A Generative Model for Joint Natural Language Understanding and Generation
Bo-Hsiang Tseng, Jianpeng Cheng, Yimai Fang, David Vandyke
Dialogue and Interactive Systems Long Paper
Session 3A: Jul 6
(12:00-13:00 GMT)
Session 4A: Jul 6
(17:00-18:00 GMT)
Abstract:
Natural language understanding (NLU) and natural language generation (NLG) are two fundamental and related tasks in building task-oriented dialogue systems with opposite objectives: NLU tackles the transformation from natural language to formal representations, whereas NLG does the reverse. A key to success in either task is parallel training data which is expensive to obtain at a large scale. In this work, we propose a generative model which couples NLU and NLG through a shared latent variable. This approach allows us to explore both spaces of natural language and formal representations, and facilitates information sharing through the latent space to eventually benefit NLU and NLG. Our model achieves state-of-the-art performance on two dialogue datasets with both flat and tree-structured formal representations. We also show that the model can be trained in a semi-supervised fashion by utilising unlabelled data to boost its performance.
You can open the
pre-recorded video
in a separate window.
NOTE: The SlidesLive video may display a random order of the authors.
The correct author list is shown at the top of this webpage.
Similar Papers
Towards Unsupervised Language Understanding and Generation by Joint Dual Learning
Shang-Yu Su, Chao-Wei Huang, Yun-Nung Chen,
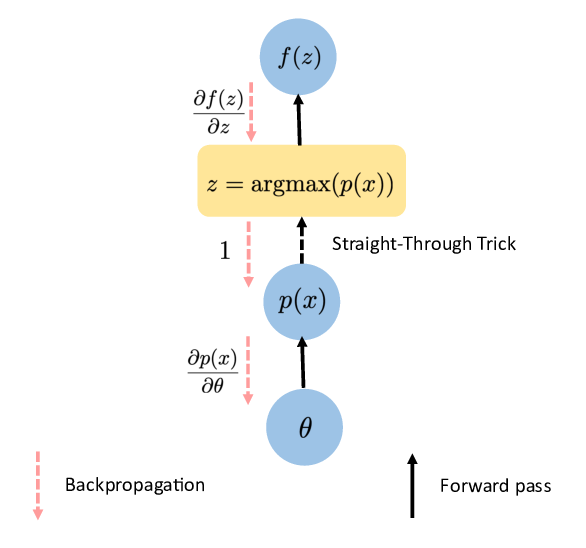
Language (Re)modelling: Towards Embodied Language Understanding
Ronen Tamari, Chen Shani, Tom Hope, Miriam R L Petruck, Omri Abend, Dafna Shahaf,
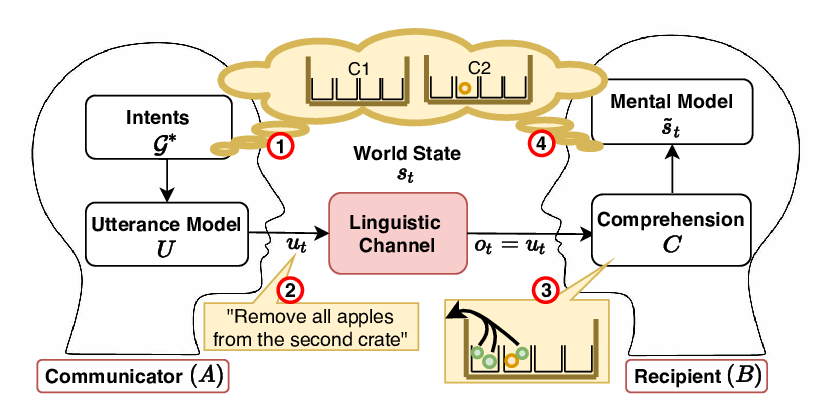
Logical Natural Language Generation from Open-Domain Tables
Wenhu Chen, Jianshu Chen, Yu Su, Zhiyu Chen, William Yang Wang,
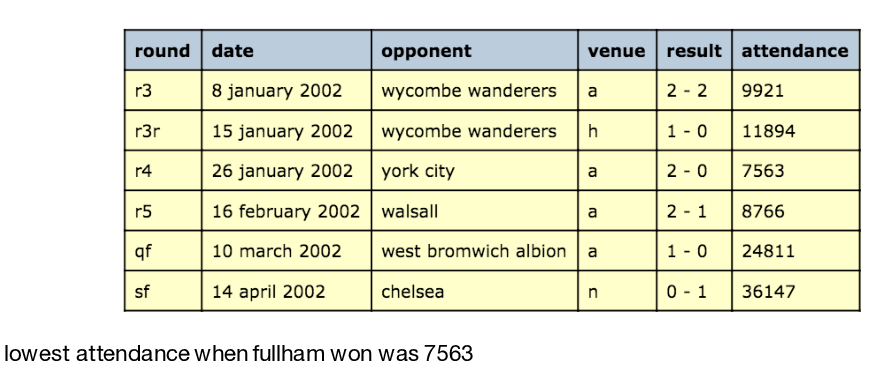
Curriculum Learning for Natural Language Understanding
Benfeng Xu, Licheng Zhang, Zhendong Mao, Quan Wang, Hongtao Xie, Yongdong Zhang,
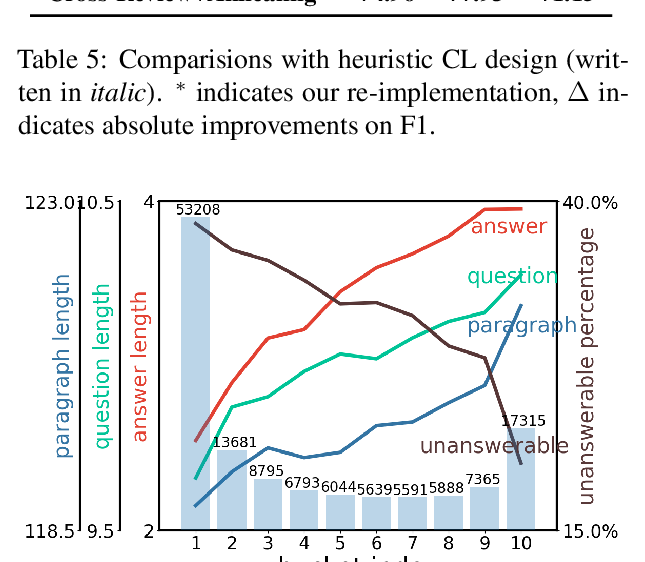