Span-ConveRT: Few-shot Span Extraction for Dialog with Pretrained Conversational Representations
Samuel Coope, Tyler Farghly, Daniela Gerz, Ivan Vulić, Matthew Henderson
Dialogue and Interactive Systems Short Paper
Session 1A: Jul 6
(05:00-06:00 GMT)
Session 3A: Jul 6
(12:00-13:00 GMT)
Abstract:
We introduce Span-ConveRT, a light-weight model for dialog slot-filling which frames the task as a turn-based span extraction task. This formulation allows for a simple integration of conversational knowledge coded in large pretrained conversational models such as ConveRT (Henderson et al., 2019). We show that leveraging such knowledge in Span-ConveRT is especially useful for few-shot learning scenarios: we report consistent gains over 1) a span extractor that trains representations from scratch in the target domain, and 2) a BERT-based span extractor. In order to inspire more work on span extraction for the slot-filling task, we also release RESTAURANTS-8K, a new challenging data set of 8,198 utterances, compiled from actual conversations in the restaurant booking domain.
You can open the
pre-recorded video
in a separate window.
NOTE: The SlidesLive video may display a random order of the authors.
The correct author list is shown at the top of this webpage.
Similar Papers
SpanBERT: Improving Pre-training by Representing and Predicting Spans
Mandar Joshi, Danqi Chen, Yinhan Liu, Daniel S. Weld, Luke Zettlemoyer, Omer Levy,
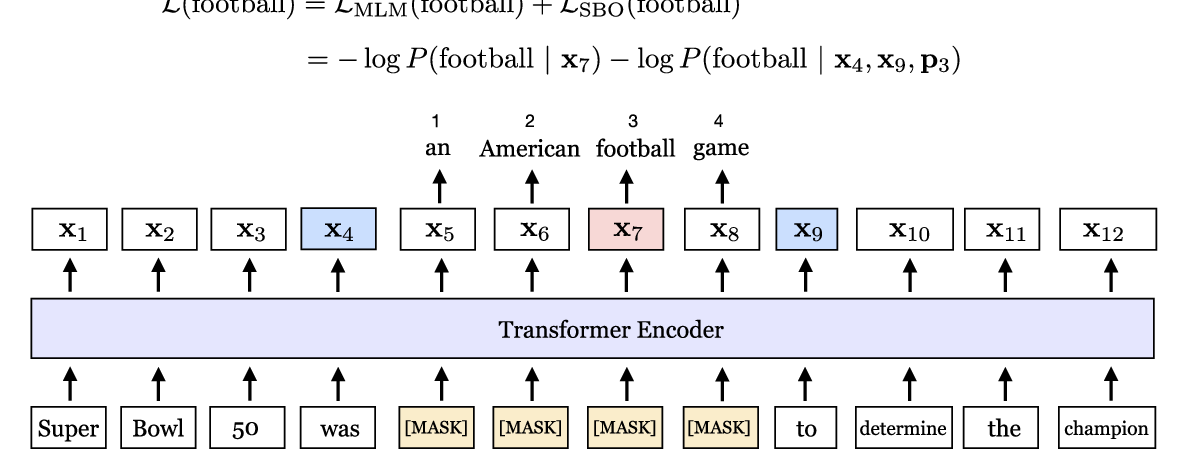
Transformers to Learn Hierarchical Contexts in Multiparty Dialogue for Span-based Question Answering
Changmao Li, Jinho D. Choi,
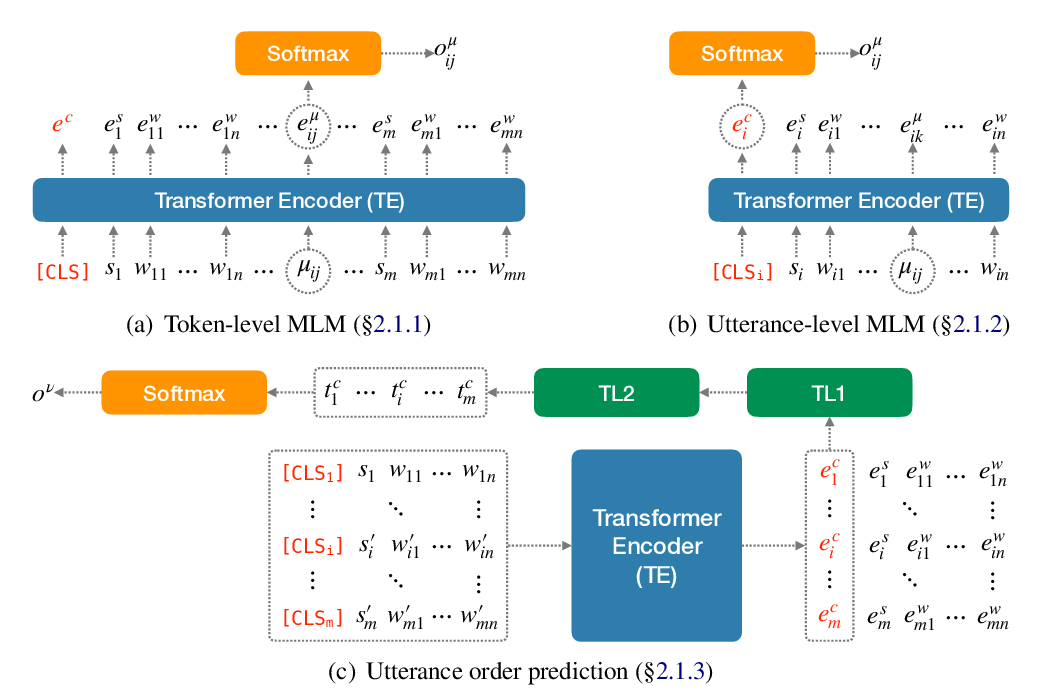
Adversarial and Domain-Aware BERT for Cross-Domain Sentiment Analysis
Chunning Du, Haifeng Sun, Jingyu Wang, Qi Qi, Jianxin Liao,
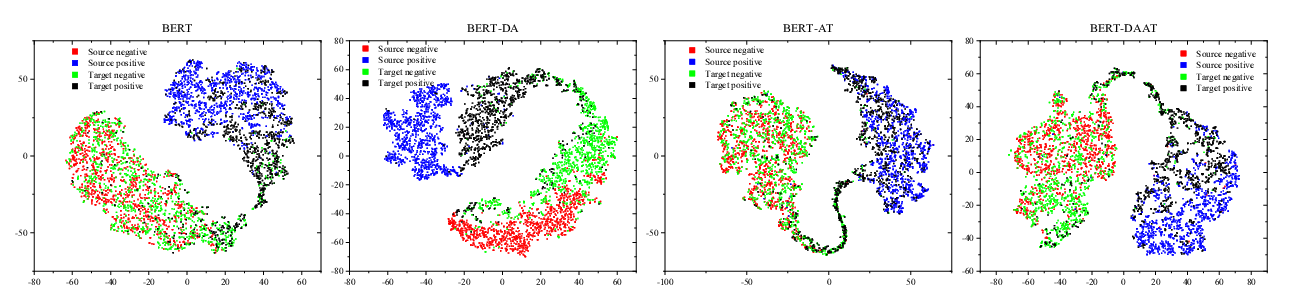
Span-based Localizing Network for Natural Language Video Localization
Hao Zhang, Aixin Sun, Wei Jing, Joey Tianyi Zhou,
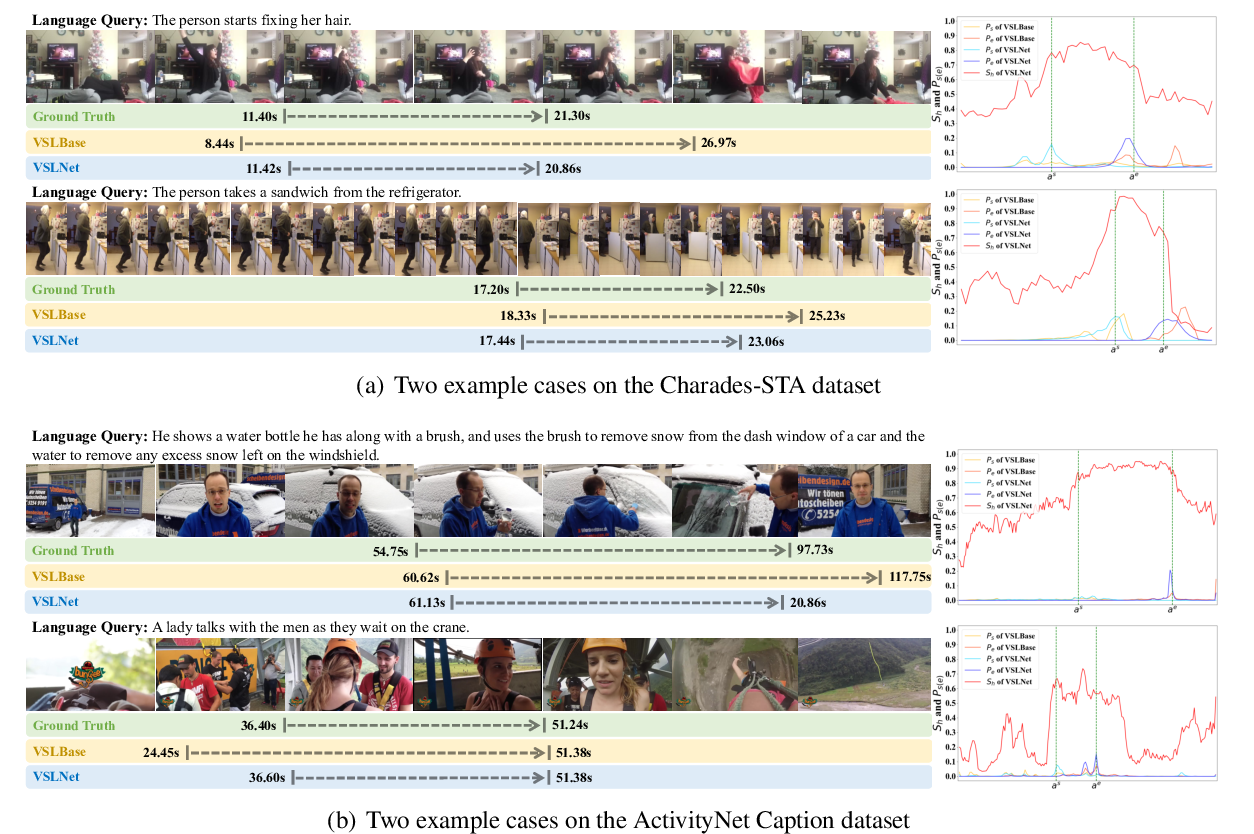