Towards Unsupervised Language Understanding and Generation by Joint Dual Learning
Shang-Yu Su, Chao-Wei Huang, Yun-Nung Chen
Dialogue and Interactive Systems Long Paper
Session 1B: Jul 6
(06:00-07:00 GMT)
Session 2A: Jul 6
(08:00-09:00 GMT)
Abstract:
In modular dialogue systems, natural language understanding (NLU) and natural language generation (NLG) are two critical components, where NLU extracts the semantics from the given texts and NLG is to construct corresponding natural language sentences based on the input semantic representations. However, the dual property between understanding and generation has been rarely explored. The prior work is the first attempt that utilized the duality between NLU and NLG to improve the performance via a dual supervised learning framework. However, the prior work still learned both components in a supervised manner; instead, this paper introduces a general learning framework to effectively exploit such duality, providing flexibility of incorporating both supervised and unsupervised learning algorithms to train language understanding and generation models in a joint fashion. The benchmark experiments demonstrate that the proposed approach is capable of boosting the performance of both NLU and NLG. The source code is available at: https://github.com/MiuLab/DuaLUG.
You can open the
pre-recorded video
in a separate window.
NOTE: The SlidesLive video may display a random order of the authors.
The correct author list is shown at the top of this webpage.
Similar Papers
A Generative Model for Joint Natural Language Understanding and Generation
Bo-Hsiang Tseng, Jianpeng Cheng, Yimai Fang, David Vandyke,
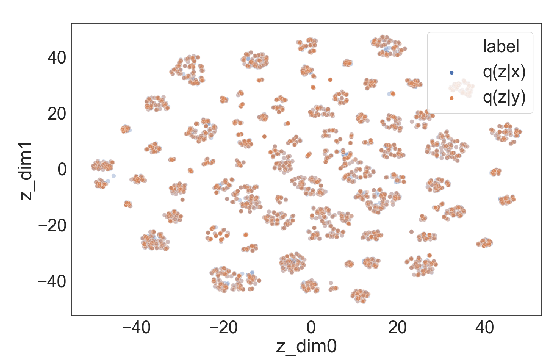
Logical Natural Language Generation from Open-Domain Tables
Wenhu Chen, Jianshu Chen, Yu Su, Zhiyu Chen, William Yang Wang,
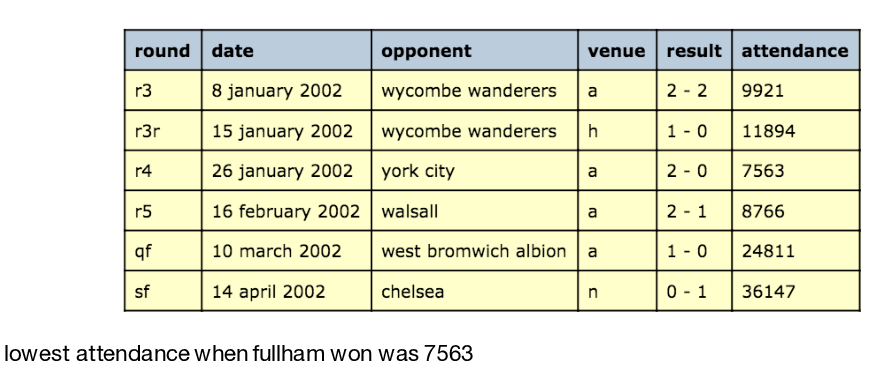
Curriculum Learning for Natural Language Understanding
Benfeng Xu, Licheng Zhang, Zhendong Mao, Quan Wang, Hongtao Xie, Yongdong Zhang,
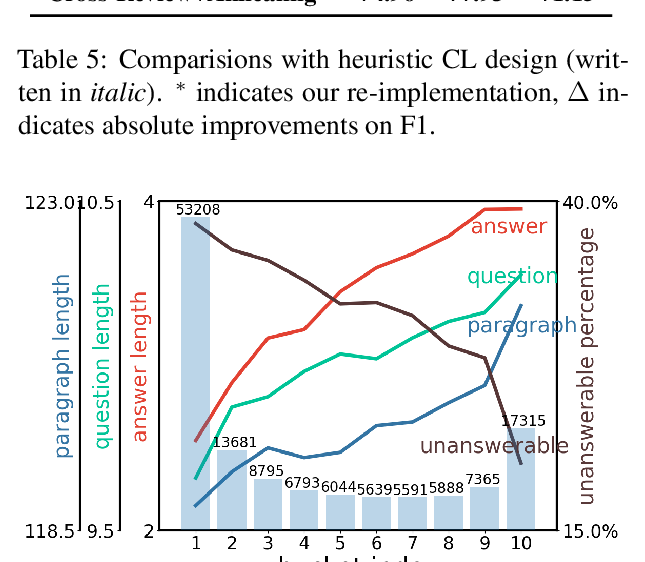