Learning a Multi-Domain Curriculum for Neural Machine Translation
Wei Wang, Ye Tian, Jiquan Ngiam, Yinfei Yang, Isaac Caswell, Zarana Parekh
Machine Translation Long Paper
Session 13B: Jul 8
(13:00-14:00 GMT)
Session 15A: Jul 8
(20:00-21:00 GMT)
Abstract:
Most data selection research in machine translation focuses on improving a single domain. We perform data selection for multiple domains at once. This is achieved by carefully introducing instance-level domain-relevance features and automatically constructing a training curriculum to gradually concentrate on multi-domain relevant and noise-reduced data batches. Both the choice of features and the use of curriculum are crucial for balancing and improving all domains, including out-of-domain. In large-scale experiments, the multi-domain curriculum simultaneously reaches or outperforms the individual performance and brings solid gains over no-curriculum training.
You can open the
pre-recorded video
in a separate window.
NOTE: The SlidesLive video may display a random order of the authors.
The correct author list is shown at the top of this webpage.
Similar Papers
Coupling Distant Annotation and Adversarial Training for Cross-Domain Chinese Word Segmentation
Ning Ding, Dingkun Long, Guangwei Xu, Muhua Zhu, Pengjun Xie, Xiaobin Wang, Haitao Zheng,
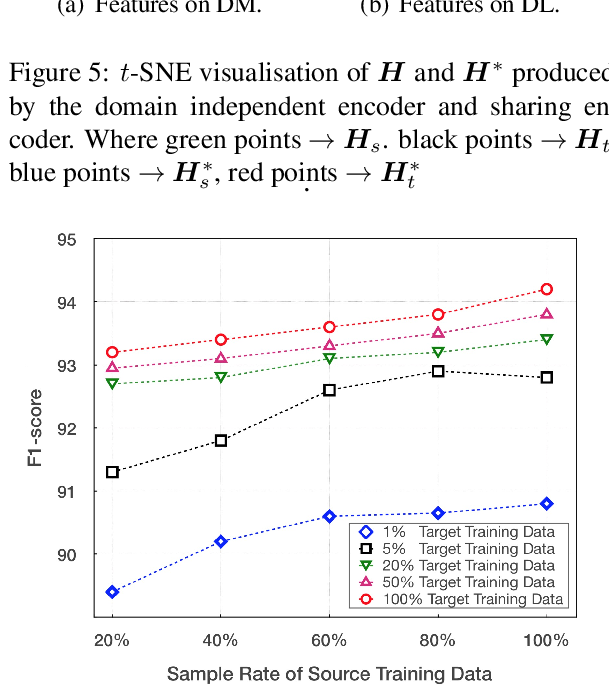
Multi-Domain Neural Machine Translation with Word-Level Adaptive Layer-wise Domain Mixing
Haoming Jiang, Chen Liang, Chong Wang, Tuo Zhao,
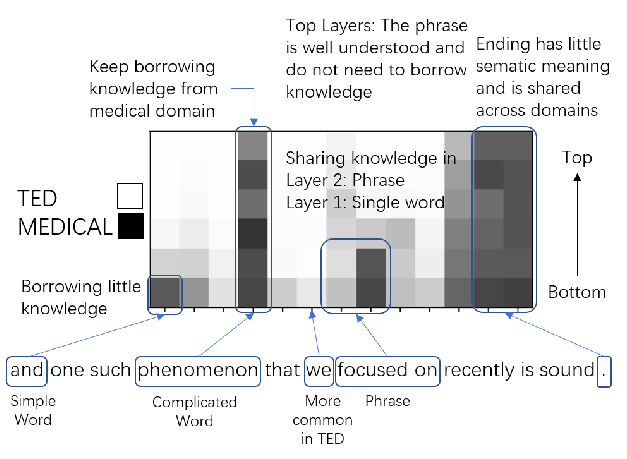
Dynamic Sampling Strategies for Multi-Task Reading Comprehension
Ananth Gottumukkala, Dheeru Dua, Sameer Singh, Matt Gardner,
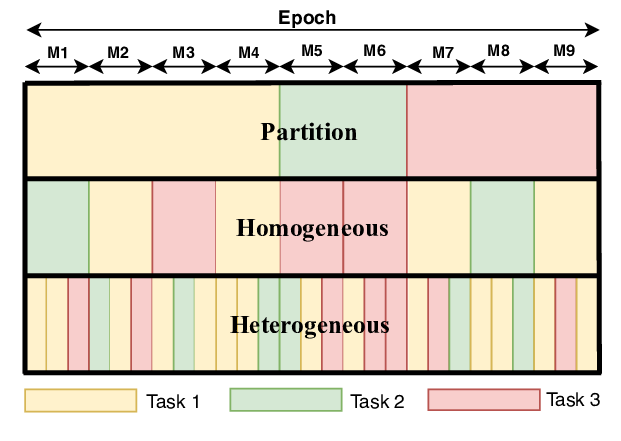