Unsupervised Domain Clusters in Pretrained Language Models
Roee Aharoni, Yoav Goldberg
Machine Translation Long Paper
Session 13B: Jul 8
(13:00-14:00 GMT)
Session 14B: Jul 8
(18:00-19:00 GMT)
Abstract:
The notion of ``in-domain data'' in NLP is often over-simplistic and vague, as textual data varies in many nuanced linguistic aspects such as topic, style or level of formality. In addition, domain labels are many times unavailable, making it challenging to build domain-specific systems. We show that massive pre-trained language models implicitly learn sentence representations that cluster by domains without supervision -- suggesting a simple data-driven definition of domains in textual data. We harness this property and propose domain data selection methods based on such models, which require only a small set of in-domain monolingual data. We evaluate our data selection methods for neural machine translation across five diverse domains, where they outperform an established approach as measured by both BLEU and precision and recall with respect to an oracle selection.
You can open the
pre-recorded video
in a separate window.
NOTE: The SlidesLive video may display a random order of the authors.
The correct author list is shown at the top of this webpage.
Similar Papers
Learning a Multi-Domain Curriculum for Neural Machine Translation
Wei Wang, Ye Tian, Jiquan Ngiam, Yinfei Yang, Isaac Caswell, Zarana Parekh,
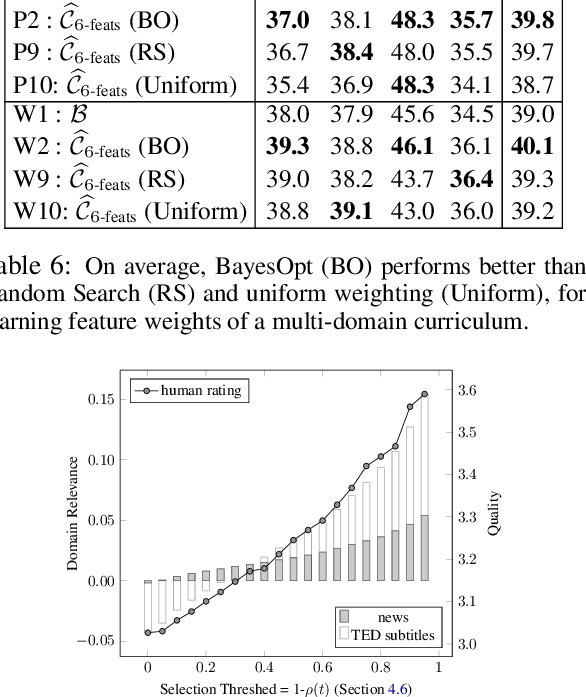
Coupling Distant Annotation and Adversarial Training for Cross-Domain Chinese Word Segmentation
Ning Ding, Dingkun Long, Guangwei Xu, Muhua Zhu, Pengjun Xie, Xiaobin Wang, Haitao Zheng,
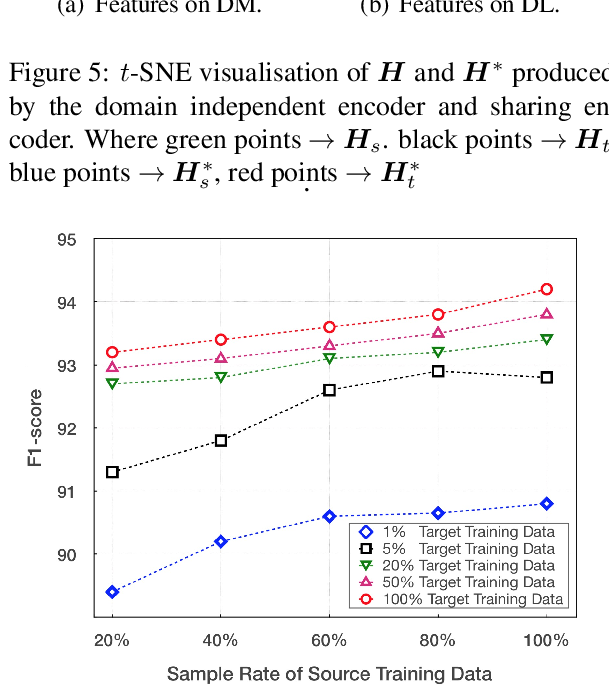
Multi-Domain Neural Machine Translation with Word-Level Adaptive Layer-wise Domain Mixing
Haoming Jiang, Chen Liang, Chong Wang, Tuo Zhao,
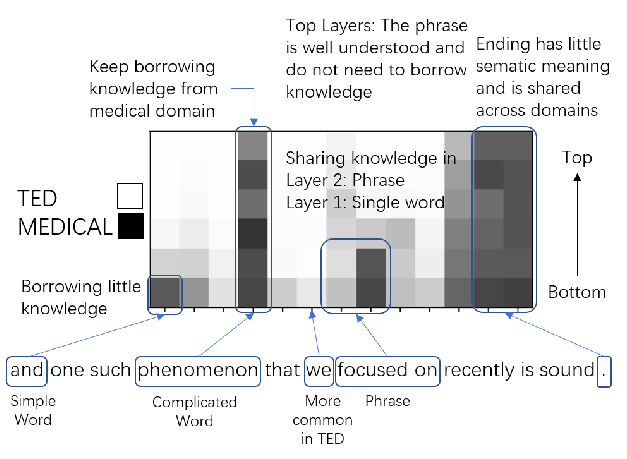
Don’t Stop Pretraining: Adapt Language Models to Domains and Tasks
Suchin Gururangan, Ana Marasović, Swabha Swayamdipta, Kyle Lo, Iz Beltagy, Doug Downey, Noah A. Smith,
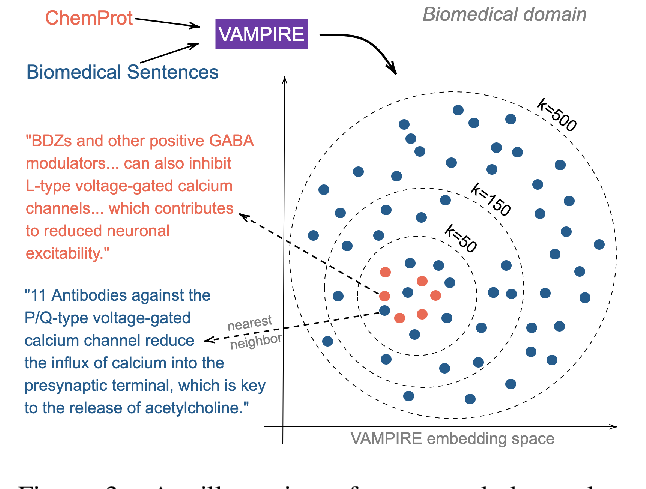