Influence Paths for Characterizing Subject-Verb Number Agreement in LSTM Language Models
Kaiji Lu, Piotr Mardziel, Klas Leino, Matt Fredrikson, Anupam Datta
Interpretability and Analysis of Models for NLP Long Paper
Session 9A: Jul 7
(17:00-18:00 GMT)
Session 10A: Jul 7
(20:00-21:00 GMT)
Abstract:
LSTM-based recurrent neural networks are the state-of-the-art for many natural language processing (NLP) tasks. Despite their performance, it is unclear whether, or how, LSTMs learn structural features of natural languages such as subject-verb number agreement in English. Lacking this understanding, the generality of LSTM performance on this task and their suitability for related tasks remains uncertain. Further, errors cannot be properly attributed to a lack of structural capability, training data omissions, or other exceptional faults. We introduce *influence paths*, a causal account of structural properties as carried by paths across gates and neurons of a recurrent neural network. The approach refines the notion of influence (the subject’s grammatical number has influence on the grammatical number of the subsequent verb) into a set of gate or neuron-level paths. The set localizes and segments the concept (e.g., subject-verb agreement), its constituent elements (e.g., the subject), and related or interfering elements (e.g., attractors). We exemplify the methodology on a widely-studied multi-layer LSTM language model, demonstrating its accounting for subject-verb number agreement. The results offer both a finer and a more complete view of an LSTM’s handling of this structural aspect of the English language than prior results based on diagnostic classifiers and ablation.
You can open the
pre-recorded video
in a separate window.
NOTE: The SlidesLive video may display a random order of the authors.
The correct author list is shown at the top of this webpage.
Similar Papers
How much complexity does an RNN architecture need to learn syntax-sensitive dependencies?
Gantavya Bhatt, Hritik Bansal, Rishubh Singh, Sumeet Agarwal,
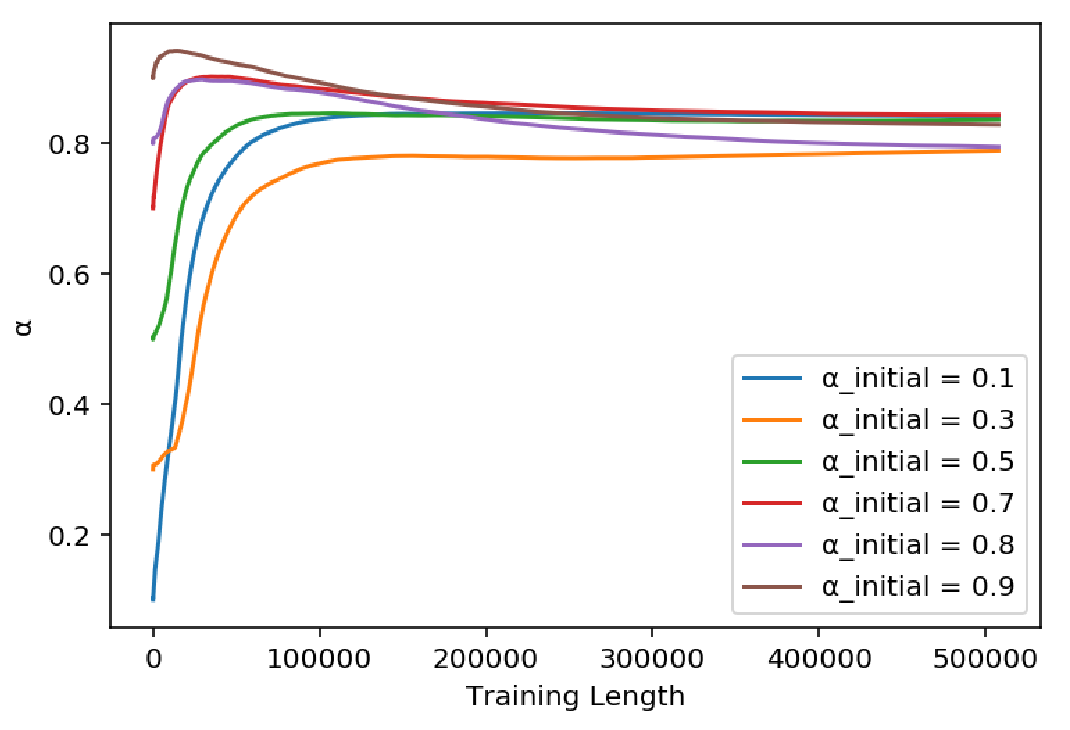
Probing for Referential Information in Language Models
Ionut-Teodor Sorodoc, Kristina Gulordava, Gemma Boleda,
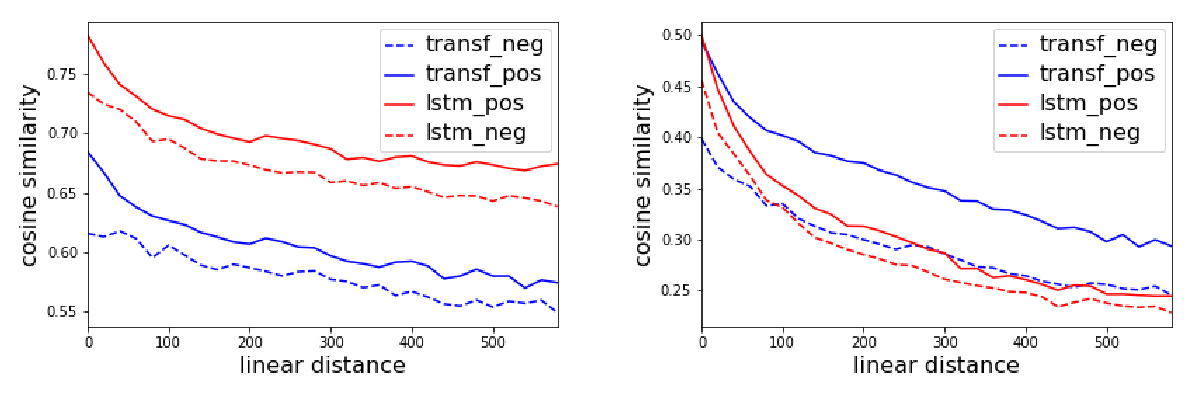
Cross-Linguistic Syntactic Evaluation of Word Prediction Models
Aaron Mueller, Garrett Nicolai, Panayiota Petrou-Zeniou, Natalia Talmina, Tal Linzen,
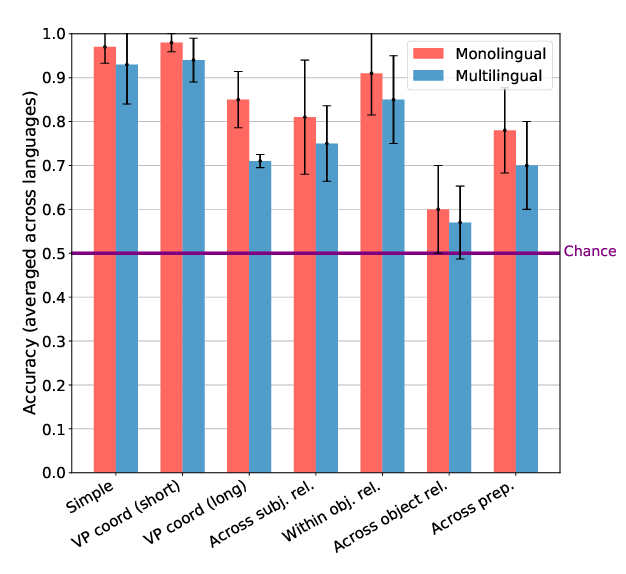
Multidirectional Associative Optimization of Function-Specific Word Representations
Daniela Gerz, Ivan Vulić, Marek Rei, Roi Reichart, Anna Korhonen,
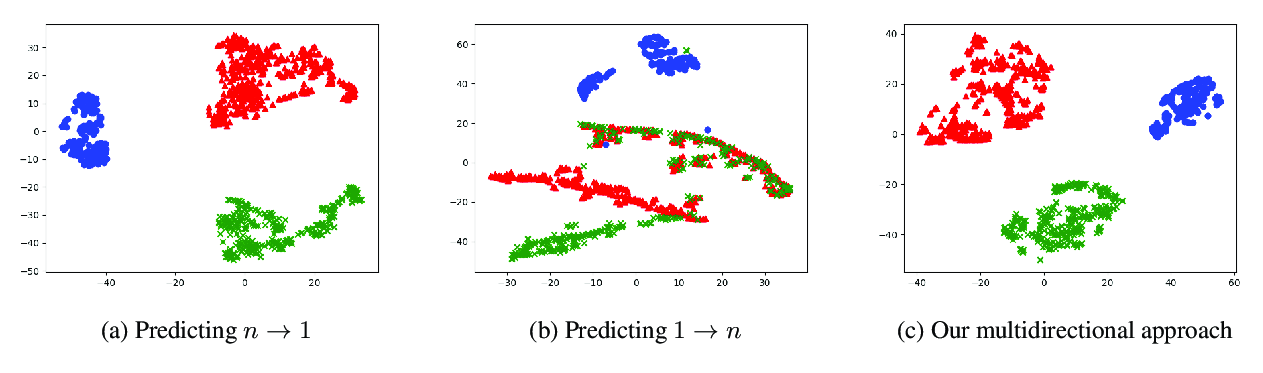