Discourse-Aware Neural Extractive Text Summarization
Jiacheng Xu, Zhe Gan, Yu Cheng, Jingjing Liu
Summarization Long Paper
Session 9A: Jul 7
(17:00-18:00 GMT)
Session 10B: Jul 7
(21:00-22:00 GMT)
Abstract:
Recently BERT has been adopted for document encoding in state-of-the-art text summarization models. However, sentence-based extractive models often result in redundant or uninformative phrases in the extracted summaries. Also, long-range dependencies throughout a document are not well captured by BERT, which is pre-trained on sentence pairs instead of documents. To address these issues, we present a discourse-aware neural summarization model - DiscoBert. DiscoBert extracts sub-sentential discourse units (instead of sentences) as candidates for extractive selection on a finer granularity. To capture the long-range dependencies among discourse units, structural discourse graphs are constructed based on RST trees and coreference mentions, encoded with Graph Convolutional Networks. Experiments show that the proposed model outperforms state-of-the-art methods by a significant margin on popular summarization benchmarks compared to other BERT-base models.
You can open the
pre-recorded video
in a separate window.
NOTE: The SlidesLive video may display a random order of the authors.
The correct author list is shown at the top of this webpage.
Similar Papers
Leveraging Graph to Improve Abstractive Multi-Document Summarization
Wei Li, Xinyan Xiao, Jiachen Liu, Hua Wu, Haifeng Wang, Junping Du,
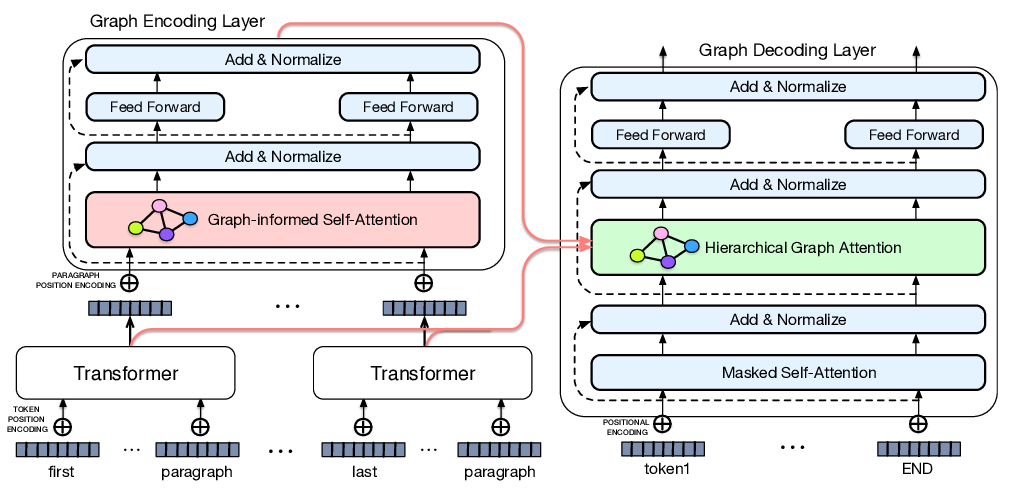
Extractive Summarization as Text Matching
Ming Zhong, Pengfei Liu, Yiran Chen, Danqing Wang, Xipeng Qiu, Xuanjing Huang,
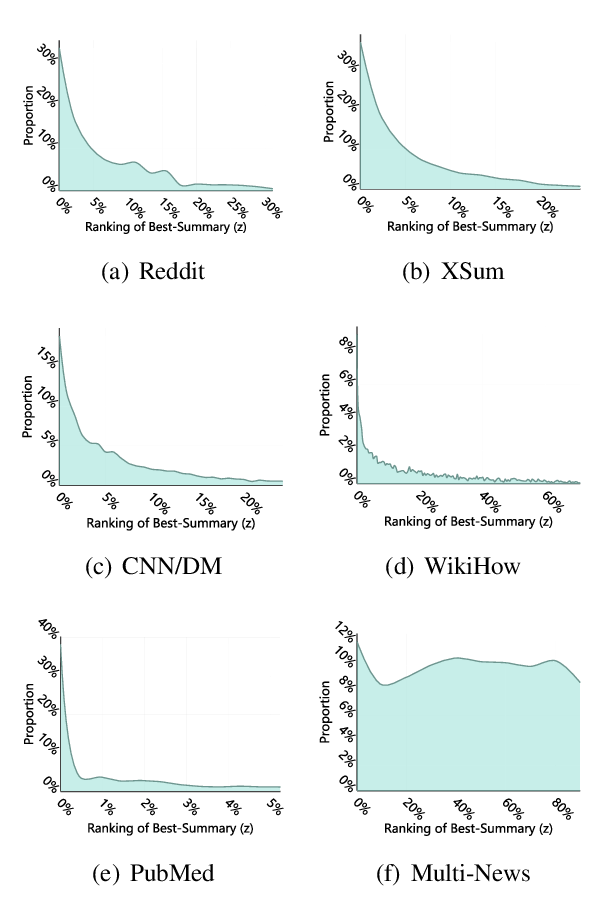
Reasoning with Latent Structure Refinement for Document-Level Relation Extraction
Guoshun Nan, Zhijiang Guo, Ivan Sekulic, Wei Lu,
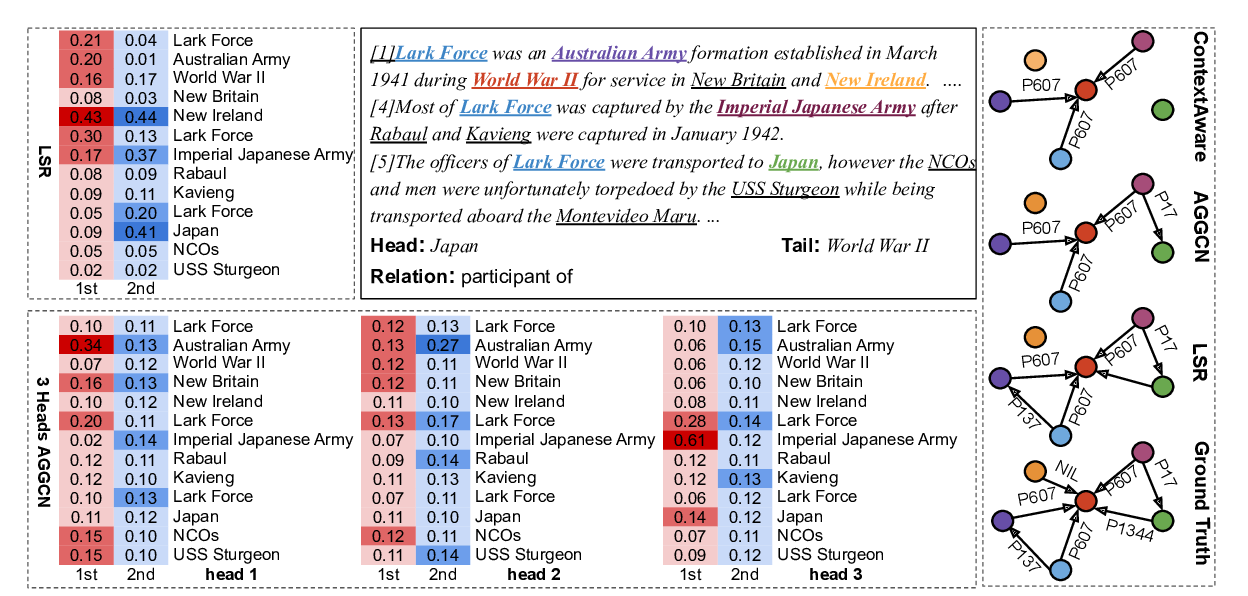
Heterogeneous Graph Neural Networks for Extractive Document Summarization
Danqing Wang, Pengfei Liu, Yining Zheng, Xipeng Qiu, Xuanjing Huang,
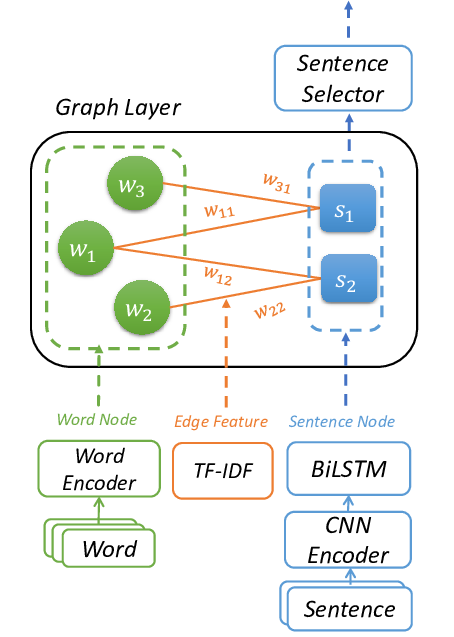