-trained models don't have the necessary components to accept two extra modalities of vision and acoustic. In this paper, we proposed an attachment to BERT and XLNet called Multimodal Adaptation Gate (MAG). MAG allows BERT and XLNet to accept multimodal nonverbal data during fine-tuning. It does so by generating a shift to internal representation of BERT and XLNet; a shift that is conditioned on the visual and acoustic modalities. In our experiments, we study the commonly used CMU-MOSI and CMU-MOSEI datasets for multimodal sentiment analysis. Fine-tuning MAG-BERT and MAG-XLNet significantly boosts the sentiment analysis performance over previous baselines as well as language-only fine-tuning of BERT and XLNet. On the CMU-MOSI dataset, MAG-XLNet achieves human-level multimodal sentiment analysis performance for the first time in the NLP community.
You can open the
pre-recorded video
in a separate window.
NOTE: The SlidesLive video may display a random order of the authors.
The correct author list is shown at the top of this webpage.
Similar Papers
Transferring Monolingual Model to Low-Resource Language: The Case of Tigrinya
Abrhalei Frezghi Tela, Abraham Woubie Zewoudie, Ville Hautamäki,
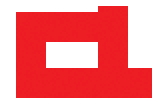
SMART: Robust and Efficient Fine-Tuning for Pre-trained Natural Language Models through Principled Regularized Optimization
Haoming Jiang, Pengcheng He, Weizhu Chen, Xiaodong Liu, Jianfeng Gao, Tuo Zhao,
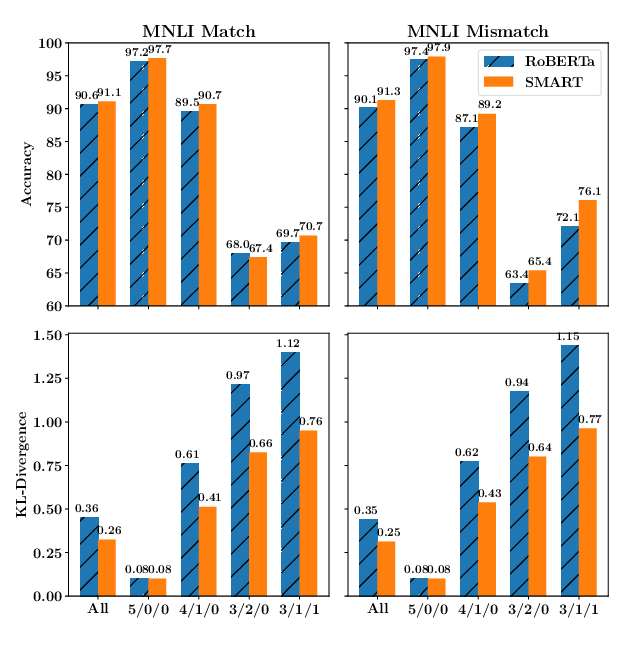
Do you have the right scissors? Tailoring Pre-trained Language Models via Monte-Carlo Methods
Ning Miao, Yuxuan Song, Hao Zhou, Lei Li,
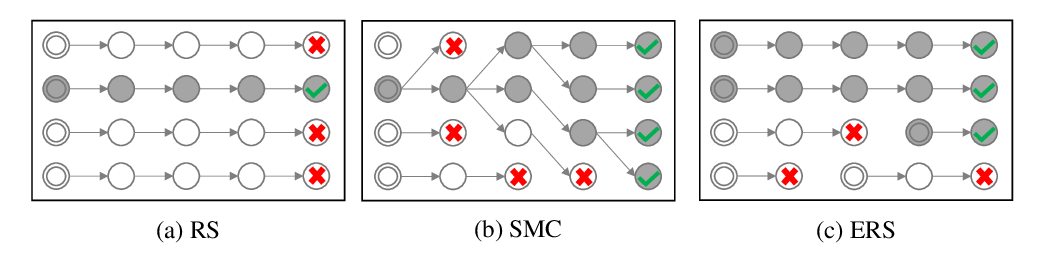
Pre-training Is (Almost) All You Need: An Application to Commonsense Reasoning
Alexandre Tamborrino, Nicola Pellicanò, Baptiste Pannier, Pascal Voitot, Louise Naudin,
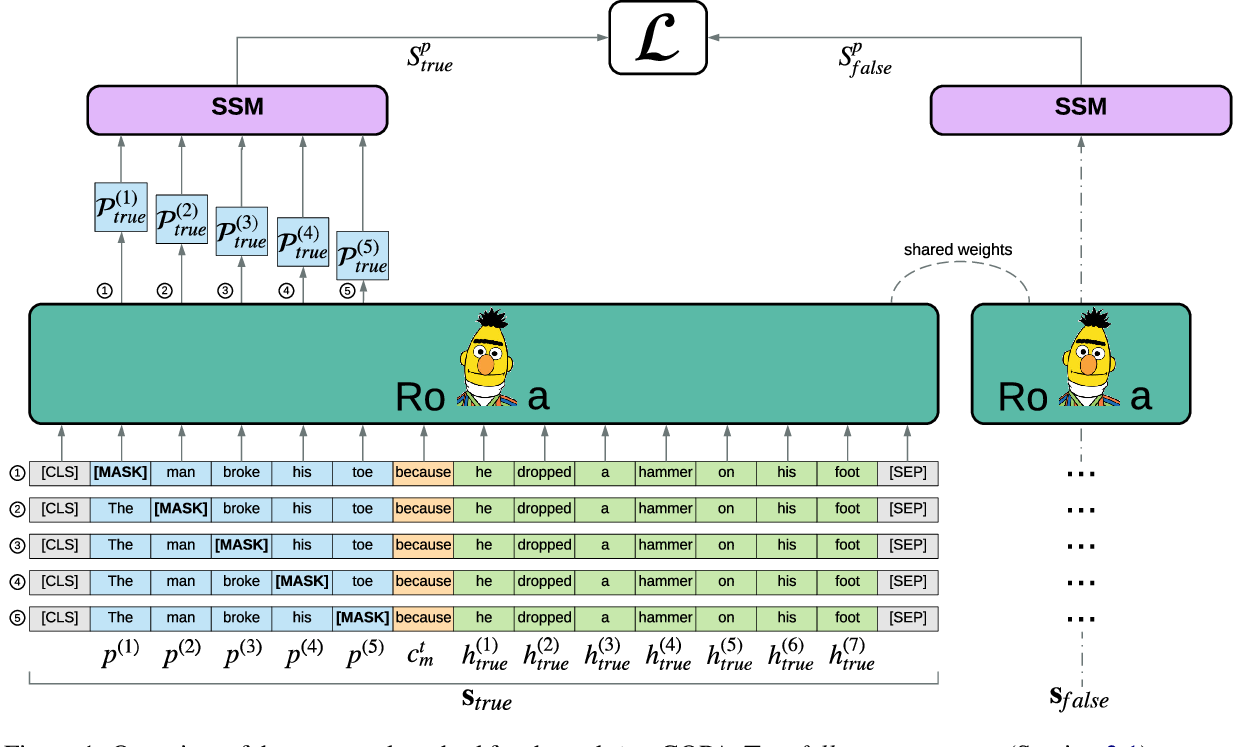