Uncertainty-Aware Curriculum Learning for Neural Machine Translation
Yikai Zhou, Baosong Yang, Derek F. Wong, Yu Wan, Lidia S. Chao
Machine Translation Long Paper
Session 12A: Jul 8
(08:00-09:00 GMT)
Session 13B: Jul 8
(13:00-14:00 GMT)
Abstract:
Neural machine translation (NMT) has proven to be facilitated by curriculum learning which presents examples in an easy-to-hard order at different training stages. The keys lie in the assessment of data difficulty and model competence. We propose uncertainty-aware curriculum learning, which is motivated by the intuition that: 1) the higher the uncertainty in a translation pair, the more complex and rarer the information it contains; and 2) the end of the decline in model uncertainty indicates the completeness of current training stage. Specifically, we serve cross-entropy of an example as its data difficulty and exploit the variance of distributions over the weights of the network to present the model uncertainty. Extensive experiments on various translation tasks reveal that our approach outperforms the strong baseline and related methods on both translation quality and convergence speed. Quantitative analyses reveal that the proposed strategy offers NMT the ability to automatically govern its learning schedule.
You can open the
pre-recorded video
in a separate window.
NOTE: The SlidesLive video may display a random order of the authors.
The correct author list is shown at the top of this webpage.
Similar Papers
Norm-Based Curriculum Learning for Neural Machine Translation
Xuebo Liu, Houtim Lai, Derek F. Wong, Lidia S. Chao,
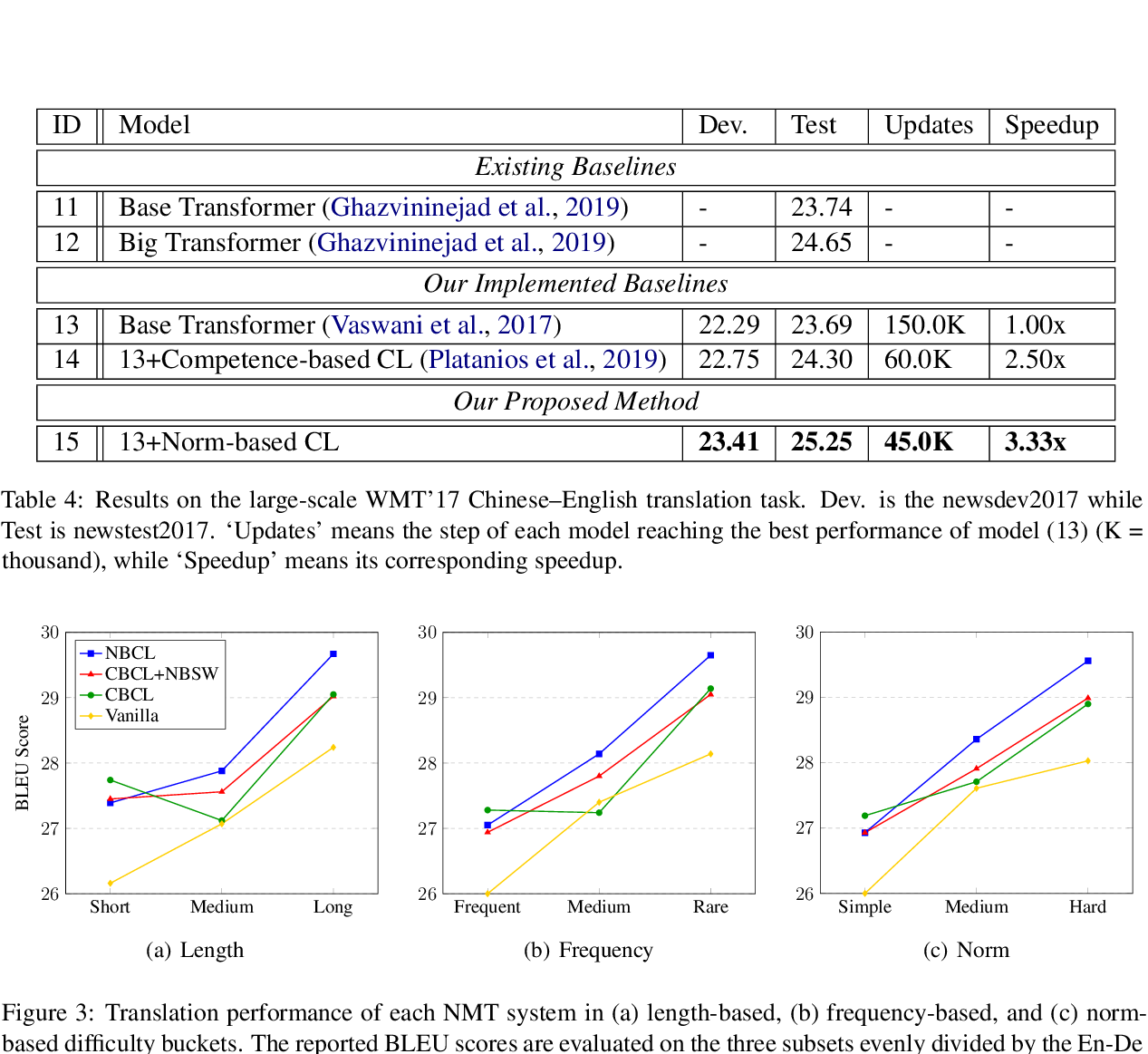
Curriculum Learning for Natural Language Understanding
Benfeng Xu, Licheng Zhang, Zhendong Mao, Quan Wang, Hongtao Xie, Yongdong Zhang,
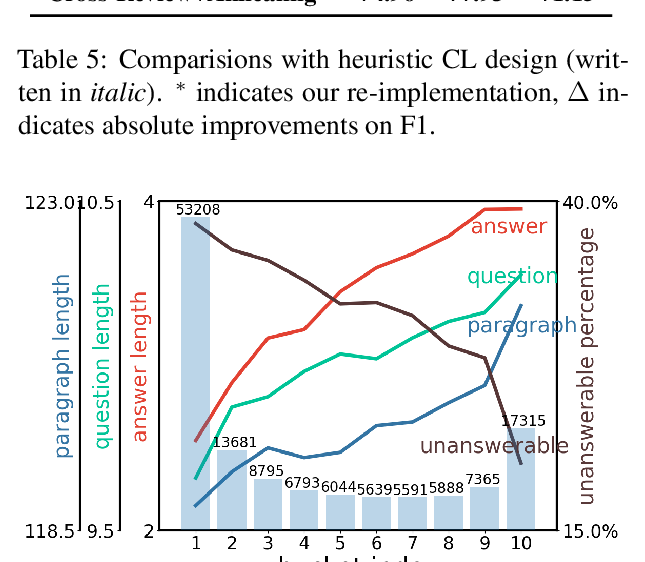
Jointly Masked Sequence-to-Sequence Model for Non-Autoregressive Neural Machine Translation
Junliang Guo, Linli Xu, Enhong Chen,
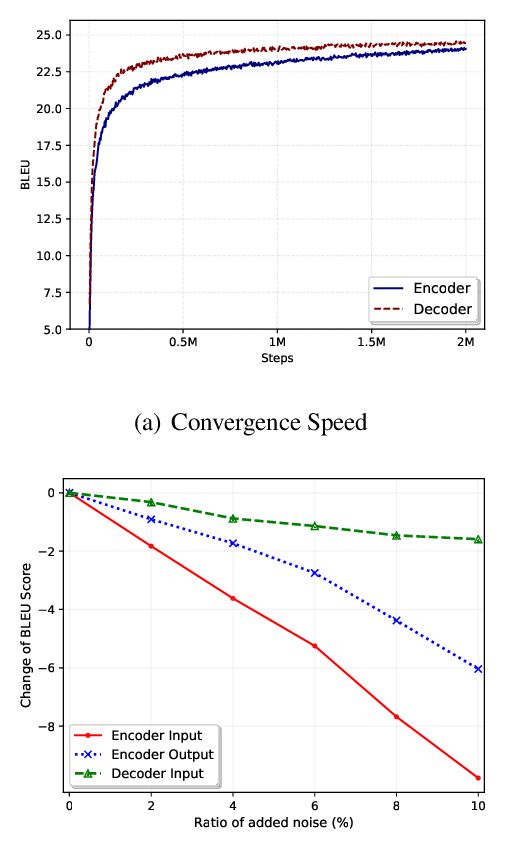