Pretraining with Contrastive Sentence Objectives Improves Discourse Performance of Language Models
Dan Iter, Kelvin Guu, Larry Lansing, Dan Jurafsky
Machine Learning for NLP Long Paper
Session 9A: Jul 7
(17:00-18:00 GMT)
Session 10B: Jul 7
(21:00-22:00 GMT)
Abstract:
Recent models for unsupervised representation learning of text have employed a number of techniques to improve contextual word representations but have put little focus on discourse-level representations. We propose Conpono, an inter-sentence objective for pretraining language models that models discourse coherence and the distance between sentences. Given an anchor sentence, our model is trained to predict the text k sentences away using a sampled-softmax objective where the candidates consist of neighboring sentences and sentences randomly sampled from the corpus. On the discourse representation benchmark DiscoEval, our model improves over the previous state-of-the-art by up to 13% and on average 4% absolute across 7 tasks. Our model is the same size as BERT-Base, but outperforms the much larger BERT-Large model and other more recent approaches that incorporate discourse. We also show that Conpono yields gains of 2%-6% absolute even for tasks that do not explicitly evaluate discourse: textual entailment (RTE), common sense reasoning (COPA) and reading comprehension (ReCoRD).
You can open the
pre-recorded video
in a separate window.
NOTE: The SlidesLive video may display a random order of the authors.
The correct author list is shown at the top of this webpage.
Similar Papers
Span Selection Pre-training for Question Answering
Michael Glass, Alfio Gliozzo, Rishav Chakravarti, Anthony Ferritto, Lin Pan, G P Shrivatsa Bhargav, Dinesh Garg, Avi Sil,
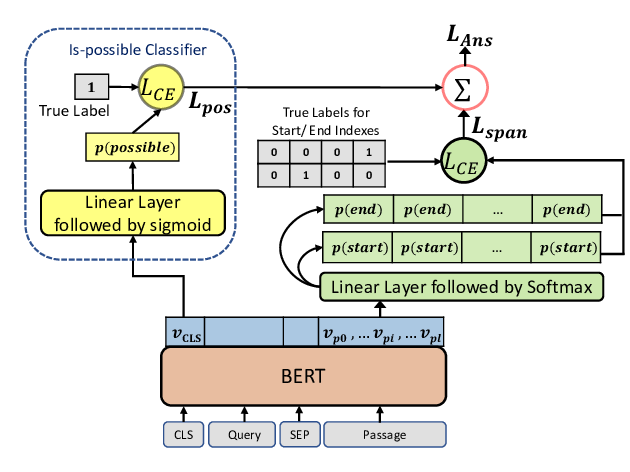
SpanBERT: Improving Pre-training by Representing and Predicting Spans
Mandar Joshi, Danqi Chen, Yinhan Liu, Daniel S. Weld, Luke Zettlemoyer, Omer Levy,
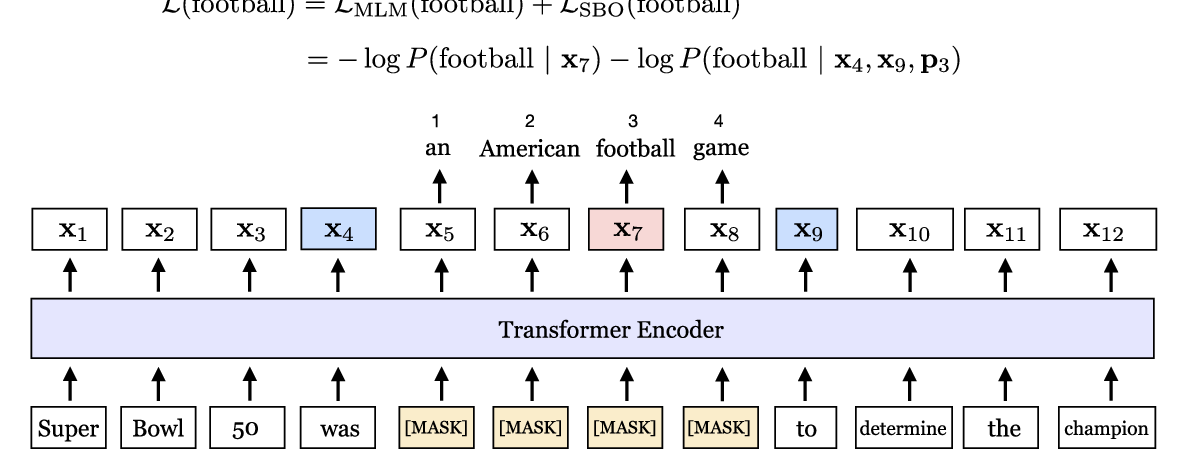