Are we Estimating or Guesstimating Translation Quality?
Shuo Sun, Francisco Guzmán, Lucia Specia
Theme Short Paper
Session 11A: Jul 8
(05:00-06:00 GMT)
Session 13B: Jul 8
(13:00-14:00 GMT)
Abstract:
Recent advances in pre-trained multilingual language models lead to state-of-the-art results on the task of quality estimation (QE) for machine translation. A carefully engineered ensemble of such models won the QE shared task at WMT19. Our in-depth analysis, however, shows that the success of using pre-trained language models for QE is over-estimated due to three issues we observed in current QE datasets: (i) The distributions of quality scores are imbalanced and skewed towards good quality scores; (iii) QE models can perform well on these datasets while looking at only source or translated sentences; (iii) They contain statistical artifacts that correlate well with human-annotated QE labels. Our findings suggest that although QE models might capture fluency of translated sentences and complexity of source sentences, they cannot model adequacy of translations effectively.
You can open the
pre-recorded video
in a separate window.
NOTE: The SlidesLive video may display a random order of the authors.
The correct author list is shown at the top of this webpage.
Similar Papers
A Simple and Effective Unified Encoder for Document-Level Machine Translation
Shuming Ma, Dongdong Zhang, Ming Zhou,
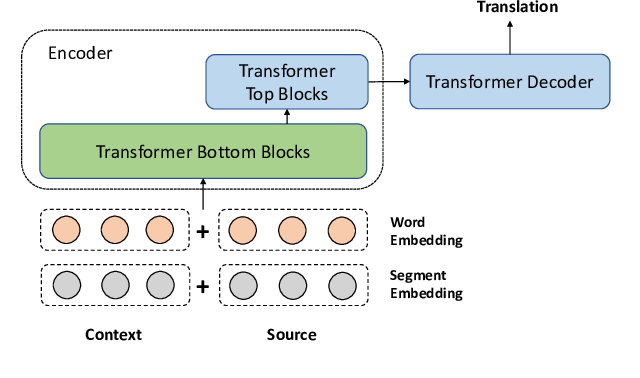
On The Evaluation of Machine Translation SystemsTrained With Back-Translation
Sergey Edunov, Myle Ott, Marc'Aurelio Ranzato, Michael Auli,
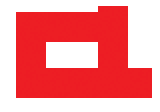