What BERT Is Not: Lessons from a New Suite of Psycholinguistic Diagnostics for Language Models
Allyson Ettinger
Interpretability and Analysis of Models for NLP TACL Paper
Session 8B: Jul 7
(13:00-14:00 GMT)
Session 10A: Jul 7
(20:00-21:00 GMT)
Abstract:
Pre-training by language modeling has become a popular and successful approach to NLP tasks, but we have yet to understand exactly what linguistic capacities these pre-training processes confer upon models. In this paper we introduce a suite of diagnostics drawn from human language experiments, which allow us to ask targeted questions about information used by language models for generating predictions in context. As a case study, we apply these diagnostics to the popular BERT model, finding that it can generally distinguish good from bad completions involving shared category or role reversal, albeit with less sensitivity than humans, and it robustly retrieves noun hypernyms, but it struggles with challenging inference and role-based event prediction — and, in particular, it shows clear insensitivity to the contextual impacts of negation.
You can open the
pre-recorded video
in a separate window.
NOTE: The SlidesLive video may display a random order of the authors.
The correct author list is shown at the top of this webpage.
Similar Papers
How does BERT's attention change when you fine-tune? An analysis methodology and a case study in negation scope
Yiyun Zhao, Steven Bethard,
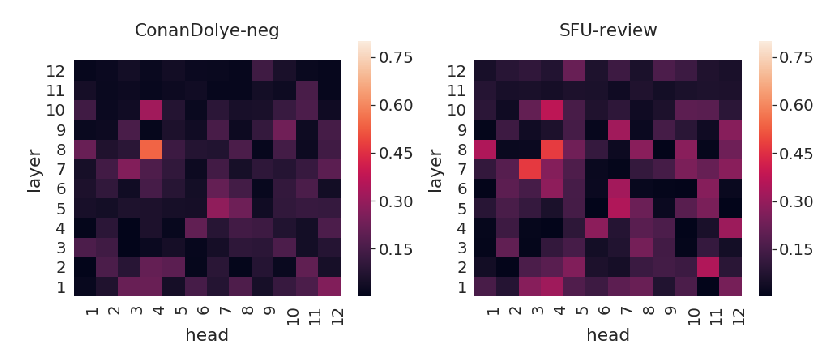
Span Selection Pre-training for Question Answering
Michael Glass, Alfio Gliozzo, Rishav Chakravarti, Anthony Ferritto, Lin Pan, G P Shrivatsa Bhargav, Dinesh Garg, Avi Sil,
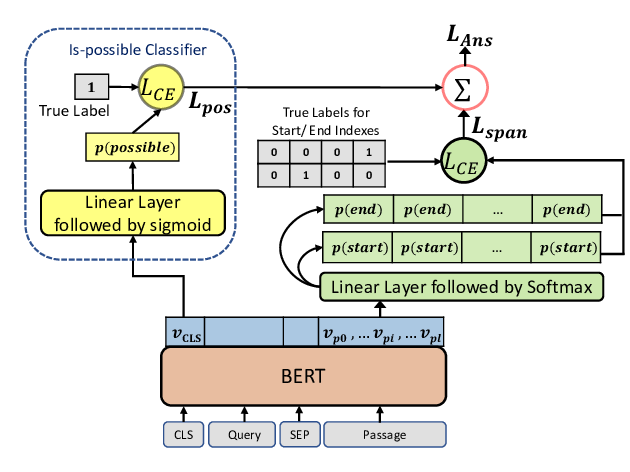
Are Natural Language Inference Models IMPPRESsive? Learning IMPlicature and PRESupposition
Paloma Jeretic, Alex Warstadt, Suvrat Bhooshan, Adina Williams,
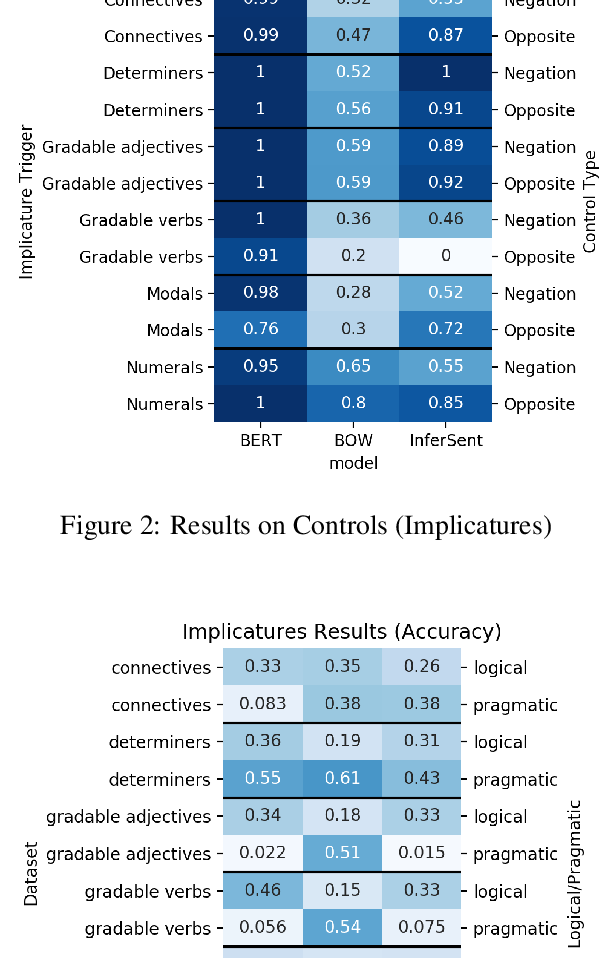
Adversarial and Domain-Aware BERT for Cross-Domain Sentiment Analysis
Chunning Du, Haifeng Sun, Jingyu Wang, Qi Qi, Jianxin Liao,
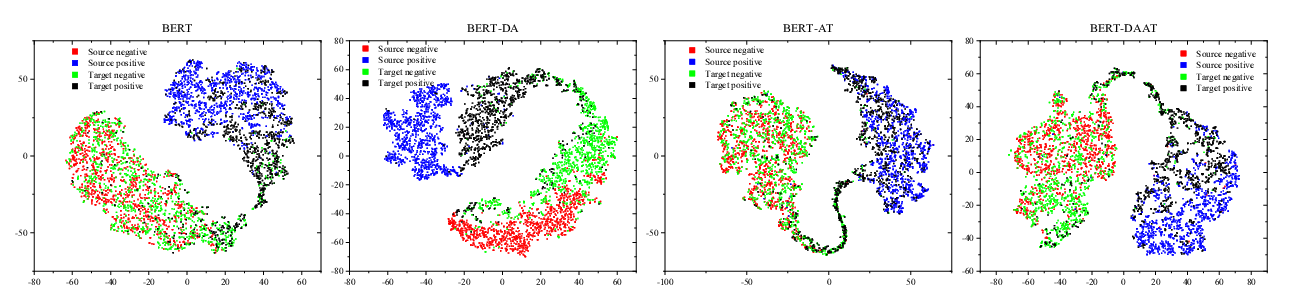