AraDIC: Arabic Document Classification Using Image-Based Character Embeddings and Class-Balanced Loss
Mahmoud Daif, Shunsuke Kitada, Hitoshi Iyatomi
Student Research Workshop SRW Paper
Session 6A: Jul 7
(05:00-06:00 GMT)
Session 13B: Jul 8
(13:00-14:00 GMT)
Abstract:
Classical and some deep learning techniques for Arabic text classification often depend on complex morphological analysis, word segmentation, and hand-crafted feature engineering.These could be eliminated by using character-level features.We propose a novel end-to-end Arabic document classification framework, Arabic document image-based classifier (AraDIC), inspired by the work on image-based character embeddings.AraDIC consists of an image-based character encoder and a classifier.They are trained in an end-to-end fashion using the class balanced loss to deal with the long-tailed data distribution problem.To evaluate the effectiveness of AraDIC, we created and published two datasets, the Arabic Wikipedia title (AWT) dataset and the Arabic poetry (AraP) dataset.To the best of our knowledge, this is the first image-based character embedding framework addressing the problem of Arabic text classification. We also present the first deep learning-based text classifier widely evaluated on modern standard Arabic, colloquial Arabic, and Classical Arabic.AraDIC shows performance improvement over classical and deep learning baselines by 12.29% and 23.05% for the micro and macro F-score, respectively.
You can open the
pre-recorded video
in a separate window.
NOTE: The SlidesLive video may display a random order of the authors.
The correct author list is shown at the top of this webpage.
Similar Papers
Joint Diacritization, Lemmatization, Normalization, and Fine-Grained Morphological Tagging
Nasser Zalmout, Nizar Habash,
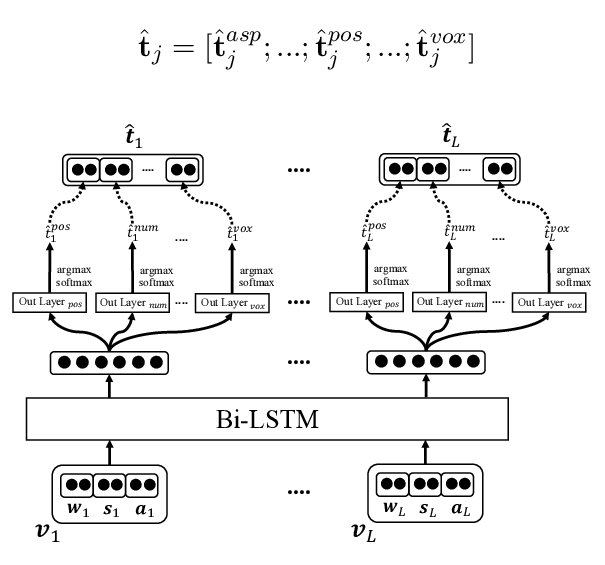
Cross-modal Coherence Modeling for Caption Generation
Malihe Alikhani, Piyush Sharma, Shengjie Li, Radu Soricut, Matthew Stone,
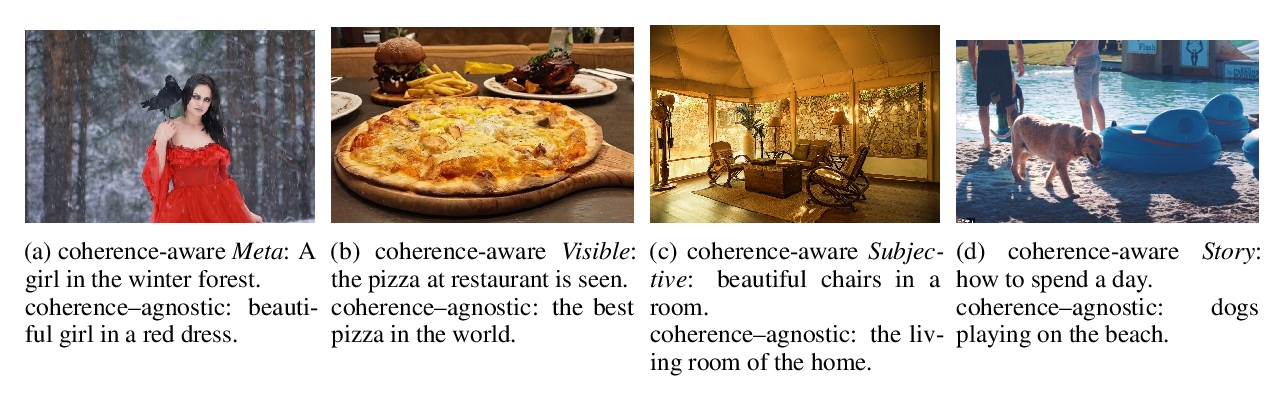
Improving Image Captioning Evaluation by Considering Inter References Variance
Yanzhi Yi, Hangyu Deng, Jinglu Hu,
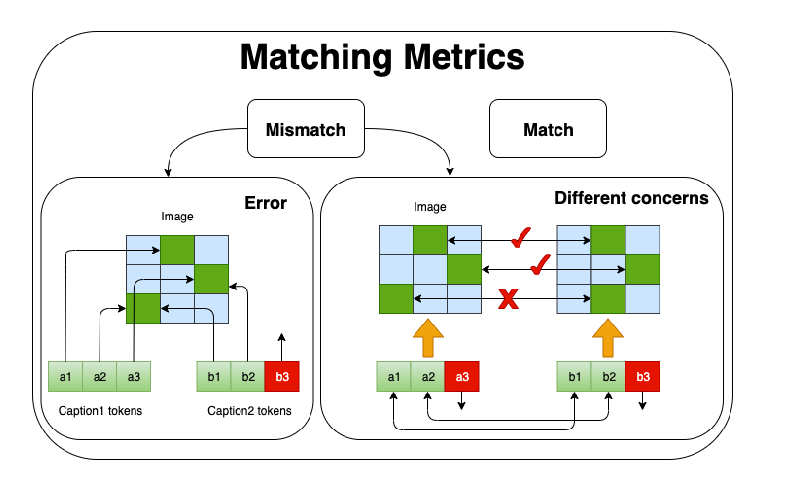
Programming in Natural Language with fuSE: Synthesizing Methods from Spoken Utterances Using Deep Natural Language Understanding
Sebastian Weigelt, Vanessa Steurer, Tobias Hey, Walter F. Tichy,
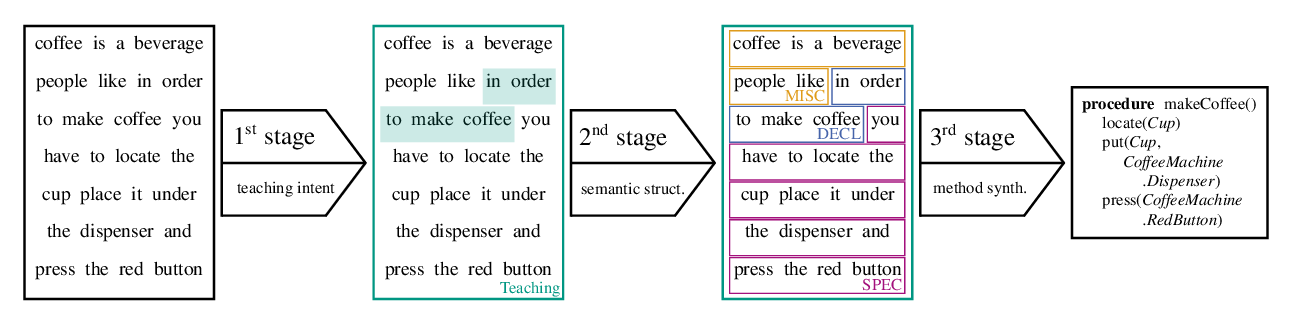