It’s Morphin’ Time! Combating Linguistic Discrimination with Inflectional Perturbations
Samson Tan, Shafiq Joty, Min-Yen Kan, Richard Socher
Ethics and NLP Long Paper
Session 6A: Jul 7
(05:00-06:00 GMT)
Session 8B: Jul 7
(13:00-14:00 GMT)
Abstract:
Training on only perfect Standard English corpora predisposes pre-trained neural networks to discriminate against minorities from non-standard linguistic backgrounds (e.g., African American Vernacular English, Colloquial Singapore English, etc.). We perturb the inflectional morphology of words to craft plausible and semantically similar adversarial examples that expose these biases in popular NLP models, e.g., BERT and Transformer, and show that adversarially fine-tuning them for a single epoch significantly improves robustness without sacrificing performance on clean data.
You can open the
pre-recorded video
in a separate window.
NOTE: The SlidesLive video may display a random order of the authors.
The correct author list is shown at the top of this webpage.
Similar Papers
Perturbed Masking: Parameter-free Probing for Analyzing and Interpreting BERT
Zhiyong Wu, Yun Chen, Ben Kao, Qun Liu,
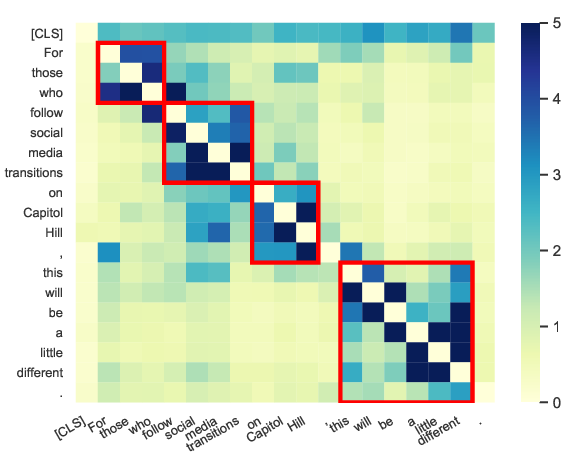
Pre-training Is (Almost) All You Need: An Application to Commonsense Reasoning
Alexandre Tamborrino, Nicola Pellicanò, Baptiste Pannier, Pascal Voitot, Louise Naudin,
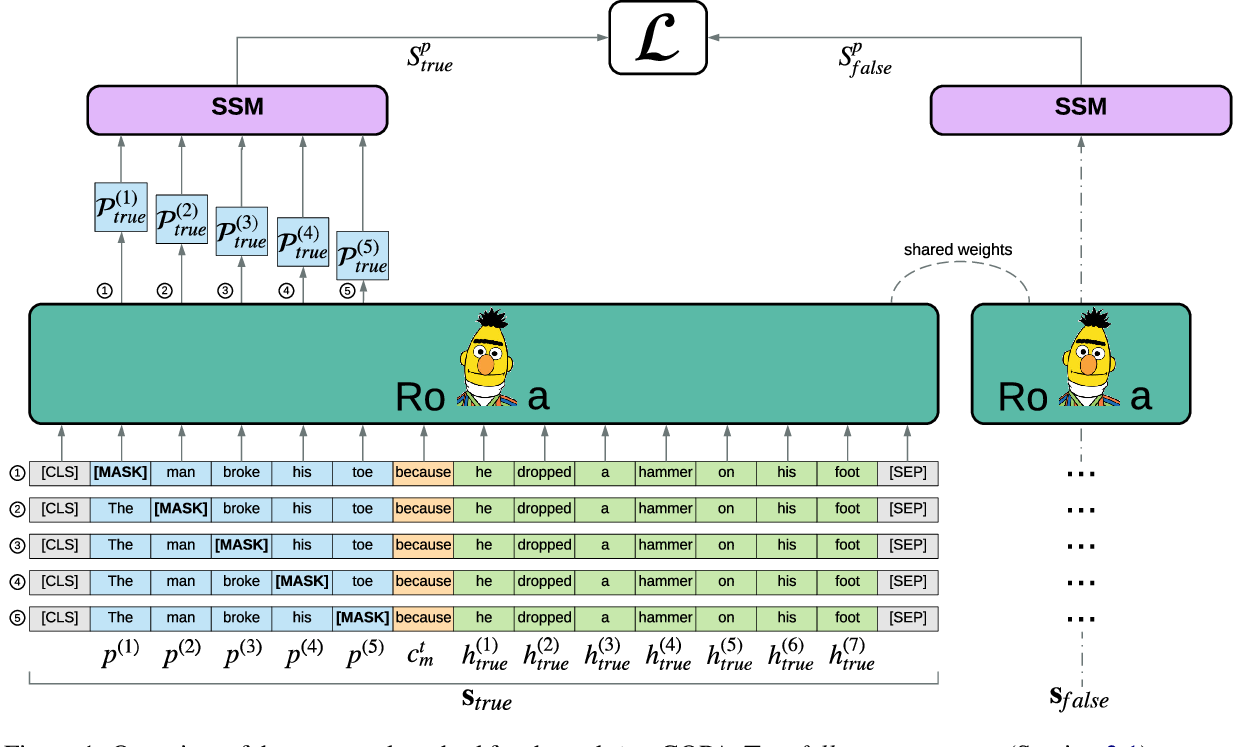
SMART: Robust and Efficient Fine-Tuning for Pre-trained Natural Language Models through Principled Regularized Optimization
Haoming Jiang, Pengcheng He, Weizhu Chen, Xiaodong Liu, Jianfeng Gao, Tuo Zhao,
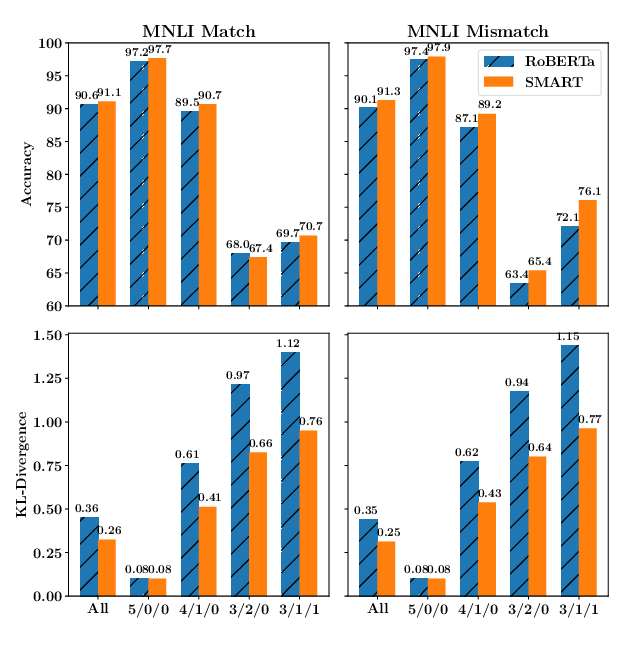
GAN-BERT: Generative Adversarial Learning for Robust Text Classification with a Bunch of Labeled Examples
Danilo Croce, Giuseppe Castellucci, Roberto Basili,
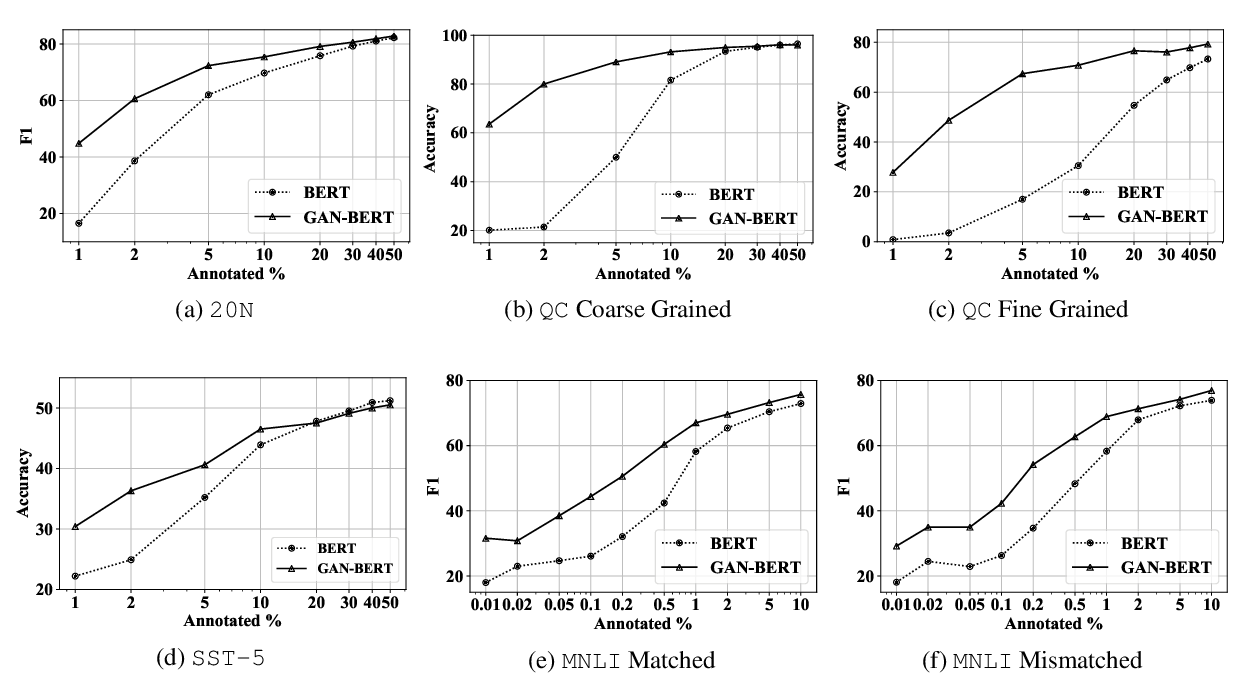